Lawsuits in the United States point to a need for AI risk management systems
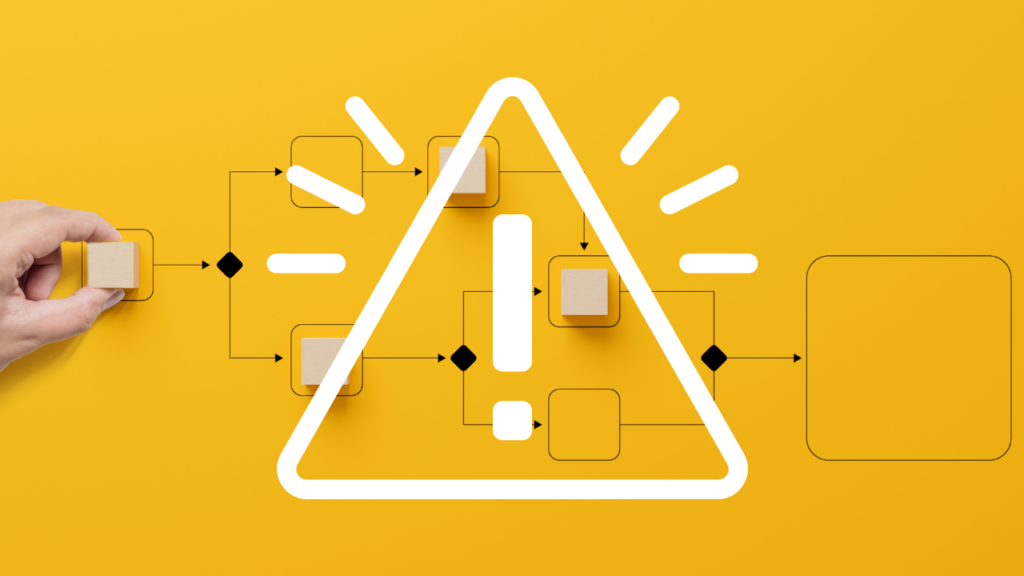
Despite its efficiencies and innovative capacities, the increasing use of Artificial Intelligence (AI) in high-risk applications raises important legal and ethical questions, with serious repercussions for those affected by its misuse. Legal battles and class actions show that the unsafe, inappropriate and even abusive uses of AI are beginning to accumulate and point to a need for some regulation to reign in the associated risks.
The consequences of AI misuse can be and have been severe, from job losses, asset seizure, and wrongful foster care placements. Threats to individuals’ life chances have left many to seek redress. Residents of the Netherlands experienced this first-hand when 1.4 million people were wrongfully flagged as fraud by an AI-powered risk profile system over more than a decade. Michigan, too, faced a $20 million settlement after 40,000 state residents were wrongfully accused of fraud by an automated system. To prevent devastating harms and loss of opportunity, AI systems must be governed with an ethical approach and equipped with safeguards to protect individuals from misuse.
HR tech lawsuits focus on discrimination
HR is a sector that is particularly capitalizing on automation and the interactive solutions offered by AI in talent management practices. However, a number of players in this space have come under fire due to the misuse of automated recruitment tools. In the US, the Equal Employment Opportunity Commission (EEOC) has sued the iTutorGroup, which provides English language tutoring in China, for age-based discrimination. The group allegedly used an algorithm in the US in 2020 that automatically rejected older applicants due to their age, with women over 55 and males over 60 being disqualified from consideration. This violates the Age Discrimination in Employment Act, where those aged 40 and over are protected against discrimination. The EEOC is suing for back pay and liquidated damages for the more than 200 applicants.
More recently, also in the HR tech sector, a class action lawsuit has been brought against Enterprise Management Cloud company Workday. As a provider of leading Applicant Tracking Software (ATS), Workday serves over 55 million users and is used by several Fortune 500 companies. However, Plaintiff Derek Mobey filed a complaint about the company in the District Court of California in February in 2023 for alleged racial, disability, and age discrimination. As an African American applicant with anxiety and depression who is over 40, Mobley claims that the AI systems used by Workday, which rely on algorithms and inputs created by humans, disproportionately impact and disqualify Black, disabled, and older job applicants.
