Testing frameworks for the classification of AI systems
What CSET and OECD learned by comparing how frameworks can guide the human classification of AI systems.
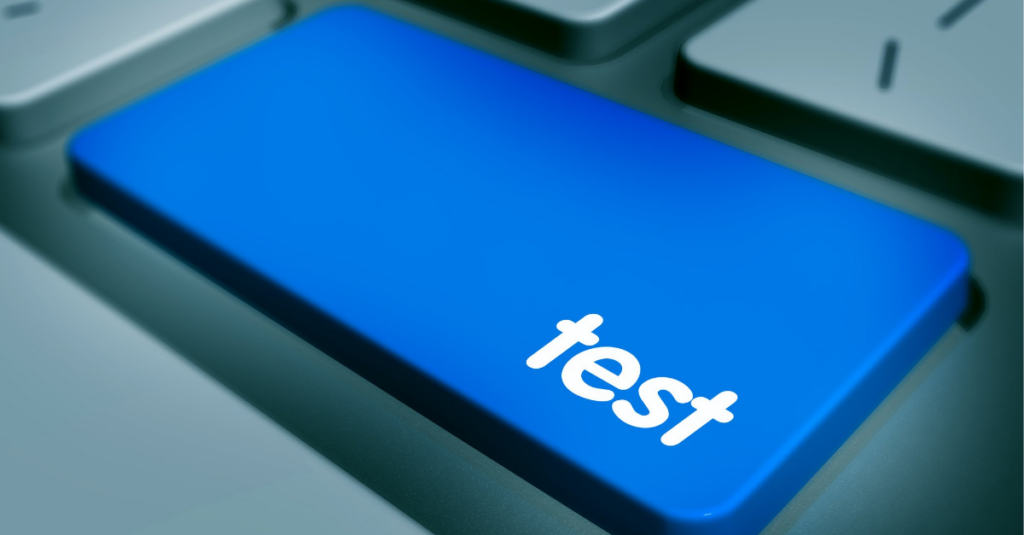
Artificial intelligence (AI) governance is a pressing policy issue. Policymakers and the public are concerned about the rapid adoption and deployment of AI systems. To address these concerns, governments are taking a range of actions, from formulating AI ethics principles to compiling AI inventories and mandating AI risk assessments. But efforts to ensure AI systems are safely developed and deployed require a standardized approach to classifying the varied types of AI systems.
This need motivated OECD and its partners to explore the potential of frameworks—structured tools to distil, define, and organize complex concepts—to enable human classification of AI systems. While discussions were underway at the OECD about what such a framework should look like, Georgetown University’s Center for Security and Emerging Technology (CSET) began to brainstorm how we could test the various frameworks being discussed. CSET’s premise was that a framework has to be both understandable and able to guide users to consistent, accurate classifications. Otherwise, it is of little use to policymakers or the public.
CSET recently published a report outlining findings from testing various frameworks and launched an interactive website where you can explore the frameworks, review framework-specific classification performance, and even try classifying a few systems yourself. Some highlights from that research, and CSET’s ongoing work with OECD on this topic, are summarized here.
Why classify AI systems?
Does the AI system act autonomously or does it provide a recommendation to a human who then acts? What sector is the AI system deployed in? How does the AI system collect data? These are questions that are relevant for AI governance and point to observable attributes of an AI system. The answers to these questions can tell us what type of AI system we are encountering. In other words, if we can observe an AI system’s autonomy and classify it according to a standard scale of autonomy levels we can better understand and communicate something about the kind of system it is and how it works.
Combining the defined level of autonomy with other observable system attributes such as impact, end-user profiles and deployment sector provides an AI system classification. When multiple systems are classified using the same attributes or dimensions, it gives us standard terms for describing AI systems and enables comparisons across AI systems and territories.
Specifically, building an AI system classification framework includes two steps:
- Identify policy-relevant characteristics of AI systems (e.g., autonomy, impact, data collection method) to be the framework dimensions;
- Define a scale for each dimension (e.g., low, medium, high) to characterize AI systems.
