Human, or human-like? Transparency for AI-generated content
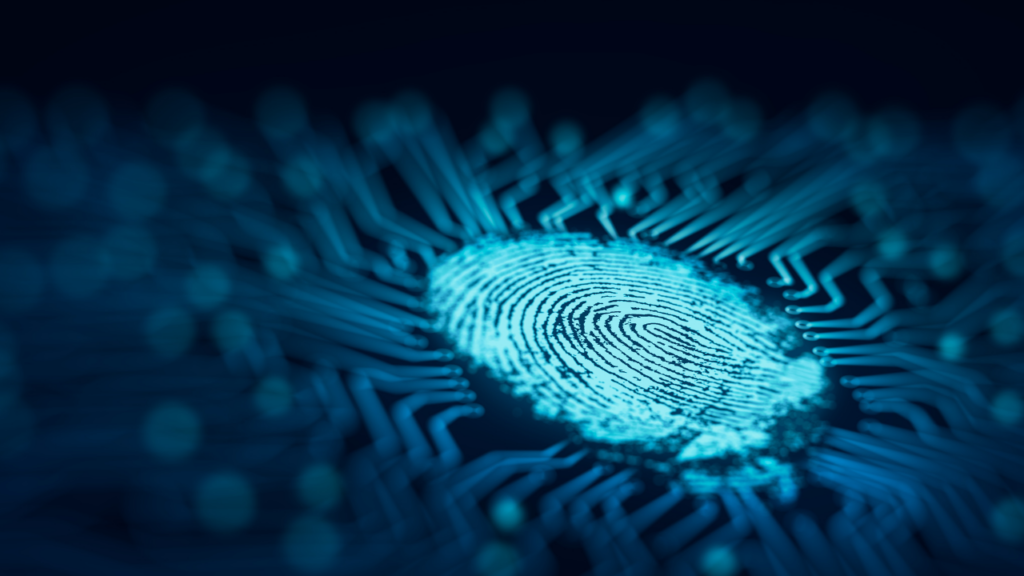
AI has demonstrated many remarkable achievements in its development, from automated translation and game-playing to self-driving cars and medical diagnosis tools. But recently, AI has acquired a brand new ability: to generate convincing novel content at a user’s request. A new generation of AI systems can generate convincing content in various domains. Large language models like ChatGPT can generate humanlike linguistic content, and diffusion models like MidJourney can generate realistic images. In combination, these technologies can generate other kinds of content.
All AI systems that have found widespread use in society have had significant influence. But the arrival of AI systems that can generate convincing content opens the door to a new category of influence. This is because content endures and affects everyone who encounters it. The influence of a content-generating AI system endures for as long as the generated content endures. This influence is particularly telling because enduring human-generated content is a key material from which human culture is built.
In a recent article, Yuval Noah Harari noted that language ‘is the stuff almost all human culture is made of’: human laws, political systems and mythologies are created and transmitted in language. Other components of culture reside in human-generated images and music. Machines can convincingly produce all of these forms of content. For Harari, AI systems that can generate such content have ‘hacked the operating system of our civilisation’. For this reason, they deserve special scrutiny.
Until recently, demands for AI transparency have focused on transparency about processes. The key transparency demands have concerned where AI systems operate in government and commerce, how they work or how accurate or biased they are, and how they affect their users, for better or worse. But if AI systems can place static content into the world, oversight processes must also extend to that generated content. Specifically, human consumers of content should have a way of knowing whether a human or a machine made a given piece of content they encounter.
If content transparency is not forthcoming, the consequences on culture may be dramatic. Harari suggests a culture of AI-generated content could trap humans ‘behind a curtain of illusions’ and imperil democracy. Daniel Dennett, in a recent warning about the dangers of ‘counterfeit people’, argues that the widespread proliferation of AI-generated content will ‘undermine the trust on which society depends’ and ‘risks destroying our civilisation’. Both writers call for urgent precautionary measures, which require AIs and the content they generate to be identifiable as such.
In policymaking terms, it is useful to distinguish between transparency governing direct interactions with AI, for instance, in conversations with chatbots, and transparency around AI-generated content more generally. The former issue is already the subject of actual or forthcoming legislation in several jurisdictions. We are concerned with the latter issue. A user can take content produced in a direct AI interaction and pass it on without disclosing its AI origin—for instance, by posting it on social media or submitting it to a teacher or work colleague.