Mobley brought the complaint against Workday following a number of failed applications to companies thought to be using Workday, having applied to and been rejected from approximately 80-100 positions since 2018 despite holding a Bachelor’s degree in Finance and an Associate’s Degree in Network Systems Administration. He alleges that human involvement in creating the screening tools caused discrimination since humans developing the algorithms have built-in biases of their own. The selection tools are also marketed as allowing employers to manipulate and configure them in a subjective and discriminatory manner.
Since the alleged discrimination based on race, age, and disability would violate Title VII of the Civil rights Act 1964 (which specifically prohibits discrimination in terms of employment: hiring, compensation etc.), the Civil Rights Act 1866, the Age Discrimination in Employment Act of 1967, and the ADA Amendments Act of 2008, such unjustified discrimination would violate federal law. Thus, Mobley has brought the case on behalf of himself and others similarly situated, including all African American applicants, including former applicants, applicants over 40, and disabled applicants who, between 3 June 2019 and February 2023, have not been referred or permanently hired for employment as a result of the discriminatory screening process. The sought resolution for this is injunctions preventing Workday, and its customers, from engaging in these discriminatory practices; an order providing that Workday institute and carry out policies that provide equal employment opportunities for minorities; punitive damages, attorneys fees and costs; and compensatory damages.
However, in a statement issued by a company spokesperson, Workday has expressed that the lawsuit is without merit. They claim that they act responsibly and transparently and are committed to trustworthy AI, using a risk-based review process throughout the design and deployment of their products to mitigate potential harms. In contrast to the lawsuit, they claim to undergo extensive legal reviews to ensure compliance with relevant legislation.
Insurance tech lawsuits
Outside of the HR sector, in the critical insurance industry, it is also being evidenced how algorithms discriminate against customers, adversely affecting their life opportunities. A case brought against exemplifies this. In late 2022, Jacqueline Huskey filed a class-action suit against State Farm under the U.S. District Court for the Northern District of Illinois, claiming State Farm discriminates against black policyholders.
According to the lawsuit, State Farm’s algorithms and tools display levels of bias in the way they analyse data. For example, their use of natural language processing creates a negative bias in voice analytics for black speakers and a negative association of typically “African American” names versus white names. The case cites a study from the Center on Race, Inequality and the Law at the NYU School of Law that surveyed 800 black and white homeowners with State Farm policies (648 white and 151 Black). The survey found that there are disparities between the way claims from white policyholders and black policyholders are met, with black policyholders having more delays, more correspondences with the State Farm agents, and overall, their claims being met with more suspicion than that of white homeowners – leading to negative impacts. Similar to the Workday case and in violation of federal law, specifically the Fair Housing Act (42 U.S. Code § 3604 (a)(b)) which covers discrimination in the sale or rental of housing and other prohibited practices.
Would due diligence help to avoid discrimination cases?
While the outcomes of both State Farm cases are pending, they present evidence of disparate impact on those from marginalised groups and a growing need for redress. It is no longer the case that people are unaware of the ways algorithms and AI have been working, perhaps unintentionally, against them. As such, vendors and users of AI systems must be made responsible for minimising bias and harm.
In HR tech, the insurance industry and elsewhere, many vendors use third-party algorithms, meaning they may not be fully aware of the model’s capabilities or disparities in performance for particular groups. Although there is a lack of transparency about the algorithms used by Workday, the platform integrations with third-party providers so it is likely that at least some of the automated systems used by Workday are outsourced.
State Farm’s outsourcing is more transparent. They use algorithms and tools from a variety of third-party vendors to automate aspects of claims processing, limit the likelihood of fraudulent claims and assess which cases are the most complex. One vendor that may have caused State Farm’s disparity is Duck Creek Technologies, “an insurance-specific software and analytics company that provides comprehensive claims management and fraud-detection tools”.
