Gender bias in AI: what the United States, Canada, and Western European nations can learn from other countries
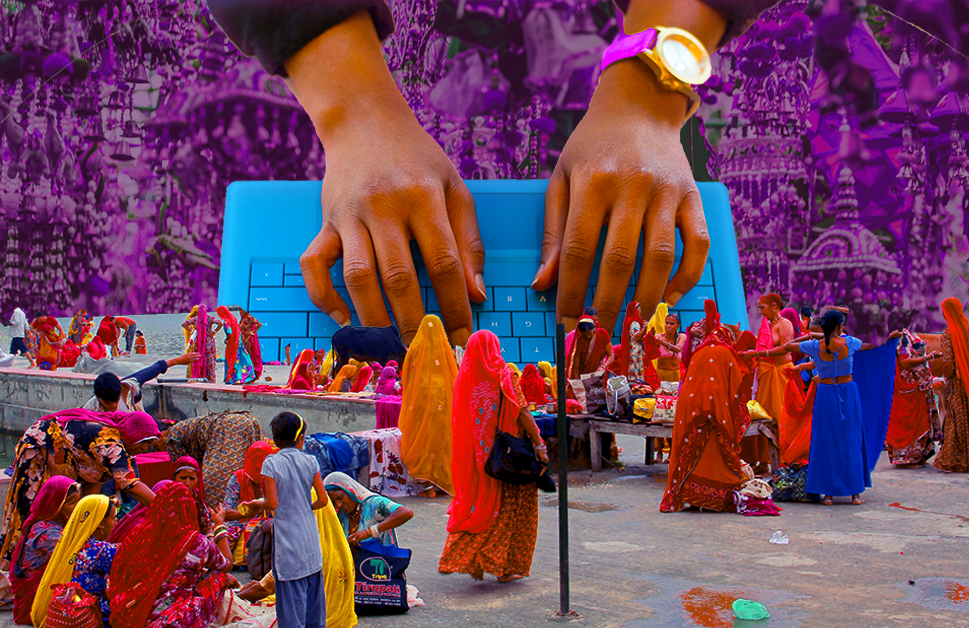
Imagine a society in which all new services are conceptualized, developed, and maintained by one group of people with very similar traits, educational backgrounds, and worldviews. The services they design would likely serve people like themselves better than others. One could argue that even with the best of intentions, their services would be tailored to the context they know best: their own. Like it or not, this homogenous group of people would exhibit bias in at least some of its decisions.
Based on emerging technology and current trends, this society would rapidly move to integrate artificial intelligence (AI) into practically every decision-making process. In time, intelligent machines would take over the homogenous group’s role. The machines would base decisions on logic and unbiased data alone, seemingly free from bias. But what would happen if the group designing the machines were as homogenous as the group that designed the new services? Would their biases impact the AI systems they create?
Unfortunately, reality currently reflects that this group is homogenous, at least in one respect—AI research lacks gender diversity. The World Economic Forum found that in 2018, only 22% of AI professionals globally were women. When calculating AI research publication data for the OECD, Elsevier detected gender disparity among authors who published in the AI field from 2010 to 2020. And in 2020, only 14% of authors around the world who wrote AI peer-reviewed articles were women. This last number closely resembles the gender balance rates found in the larger computer science field of research. With such a gender imbalance in the AI workforce, it’s unsurprising that people have found gender biases in multiple AI systems, such as Amazon hiring AI and Google Translate.
Biases in AI systems are usually attributed to the biased data used to train algorithms. However, this bias is linked to an even deeper problem: the lack of diversity in the teams that develop AI. Homogeneous teams are less equipped to de-bias data sets and detect discriminatory outcomes than more diverse ones. The solution to such issues starts with recognizing the lack of diversity in AI and looking for ways to create more diverse teams. Examining the data on gender diversity and associated historical and cultural factors can help this process.
The surprising gender (dis)parities in AI on national levels
When looking at the share of women authors who contribute to AI research publications around the world, some countries seem to have less of a gender gap than others. This is especially true for India, Bulgaria, Malaysia, Indonesia, Romania, Serbia, and Ukraine. Indeed, Elsevier’s work for the OECD revealed that the share of women authors who contribute to AI articles in each of these countries has hovered at around 30% for the past few years. While this may not be perfect parity, it is almost double the global average. Furthermore, in 2020, India and Bulgaria were found to have higher shares of women authors for AI publications than for overall publications nationally.