What mechanisms could provide transparency about AI-generated content in indirect encounters? The mechanism will have to operate on arbitrary items of content encountered in arbitrary contexts. The quest for content transparency mechanisms takes centre stage in Joe Biden’s recent Executive Order on AI, which calls for the establishment of ‘standards and best practices for detecting AI-generated content and authenticating official content’. US policymakers are undoubtedly thinking about ways to safeguard democratic processes in the forthcoming US election.
A provenance scheme to encode contents’ history
Broadly, two mechanisms have been proposed to deliver content transparency— Biden’s executive order refers to both of them. One proposal calls for introducing a provenance scheme whereby the software ecosystem that creates, modifies, transmits and displays online content is instrumented so that each content item can carry an encoding of its complete history since creation. This scheme addresses very broad authentication issues, potentially allowing authentication of human-generated and AI-generated content.
The idea is to tie content to known producers because attitudes towards content depend heavily on provenance. An item flagged as originating from a trusted producer, perhaps an individual or news outlet, may be trusted; an item flagged as AI-generated may be treated more cautiously. A provenance scheme would undoubtedly be extremely useful. But to work, it would have to be adopted across a whole software ecosystem for creating, transmitting, modifying and displaying content.
Some initiatives seek to bring about the necessary changes. In particular, the Content Authenticity Initiative, but the goal is undeniably an ambitious one. Creating a scheme in which provenance information cannot be altered or ‘spoofed’ will also be technically challenging. Blockchain methods show some promise in addressing these challenges, but work is still needed to show how they would operate.
AI-generated content detectors
The other proposal for content transparency turns on using detectors for AI-generated content. This scheme has a narrower scope than a provenance scheme. The detector would tell a consumer whether AI generated a given item and provide no further provenance information. It would also indicate if an AI system produced parts of the item and if these parts are large and distinct enough: no detection scheme can be reliable for short texts. Again there are technical challenges to overcome here.
As AI content generators improve, it will become increasingly hard to distinguish the content they produce from human-generated content. But there is an important possibility to consider: if AI content generators are designed to support detection, the prospects for reliable detection are much better. Already, many AI generators include ‘watermarks’ in the content they produce.
But there are other ways of supporting detection which are less commonly discussed. In particular, a generator can keep a private log of all the content it generates: a detector can then be implemented as a ‘plagiarism detector’ that consults this private log. Plagiarism detection is old technology, relying on an Information Retrieval (IR) process similar to web search.
Again, attention must certainly be paid to strategies for evading detection. For instance, changing some words in an AI-generated text can effectively evade watermarking schemes. However, IR methods are more resistant to these strategies, and companies already have much experience countering them. This IR-based method raises questions of its own, particularly regarding privacy. But there is active research in IR on sensitive datasets, and big tech companies are the acknowledged leaders in IR; we think there are prospects for technical solutions here too.
We believe policymakers should pursue provenance and detection schemes per Biden’s Executive Order. But in the short term, we feel the most practical regulatory action is to require any organisation developing an AI content generator to demonstrate and make a reliable detection tool for the content it generates freely available as a condition of its release to the public.
Our proposal and the EU trilogue negotiations
We have published two papers in the project we are working on that articulate this proposal in more detail. Our first paper generated considerable interest among policymakers. The EU Parliament adopted the concept in its proposed amendments to the AI Act catering for generative AI models; these amendments are currently the subject of trilogue negotiations in the EU, which will conclude very soon. Our paper was also discussed at the US Senate’s recent Judiciary Hearing on AI Oversight, where two co-authors, Yoshua Bengio and Stuart Russell, gave expert evidence. We have also had productive discussions with AI groups at the OECD and the EU Commission. Our second paper was published last month. It summarises our proposal and our many discussions since we first expressed it.
Our proposal raises many questions about technical feasibility, costing, and the practicalities of enforcement: our second paper enumerates these questions and discusses them. For example, how would a requirement to support detection be enforced for open-source content generators? We argue a pragmatic solution is possible because the large set of open-source generators derives from a small number of systems produced by the largest companies. If large companies’ models must support detection, and licenses for open-source use require this support to be maintained, support will also percolate into the open-source ecosystem. Our proposed scheme will certainly not prevent all malicious use of AI content generation: for instance, malicious state actors will not be bound by it. But it would help to create transparency about the great majority of AI-generated content, which is, for us, Harari, Dennet and many others, a vital objective for the health of our society. We argue that responsibility for AI-generated content rests morally and practically with the organisations that build the generators. We call for a rule that recognises this responsibility and obliges these organisations to ensure transparency about AI content.