Both State Farm and Workday integrate systems from third-party providers to perform the tasks that are at the centre of legal actions, highlighting the importance of carrying out due diligence when outsourcing AI systems. If for no other reason than simply because it is the entity that uses the algorithm that is liable for any harm. To protect themselves and others, entities must inquire about how systems work and any known potential for bias before procurement, as well as continually monitor AI systems’ outputs for bias and other harmful outcomes while deployed.
Risk management through explainability, robust systems and privacy
In light of the above lawsuits and several cases of harm from the misuse of AI in recent years, there is a growing need for AI Risk Management. The National Institute of Standards and Technology (NIST) released the first version of its AI Risk Management Framework (AI RMF 1.0) in January 2023. To ensure that AI algorithms are safe, secure, and compliant with applicable regulations, anyone using AI in their businesses should adopt an AI risk management framework.
Steps must be taken to measure and mitigate bias to prevent discriminatory treatment and other negative and unfair outcomes. Explainability and transparency can help.Clear and meaningful explanations of a system’s outcomes are essential to build and maintain users’ trust.
Explainability was useful when the Apple Card caused a stir, and customers accused Goldman Sachs, its issuer, of gender discrimination. After the New York Department of Financial Services conducted a statistical analysis of nearly 400,000 applicants in the state, it determined that the algorithm used by Goldman Sachs had not been discriminatory. This was further reinforced when Goldman Sachs explained the factors it considered when making decisions.
Explainable AI systems provide users with the ability to understand and challenge decisions, as well as seek redress if needed. Thanks to explainability, Goldman Sachs was able to clear their name. Explainability processes that document an AI system’s lifecycle can help to reduce risks. There are also tools available to better interpret a model’s decisions that do things like help understand how different features are weighted.
Robustness and security prevent AI harms
AI systems must be able to persist and perform well despite potential digital security risks or misuse. The launch of Microsoft’s Twitter chatbot, Tay, gained notoriety when users noticed the lack of filters on the system. This allowed internet trolls to feed Tay profane and offensive tweets, causing the bot to mimic them and consequently post offensive tweets. Microsoft had intended for the chatbot to have “casual and playful conversation,” but they had to shut it down only 16 hours after its launch due to the offensive content it had been posting.
To ensure robustness and reduce security risks, AI models can be improved by generalising their performance, retraining on new data, employing adversarial training to detect attacks, and continuously monitoring for signs of failure. These measures can help ensure a system’s resilience and reduce the chances of it being exploited.
Personal data and privacy integrity calls for built-in protections
Algorithms should have built-in safety measures to ensure they do not leak sensitive or personal data. The Mutnick v. Clearview AI case highlights the importance of privacy practices to protect against data breaches and unlawful processing and to ensure consent for the use of personal data.
Clearview AI created a facial recognition database of millions of Americans without their knowledge or consent by using 3 billion photos scraped from online social media and other internet-based platforms such as Venmo and sold it to over 600 law enforcement agencies and private entities.
Some steps can help ensure that users are not identifiable by the system. When assessing the privacy risks of an algorithm, it is important to consider the type of data used and the amount retained. Actors can minimise the amount of data collected by doing such things as reducing the training data, perturbing it, anonymising or pseudonymising it, or using models in a de-centralised or federated way.
Confronting unknown AI risks
All risks associated with AI must be considered to prevent potential harm, whether known or unknown. To identify and address the unknowns, companies can conduct risk and effect analyses at various stages of implementation. An effective risk management framework is crucial for developing reliable AI, improving its performance, and avoiding legal action and reputational damage. Inventory documentation and periodical and central monitoring with an escalation process are key components of this framework. Proper documentation throughout the AI lifecycle can enhance control of the system and the risk management process. While it is impossible to predict all potential risks, implementing AI risk management can help reduce the likelihood of future harm and mitigate legal liability.
Emre Kazim and Adriano Koshiyama also contributed to this post.