This observation is striking given that some of these countries harbour significant gender inequalities as a whole. As reported in the Global Gender Gap Report 2021, some of these countries did not rank well in the Global Gender Gap Index: Indonesia was 101st, Malaysia was 112th, and India was 140th out of 156 countries.
However, the same report also revealed a virtual gender parity among Indian higher education graduates with 6% of women and 7% of men having pursued programmes from the larger field of information and communication technologies (ICT). This is much more balanced than the United States, Canada, and Western European nations in general. For instance, the Global Gender Gap Index ranked the United States as 30th, but the same report showed that the US was much more imbalanced when it came to higher education graduates, with 1.5% of women and 7% of men chosing to study ICT. From the aforementioned countries with a smaller gender gap, only Ukraine (ranked 74th on the Index), had a worse ICT higher education graduates balance rate than the United States with 1.5% of women to 8.2% of men higher education graduates studying ICT.

Social norms, workforce needs, and education policy
In the countries exhibiting less disparity, this relative gender balance is linked to the Computer Science (CS) field in general being perceived positively by all genders. In India and Malaysia, jobs related to CS are broadly perceived as appropriate and desirable for women, as they are high-paying jobs in a safe environment. The sector also has very high growth and perceived job safety, something that research has shown that both Indian and Malaysian women see as a way to gain increased social independence. Furthermore, CS-related jobs and the people that hold them are held in high esteem in these countries.
In the former Soviet Bloc countries of Bulgaria, Romania, Serbia, and Ukraine, the better gender balance in AI may be linked to their socialist history. During the Soviet era, women were a vital part of the workforce and often supported in entering professions previously dominated by men, lessening gender role biases for future generations. Other ex-Soviet Bloc countries such as the Czech Republic and Hungary closely follow the global average when it comes to the gender balance rate in AI publications, indicating that there are likely to be other contributing factors.
In Bulgaria, it is evident that other factors are also at play. Bulgaria’s education system has a strong focus on STEM and CS, both in and out of school. This focus and valorisation of STEM fields in the school curriculum are believed to play a vital role in increasing the level of self-efficacy and confidence that girls have in these areas, providing opportunities to overcome initial self-doubt.
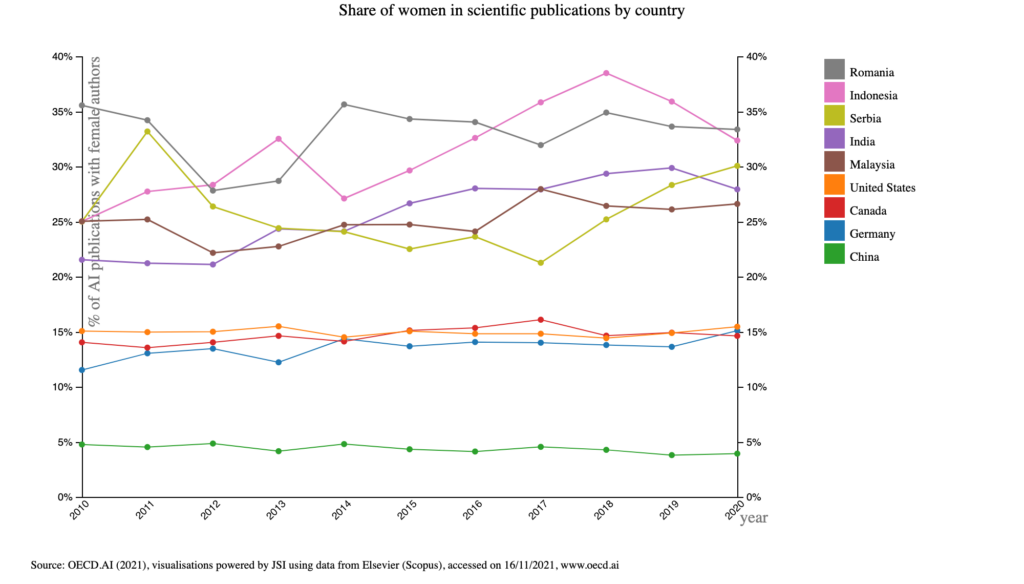
Source of data: Elsevier (Scopus)
Please cite as: OECD.AI (2021), visualisations powered by JSI using data from Elsevier (Scopus), accessed on 16/11/2021, www.oecd.ai
The roots of Western gender disparity in computer science
Compared to these countries with a small gender gap in AI, Western countries exhibit greater gender disparity. In 2020, women made up approximately 14% of authors on AI papers in the United States, Canada, the United Kingdom, and Germany. In contrast, the same report shows that in the United States, a greater share of women was engaged in science generally in 2020, making up 30% of the authors on science-related papers published in that year. In some research fields, such as nursing and psychology, most authors were women.