Using such a framework, system developers and governing bodies can classify systems in a uniform way and use those classifications as a first step toward assessing risk, monitoring bias, and managing system inventories.
Classifying the gaming AI system AlphaGo Zero
AlphaGo Zero is a system that plays the board game Go in a virtual environment, often beating professional human players. AlphaGo Zero uses both human-based inputs, including the rules of Go, and machine-based inputs, primarily data learned through repeated play against itself, to play the game. It abstracts data into a model of moves through reinforcement learning and then uses the model to make its next move based on the current state of play. The system is deployed in a narrow setting for research and recreational purposes and has no immediate impact beyond the outcome of the game.
Using an AI system classification framework described below (Framework A) and only this basic information about AlphaGo Zero, we can classify it as a “high autonomy” and “low impact” AI system. We can now clearly communicate two policy-relevant characteristics of AlphaGo Zero—it can take an action (make a move in the game) autonomously, but that action has minimal impact on individuals or on society. We can also more easily compare AlphaGo Zero to other systems classified using the same framework. For example, it is similar, along these dimensions, to other systems classified as “high autonomy” and “low impact,” such as a virtual website navigation assistant. But it is very different from a missile defence AI system, classified as “high autonomy” and “high impact.”
In addition to clear, consistent, and comparable system information, we can now use AlphaGo Zero’s classification to inform an evaluation of its risk profile and identify management and regulatory needs, or the absence of such needs. Specifically, one could now flag AlphaGo Zero as a system that does not require a high degree of regulation. Applying the same framework to a wider sample of AI systems, or even all of them, would make it possible to place AI systems on an autonomy scale (low, medium, high) and then target AI systems that would need regulation.
Designing a test for the frameworks
CSET designed a survey experiment to test the usability of various classification frameworks. These included a version of the OECD’s Framework for the Classification of AI Systems as well as several versions of a framework CSET developed in discussions with the U.S. Department of Homeland Security Office of Strategy, Policy, and Plans. These collaborative efforts prove that the need for AI system classification is not specific to a single agency, or even country, and the proposed solution of a classification framework is based on input from a wide range of stakeholders.
Each tested framework used the OECD definition of an AI system as:
A machine-based system that is capable of influencing the environment by making recommendations, predictions or decisions for a given set of objectives, which uses machine and human-based inputs to perceive the environment, abstract perceptions into models, and formulate options for outcomes.
The frameworks varied in the dimensions and labels they included. Frameworks A, B, and C were variations on a framework with two core dimensions: autonomy and impact. During the experiment, each framework included a rubric that summarized the dimensions, with the exception of Framework A, which was provided to some respondents without a rubric included.
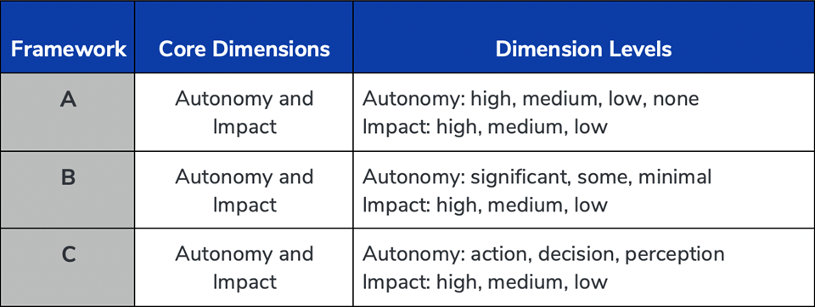
Framework D, meanwhile, had four core dimensions—context, input, AI model, and output—each with one or more sub-categories, for a total of nine dimensions. This framework is an earlier version of the OECD’s framework for the classification of AI systems.
