How much water does AI consume? The public deserves to know
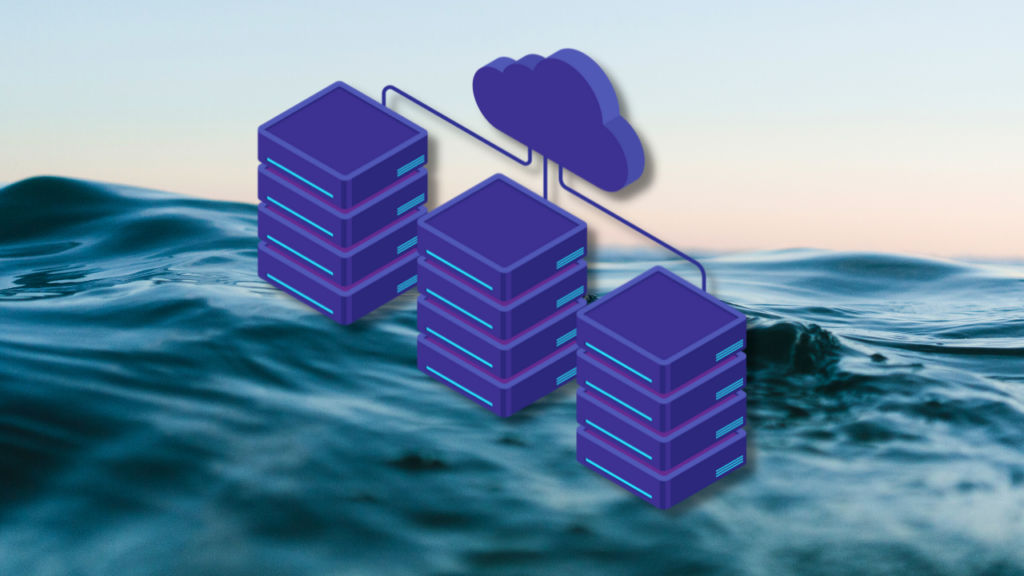
Everyone is talking about artificial intelligence. It’s not simply a buzzword; it has already become the backbone for scientific breakthroughs, accelerated business growth, and approaches to global challenges such as climate change. AI models are essentially complicated mathematical functions with many parameters. AI’s ever-growing capabilities rely on huge volumes of data and computationally intensive calculations to extract useful patterns.

Servers are hungry. And thirsty
Large AI models like GPT-3, with many billions of parameters, are often trained and deployed on large clusters of servers with multiple graphic processing units (GPUs). These servers are so power-hungry they can consume a few kilowatts each, the equivalent of the average power consumption of an entire house. So, despite the recent algorithmic and hardware efficiency improvements, AI model training and inference still result in enormous energy consumption.
Air pollution and carbon emissions are well-known environmental costs of AI. But, a much lesser-known fact is that AI models are also water guzzlers. They consume fresh water in two ways: onsite server cooling (scope 1) and offsite electricity generation (scope 2).
- Scope-1 onsite water consumption: AI servers’ massive energy consumption generates much heat. To dissipate the heat into the outside environment and avoid server overheating, data centres commonly use cooling towers and/or outside air, which need a staggering amount of clean, fresh water. Cooling towers rely on water evaporation to produce cold water, and outside air needs water for evaporation assistance when it’s too hot and for humidity control when it’s too dry. Because of the high power densities of AI servers, on-chip liquid cooling may be employed: closed-loop circulating liquid directly moves the heat from the servers to the data centre facility (e.g., the facility’s cooling water loop). Then, the heat moved to the facility will be rejected by cooling towers or outside air, which consumes water.
- Scope-2 offsite water consumption: Generating electricity also consumes a lot of water through cooling at thermal power and nuclear plants and expedited water evaporation caused by hydropower plants. Thus, AI is responsible for scope-2 offsite water consumption.
The scope-1 and scope-2 water consumption are sometimes collectively called operational water consumption. There is also scope-3 embodied water consumption for AI supply chains. For example, to produce a microchip takes approximately 2,200 gallons of Ultra-Pure Water (UPW). That aside, training a large language model like GPT-3 can consume millions of litres of fresh water, and running GPT-3 inference for 10-50 queries consumes 500 millilitres of water, depending on when and where the model is hosted. GPT-4, the model currently used by ChatGPT, reportedly has a much larger size and hence likely consumes more water than GPT-3.
Water consumption vs. water withdrawal
There are two related but different types of water usage: water withdrawal, a.k.a. water abstraction, and water consumption, both of which are important for holistically understanding the impacts on water stress and availability. Despite appearances, water consumption is a technical term and differs dramatically from water withdrawal.
Water withdrawal refers to freshwater taken from the ground or surface water sources, either temporarily or permanently, and then used for agricultural, industrial or municipal uses. On the other hand, water consumption is defined as “water withdrawal minus water discharge”, and means the amount of water “evaporated, transpired, incorporated into products or crops, or otherwise removed from the immediate water environment”.
While the evaporated water still stays within our planet just like any other matter, it may go somewhere else and further contribute to the already uneven distribution of global water resources.