The gender disparity in AI and CS didn’t always exist in these countries. In fact, the share of women among those awarded with a CS bachelor’s degree declined from 37% in 1985 to only 20% in 2018. The imbalance between women and men within CS has grown, a subject of research for about 30 years. It was just over 30 years ago when computers started appearing in American homes; prior to this, women dominated the field. In fact, the marketing push behind household computer adoption mostly targeted men and is thought to have played a role in decreasing the share of women pursuing studies in CS, making the field evolve from being women-dominated to its current state. There are more reasons for the decreased share of women in CS that have been unearthed through research.
Negative stereotypes and men-dominated environments
Societal influences such as stereotyping along gender lines from a very young age play a role, as they falsely persuade girls into believing they are less capable than boys at CS. These societal cues have been found to affect children as young as six years old, with girls being less likely than boys that age to believe that members of their gender are “really, really smart.” These internalized stereotypes drive girls away from activities that society has designated for boys. These stereotypes are also linked to lower CS self-efficacy in women, another reason women shy away from majoring in CS.
The second set of factors comes from the masculine nature of current CS environments. Being one of the only women in a space can feel isolating and lower the sense of belonging, especially if colleagues share different interests and engage in traditionally masculine activities. This association between men and CS is largely absent from cultures with more balanced gender rates.
Current tech culture can also feel unwelcoming for people wanting to start a family by perpetuating unreasonable expectations. For example, some companies pressure new mothers to remain available while they are on maternity leave. These environments are also more likely to exhibit gender discrimination through sexual harassment and discriminatory policies, among other forms. Even companies who proudly claim they value diversity, equity, and inclusion, such as Google and Microsoft, are not immune to such behaviours. This climate contributes to the fact that only 38% of women holding a degree in computer science in the United States actually work in the field, compared to 53% of men.
A lack of role models
A lowered sense of belonging also stems from the lack of women role models in the field. The overrepresentation of men is apparent simply by looking at the founders and current CEOs of the GAFAM companies, all of whom are men. This is despite pioneering women in CS such as Ada Lovelace and Grace Hopper. However, the problem resides more in the lack of role models who would have direct contact with girls and women. Statistically, the lack of women working in CS leaves most girls without individuals they can identify with and emulate throughout their education and career, which is known to have important consequences, especially when it comes to retaining women in the field.
Compounding these issues is the popular depiction of programmers in the United States and other Western cultures as men who are “nerds” obsessed with computers, to the point of neglecting their personal lives. This is a damaging stereotype that is largely absent from other cultures and sometimes the only reference some people have to the CS field.
Addressing the AI gender bias
These observations reveal that greater inclusion of women in CS and AI research must go hand-in-hand with societal changes. In this regard, Western countries should take examples from India and Bulgaria. The detrimental stereotype that only men belong in AI and CS must change. Further, the overly masculine climate typical of the modern tech world must be overhauled, making diversity, equity, and inclusion core principles of any workplace and school. This would go a long way toward ensuring that women who undertake CS studies actually reach the CS workplace and stay there. Luckily, many United States institutions are making efforts to ensure that early opportunities for all girls to develop CS skills exist, and the push to make CS a core part of the school curriculum should continue.
Doing so will nurture diverse and inclusive AI teams that are better equipped to detect biases through greater awareness of existing issues thanks to more diverse perspectives. What’s more, diverse teams are more innovative and may produce higher-quality science. That means that skipping over diverse profiles because of gender or sociodemographic differences reduces competitiveness. In a highly competitive field such as AI, this could have important positive economic consequences for countries that succeed and negative ones for those that fail to foster diversity.
Of course, gender diversity is not the only kind of diversity that matters in AI research teams. Diversity should also be nurtured across race, ability, socioeconomic status, age, nationality, and other social identifiers. But increasing team diversity alone will not miraculously remove all bias issues from AI. Other safeguards should also be put in place such as systematically testing AI models for biases before use and developing more transparent “explainable AI” algorithms. However, it is still vital for the field and society at large to become more supportive of women who wish to pursue a career in CS and for AI to be impartial.