Artificial intelligence and the future of science
Advances in science are essential to human flourishing and the natural world
The world faces many urgent challenges. Climate change, loss of topsoil, disease threats ranging from multi-drug resistant bacteria to the growing burden of neurodegenerative disease, are just some of them. Furthermore, the current pace of innovation is insufficient in some crucial fields. If we look at today’s leading energy generation technologies, most were developed over a century ago: the combustion turbine was invented in 1791, the fuel cell in 1842, the hydro-electric turbine in 1878 and photovoltaic cells in 1883. It’s not that these technologies have not evolved for the better. It’s just that there haven’t been any truly disruptive breakthroughs since (Webber et al., 2013).
There is always hope that progress in science and technology will address these and other challenges in ways that are cost-effective. For instance, synthetic biology could turn sugar-based microbes into substitutes for petroleum-based products to clean up production processes and slow global warming. Nanotechnology could create better hydrogen fuel cells for transport systems. There is even the prospect of construction materials that generate their own energy, and myriad other radical innovations stemming from materials science.
Is scientific productivity stagnating?
Claims of a slowdown in science stemming from numerous alleged causes are not new. However, such claims have been given new prominence by Bloom et al., (2020) and others. The metrics cited include the sharply rising number of researchers needed to maintain Moore’s Law and the decreasing number of those necessary to maintain improvements in crop yields and lower mortality due to cancer and heart disease. In addition, the real cost of developing a new drug doubles about every nine years and the share of breakthrough patents may be falling.
The evidence is disputed. But if it proves to be correct, any slowdown could lengthen timeframes for essential scientific progress. And governments under acute budgetary pressures might have to spend more just to support existing rates of growth in useful science.
Artificial intelligence is helping scientific progress
Spurred by advances in machine learning and fed by vast realms of scientific data, AI is being adopted across most stages and fields of science and in revolutionary ways. AI is helping to choose, design and plan experiments while improving measurement and observation: AI can now convert low-resolution images of things like mitochondria in cells into high-resolution, low-noise images. AI can also discover meaningful relationships in data sets that are so large they sometimes exceed the data created by the entire Internet. AI can generate hypotheses and learn scientific rules in areas like chemistry to predict how to make medicines. It can identify the most suitable patients for clinical trials of drugs and train laboratory robots to perform new tasks. It can predict the replicability of research and even suggest experts review research proposals.
There is much that AI cannot do, from explaining its own findings to truly understanding natural language, but scientists like those at the Alan Turing Institute in London hope that AI will increase the pace of discovery. They recently created the Turing AI Scientist Grand Challenge, with the goal of developing AI systems that can make Nobel quality scientific discoveries highly autonomously at a level comparable to the best human scientists, if not better, by 2050.
But AI isn’t a panacea yet, and machine intelligence needs further development
Nevertheless, AI might not sit on the critical path to raising the productivity of science. It may be able to raise productivity in individual processes, but the effects could be small in a context of system-wide constraints such as inefficient processes of peer review or misaligned incentives for careers in science, and so on. Scientists also need new AI tools to do things like interpreting the complex relationships that AI applications reveal, to truly understand natural language and scientific text, and create AI applications that from the start embody knowledge of existing scientific theory.
Good policies will increase the positive impacts of AI on science
Policies that affect things such as data access can help to quicken things like drug discovery (New, 2019). Public R&D could help advance a number of fields of relevant research, for instance, to develop less computationally intensive forms of AI, which could allow students and other researchers to engage in cutting-edge AI research that would otherwise be prohibitively expensive.
Governments and other entities need to act to improve skills, education and access to high-performance computing for research projects. This occurred in various public-private partnerships during the Covid-19 pandemic. Norms in scientific institutions like the format of the typical scientific paper may also need to adapt to new forms of discovery. Along the same lines, institutions need to publish more negative experimental results.
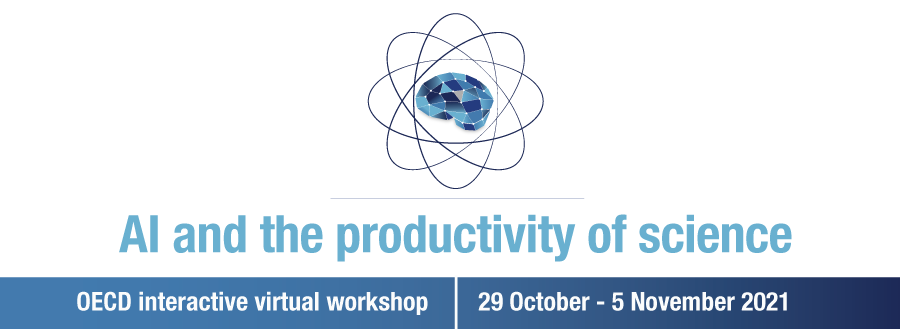
OECD workshop on AI and the productivity of science (October 29th – November 5th)
Our OECD workshop that will contribute to a publication, will bring together scientists, AI researchers, policy analysts and scholars who specialize in the economics of science. From multiple vantage points, these experts will assess the evidence on progress in science and examine how AI is contributing to all stages of the scientific processes. They will consider AI’s current limitations in science, along with the impact of AI on science in the developing world, and the policy implications for current and future developments. The workshop will be live-streamed and is open to the public. An OECD publication on these topics will be launched in the second quarter of 2022.
save the date and register for the forthcoming OECD workshop on AI and the productivity of science, which will be held virtually on 29 October – 5 November 2021, in collaboration with FONDATION IPSEN.