By default, water footprint refers to water consumption. But water withdrawal is also a crucial metric and indicates the level of competition and dependence on water resources among different sectors. Indeed, electricity generation is among the top sectors for water withdrawal in many countries. The global AI demand may even require 4.2 – 6.6 billion cubic meters of water withdrawal in 2027, which is more than the total annual water withdrawal of 4 – 6 Denmark or half of the United Kingdom. If the U.S. hosts half of the global AI workloads, the operation of AI may take up about 0.5 – 0.7% of its total annual water withdrawal. Simultaneously, the total scope-1 and scope-2 water consumption of global AI could exceed 0.38 – 0.60 billion cubic meters, i.e., roughly evaporating the annual water withdrawal of half of Denmark or 2.5 – 3.5 Liberia.
Energy efficiencies are outpaced by growth in AI computing
While there have been efficiency gains elsewhere, the exponentially growing demand for AI has increased the water footprint.
For example, driven partly by the growth in AI, Google’s scope-1 onsite water consumption in 2022 increased by 20% compared to 2021, and Microsoft saw a 34% increase over the same period. Most big tech water consumption for server cooling comes from potable sources. Here, the consumed water is actually evaporated and “lost” into the atmosphere.
AI water consumption creates social tensions
We haven’t come to the point yet where AI has tangibly taken away our most essential natural water resources. But AI’s increasing water usage (both withdrawal and consumption) is definitely concerning. Water scarcity has become one of the most pressing global challenges as we deal with the rapidly growing population, depleting water resources, and ageing water infrastructures, especially in drought-prone regions. The concern is not only about the absolute amount of AI models’ water usage, but also about how AI model developers respond to the shared global challenge of water shortage.
As droughts are one of the first catastrophic consequences of climate change, everyone should take their share of the responsibility to address the water challenge. We already see heated tensions over water usage between AI data centres and local communities. If AI models keep on guzzling water, these tensions will become more frequent and could lead to social turbulence.
There needs to be more transparency
If we want AI to grow healthily and responsibly, we have to reduce its water usage, especially its water consumption. Water has been undervalued for so long that its true value might have been forgotten. Today, AI’s water footprint has received much less attention than it deserves.
Looking at the details of an AI model card, a fact sheet that includes information about how an AI model is trained and how it should be used usually includes scope-2 offsite carbon footprint associated with the energy usage for model training.
Unfortunately, the model card contains almost no information about water. Even the scope-1 onsite water consumption for AI model training is not reported, not to mention the scope-2 offsite water consumption from electricity generation. This is like excluding the calorie information from the nutrition facts label of a food product. This lack of transparency impedes innovations to enable water sustainability and to build genuinely sustainable AI.
Water is a vital and finite resource that should be shared equitably. As the AI industry continues booming, the public definitely deserves to know its increasing appetite for water. Big techs have started replenishing watersheds to offset their cooling water consumption and achieve “water positive by 2030” for their data centres.
These water conservation efforts are certainly commendable, but this doesn’t mean that AI models, especially public AI models for critical applications, can continue guzzling water under the radar. Just as they report the carbon footprint, AI model developers should be more transparent about their AI models’ water footprint as part of the environmental footprint disclosure in the model card.
It is quite simple to calculate AI’s water footprint:
WaterFootprint = ServerEnergy*WUEOnsite+ServerEnergy*PUE*WUEOffsite
AI’s water footprint varies significantly depending on where it is trained and hosted. For example, AI consumes 1.8 – 12 litres of water for each kWh of energy usage across Microsoft’s global data centres, with Ireland and the state of Washington being the most and least water-efficient locations, respectively. The scope-1 onsite water consumption may be higher or lower than scope-2 offsite water consumption, depending on the data centre cooling technique and how electricity is generated in the local grid. For example, if a cooling tower is used for data centre cooling and the local grid primarily uses solar and wind energy, the scope-1 onsite water consumption can dominate.
Built-in power sensors or metres can easily measure server energy for AI model training and inference The onsite WUE measures the water efficiency for cooling systems. Power Usage Effectiveness, PUE, measures the non-IT energy overheads such as cooling energy and power distribution losses. Offsite WUE, also called the electricity water intensity factor, measures water efficiency for electricity generation.
The disclosure of AI’s water footprint can be easily streamlined and standardised for better transparency. Accurate information about AI’s water footprint allows holistic benchmarking of AI’s environmental impact and complements existing water sustainability efforts.
AI is one of the most flexible workloads in data centres: models can be trained almost anywhere in the world, and inference can be performed with different model sizes to yield different tradeoffs between model accuracy and resource usage.
If we don’t measure AI’s water footprint, we can’t optimise it. Monitoring AI’s water footprint can open up many new ways to reduce it. For example, it can inform AI developers and help them exploit the spatial and temporal flexibilities of AI, deciding where and when to train and deploy AI models. Monitoring can enable flexible performance tradeoffs between an AI’s model accuracy and water consumption: if the AI model is deployed in a water-stressed area, it probably makes more sense to use a compact AI model with a smaller water footprint.
Importantly, factoring locational water efficiency differences into AI’s scheduling decisions can help mitigate emerging environmental inequity. We could move AI workloads around to balance AI’s water footprint across different regions equitably rather than letting a few disadvantaged and drought-hit regions bear the negative impact disproportionately.
Everyone has a shared responsibility to cut water usage as we’re battling a global water shortage and drought challenges. AI undoubtedly holds enormous potential to make our world a better place and help us address many challenges, including water scarcity. But this doesn’t mean we should let AI models guzzle our vital and finite water resources under the radar. Instead, every drop matters and AI should clearly lead by example to address its own water footprint while helping to combat global water challenges. Although many potential solutions can slash AI’s water footprint, the first step is simple: measure AI’s water footprint and make it public.