Measuring compute capacity: a critical step to capturing AI’s full economic potential
The development and use of trustworthy artificial intelligence (AI) require many things, including a skilled workforce, enabling public policies, legal frameworks, access to data, and sufficient computing power. Although there are widely accepted metrics to measure and benchmark many of these components, measuring national AI compute capacity remains underexplored. Many governments neither know what compute capacity their countries have, nor what they need, to realise their AI plans and fully benefit from the development and use of AI in their countries.
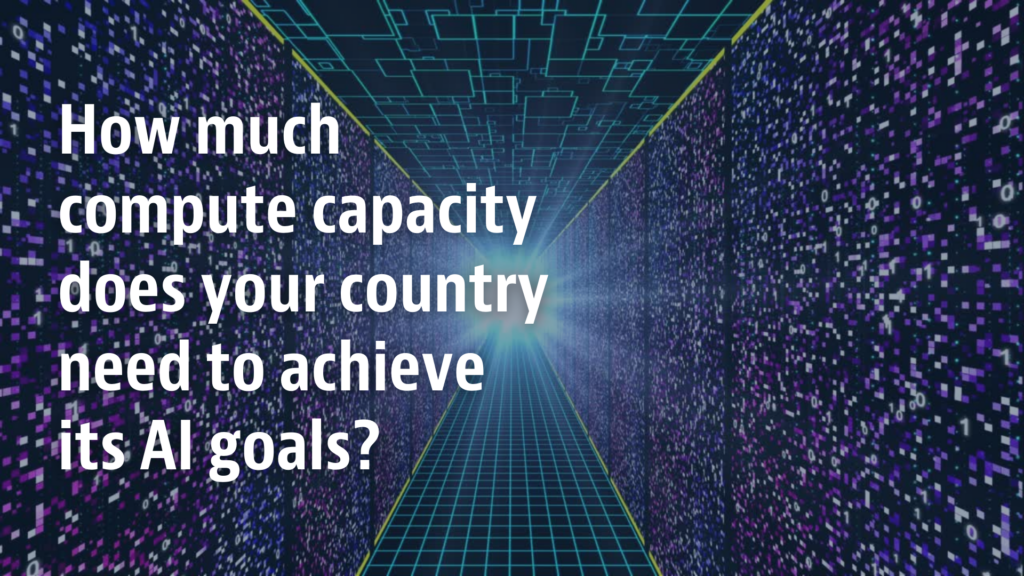
We increasingly co-exist with digital technologies in both physical and virtual worlds. This includes machines that can be pre-programmed and follow a precise set of rules, or ones that are fully autonomous and can operate without human intervention. Digital technology platforms can also be immersive like gaming, or life-impacting like medical diagnostics. Behind most of these innovations are complex mathematical models trained on large computers to emulate human-like cognitive function – this is artificial intelligence (AI).
Most countries have by now identified AI as a priority technology, and as a lever for economic growth, leading to national AI plans. For the most part, these plans are aspirational, reflecting how countries aim to capture the benefits of AI and to establish guardrails to protect society from the downside risks. Yet these national AI plans often share one limitation: they were developed without a full assessment of a country’s access to the compute capacity needed to create, train and use AI models. In brief, countries are planning for economic dividends from AI development and use without knowing whether they have sufficient compute capacity to attain these goals.
The relative lack of attention to this issue can be explained, at least in part, by the very technical nature of what AI compute is and how it works, which often requires a level of training and education beyond that of most policy makers. Another reason for the lack of focus on AI compute may be that many government officials mistakenly believe that AI compute is commoditized and easily sourced, as is the case with traditional information technology (IT) infrastructure (see US National AI R&D Strategic Plan: 2019 Update), given that commercial cloud providers are now widespread and offer robust services at scale.
While no widely used definition of AI compute capacity exists, it is comprised of a specialised stack of software and hardware (inclusive of processors, memory and networking) engineered to support AI-specific workloads or applications. It can include large data centres, supercomputers and cloud providers, as well as smaller data science laptops and workstations.
A lack of access to AI compute capacity can be problematic
Alongside data and algorithms, access to computing resources is a critical enabler for the advancement and diffusion of AI. As such, the ability to measure and understand a country’s access to AI compute capacity is a foundational element to building a strong AI ecosystem where countries and citizens can enjoy the full benefits of AI technologies.
Without a clear framework to help countries measure and benchmark their relative access to AI compute capacity, countries may be unable to make fully informed decisions on which investments are needed to fulfil their AI plans. This risks creating a divide between countries in their ability to compute the complex AI models that lead to competitive advantage in a global digital economy.