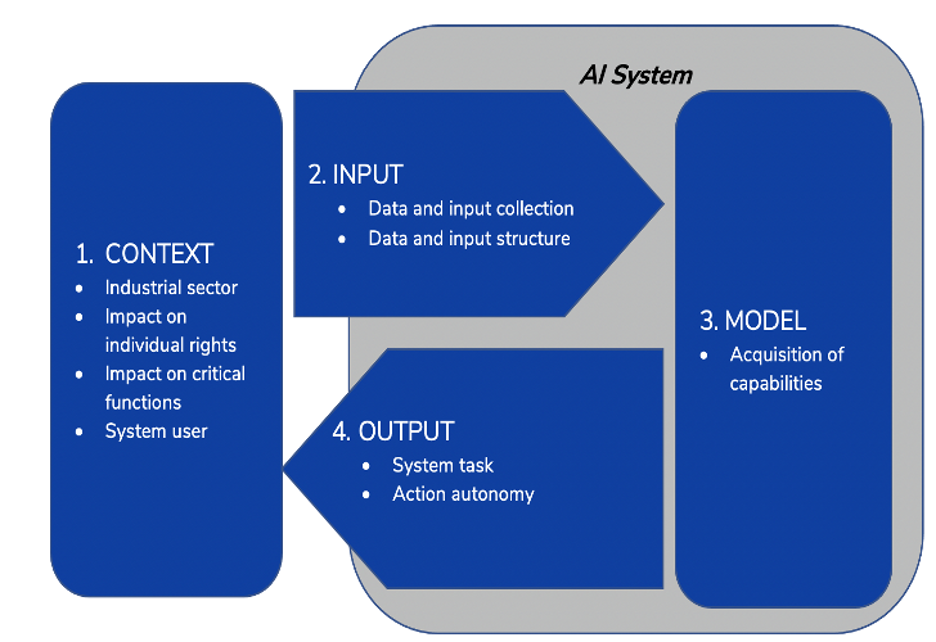
The experiment randomly assigned survey respondents to one of these frameworks and then asked them to read the framework and use it to classify a small number of real-world AI systems. While the example systems and other survey questions remained constant, which framework the respondent was asked to use varied. The survey was conducted over two rounds from September to December 2020 and resulted in more than 360 respondents completing over 1,800 unique AI system classifications using the frameworks.
What we learned
We compared respondents’ assignment of consistent and accurate system dimension (e.g., AlphaGo Zero’s autonomy level) classifications across frameworks. By classification consistency, we mean when 65 per cent or more respondents provided the same system dimension classification and matched an expert classification. The goal was to determine which framework, if any, was most effective.
Certain frameworks produced more consistent and accurate classifications. The higher-performing frameworks (C and D) more than doubled the percentage of consistent and accurate classifications, compared to the lowest-performing framework (A without a rubric).
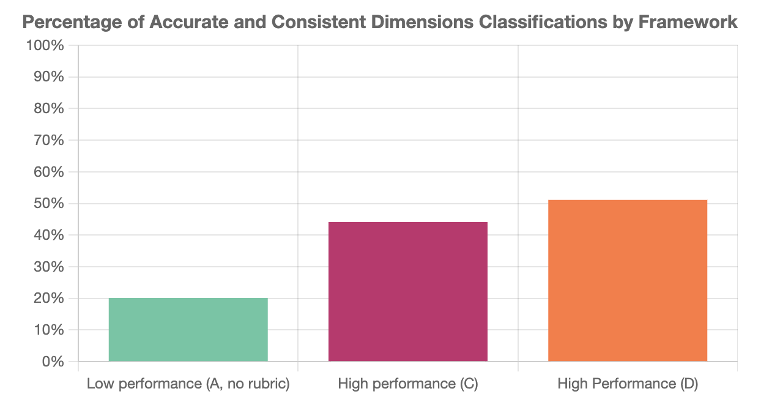
Including a summary rubric of framework dimensions improved classification. We found a significant decrease in consistent and accurate classifications when users were not provided with a rubric, compared to instances when a rubric was provided.
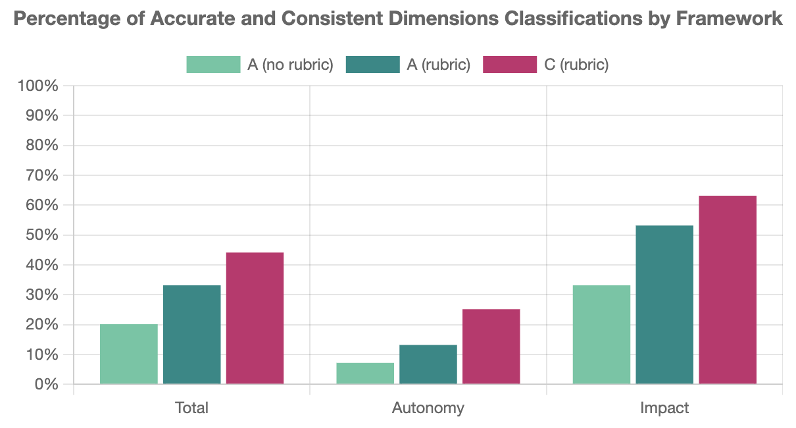
Users were better at classifying an AI system’s impact level than its autonomy level. Across all frameworks, users consistently assigned the accurate system impact but struggled to consistently assign the accurate level of system autonomy. Specific to Framework D, users were better at classifying system deployment context than technical system characteristics.
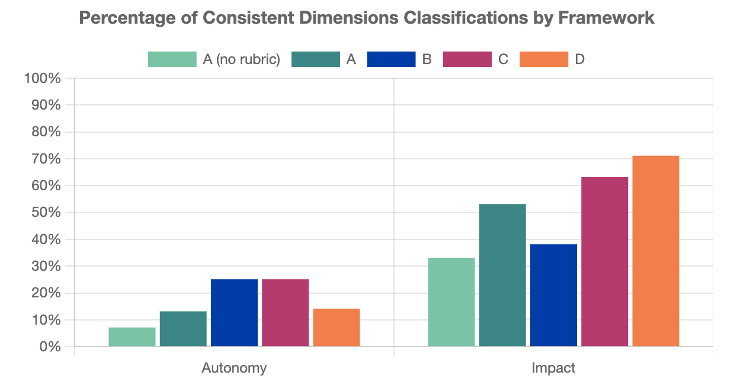
We also found some evidence that users were better at classifying an AI system’s autonomy level when the framework provided more descriptive levels, such as “action,” “decision,” and “perception” as opposed to “high,” “medium,” and “low.”
A broader finding from the survey, and from the overall process of designing and conducting this study, was that classification depends on sufficient accessible information about the system. We found classifications were more varied when system descriptions did not include a specific use case, thus leaving critical context about the system’s operation open to interpretation. Additionally, classifications were less accurate and consistent for more technical (i.e., data and model) dimensions because classifying these characteristics requires more information than is typically available about an AI system due to its proprietary or technically complex nature.
A usable AI system classification framework is the first step toward risk assessment, efficient AI management by organizations, and increased public awareness of AI systems—but a framework is only part of the task. Ensuring users have access to relevant system information, including technical system characteristics, is key to the process of classifying AI systems.
What’s next?
We found that with minimal system information, individuals with limited technical knowledge can, with moderate success, classify policy-relevant characteristics of AI systems. But there is still much work to be done.
To that end, CSET continues to support the testing and implementation of these frameworks, or improved versions of them, in actual policy contexts. This includes continued participation in discussions with the OECD.AI Network of Experts’ working group on AI Classification. That working group is currently integrating feedback from public consultation, and the updated framework is slated to be launched in early 2022. This also includes exploring and evaluating complementary frameworks for assessing AI risk, bias, or trustworthiness. Given the importance of classification for AI governance, CSET and OECD are eager to continue developing an agreed-upon, validated, and usable classification framework for AI systems.
To learn more about this research, see CSET’s Classifying AI Systems data brief and interactive site.
CSET did not receive any funding for this research from the U.S. Department of Homeland Security or any other government entity. The views and conclusions contained in this document are those of the author and should not be interpreted as necessarily representing the official policies, either expressed or implied, of DHS and do not constitute a DHS endorsement of the rubric tested or evaluated.