Take supercomputers, for instance; many suppliers operate in highly concentrated markets with most based outside of the country of use. This risks creating or widening interdependencies between countries in what could be seen as an “AI compute divide” for the most advanced and complex AI models. This point is made clear through a simple analysis of the 2021 TOP500 list of supercomputers. It shows that fewer than 31 countries have a system that today qualifies as a top supercomputer. The majority of such systems sit in the United States (US) and China, and the global south is nearly fully absent from the list.
Evidence also suggests that an AI compute divide could exist not only between countries but also within them. For example, only a small number of private firms and universities in the US can afford to procure and maintain a leadership-class AI supercomputer, which risks creating “haves and have nots” among US researchers (see VentureBeat article). Likewise, a recent report on large-scale computing published by the United Kingdom (UK) Government Office for Science noted that many smaller research centres and businesses have difficulty gaining access to large-scale computing platforms in the UK, which curtails the scope of their AI development.

Scale up, scale out and diversify
Countries need to consider how investments in domestic AI compute capacity can advance different types of policy objectives. For example, “scaling up” AI compute involves investment in a smaller number of larger AI systems intended for training the largest and most complex AI models. This policy goal of scaling up supports advances in domains such as natural language processing (NLP), precision medicine and autonomous vehicle development.
Alternatively, “scaling out” AI compute involves investment in a larger number of smaller AI systems to enable AI research and development (R&D) projects such as workforce training and student education. In this latter example, the goal is more about access than breakthroughs. We see this scaling out approach more commonly in Southeast Asia in countries such as Thailand and Indonesia where multiple smaller AI clusters are installed across universities, with government support, for the purpose of broadening access.
Governments should consider AI compute investments relative to their policy objectives – put differently, there are different ways to boost domestic AI compute capacity and the most resilient approach will depend on a country’s context and domestic needs. Such an approach could include investments in nationally owned or sponsored AI supercomputers and/or strategic partnerships with global and regional commercial cloud providers. But valuable AI compute can also be small, especially for students and junior researchers. Governments should keep in mind that even providing a data science laptop or workstation – which do not require the overhead of a data centre – can be a powerful path to AI innovation, broadening access and helping to close the compute divide.
The lack of consensus on how to measure and benchmark national AI compute capacity could lead governments to make sub-optimal policy decisions that may jeopardize the economic potential of their national AI strategies. The OECD’s mission is to provide evidence and data-driven insights and advice to help government decision-makers design better policies. The OECD.AI Network of Experts has created a dedicated Task Force on AI Compute to understand and measure national AI compute capacity for the purpose of closing this critical policy gap.
AI compute and the climate crisis
Future-proofing a national AI program requires more than domestic AI compute capacity. Countries must also be concerned with the environmental impacts of operating large-scale computers given the energy and water requirements. There is a clear policy imperative to measure national AI compute capacity and to use this information to promote not only more informed but also more sustainable public and private sector investments. For starters, the impact of AI on the environment can be mitigated by more efficient data science techniques that reduce the size of training data sets, and system architectures that optimize the entire data centre to promote energy efficiency.
Many agree that data centres must be optimized to decrease the environmental impact of AI development and use. This effort includes options such as integrating more energy-efficient server designs, network architectures and cooling methods as well as the use of renewable energy sources. Advances in data science that lead to smaller training data sets and fewer training runs are particularly important because knowledge diffuses rapidly relative to the time and effort required to update and modernise physical structures, such as data centres.
In a joint project with the Global Partnership on Artificial Intelligence (GPAI), the task force is assessing available indicators on the environmental impact of AI compute, including energy consumption, renewable energy procurement, water usage, lifecycle carbon emissions, data centre site selection, and the application of trustworthy AI principles through environmental impact transparency, data accessibility and supply chain accountability.
Understanding and addressing a growing AI compute divide
Our work on the task force to date highlights the need to understand and address the risks of a growing AI compute divide among, and within, countries. By filling the AI compute measurement gap, we aim to equip policy makers with the data they need to make more evidence-based decisions. As countries around the world grapple with global challenges – from COVID-19 to climate change – AI will remain an important vector of change with huge potential. This is why in 2019, governments around the world joined together to adopt the OECD AI Principles, in which they committed to “fostering the development of, and access to, a digital ecosystem for trustworthy AI”. Measuring the underlying compute capacity that makes AI possible is foundational to implementing the OECD AI Principles and ensuring that AI promotes inclusive and sustainable economic growth for all.
As the task force embarks on the next phase of this challenge to measure national AI compute capacity, broad collaboration is essential. To this end, we welcome participation and contributions from the private and public sectors, as well as technical and academic communities, to help the task force deliver on its mission.
For more information, please contact the OECD Secretariat at: AI@oecd.org.