Navigating the environmental impact of AI
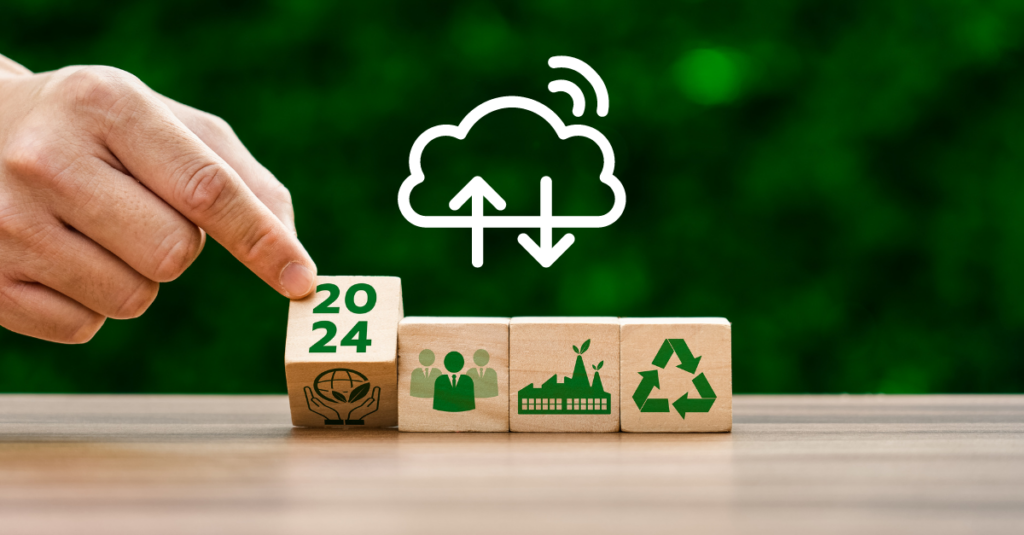
Artificial Intelligence (AI) offers unprecedented opportunities for innovation, efficiency, and problem-solving across diverse domains. It can potentially drive positive transformation, from revolutionising healthcare and finance to enhancing customer experiences and optimising industrial processes.
But as we delve deeper into the era of AI, it is crucial to recognise its environmental implications. The rapid proliferation of AI technologies, particularly large-scale language models, broadly called generative AI, has raised awareness on the significant energy consumption and carbon emissions associated with their development and deployment. Organisations are now reevaluating their approach to technological innovation in the context of sustainability and environmental responsibility.
AI’s energy consumption and carbon emissions
Training an AI model is a computationally intensive process, and the latest models emitted significant amounts of CO2e during their development. For example, the training of GPT-3, the model ChatGPT was initially based on, is reported to have caused the emission of 552 tCO2e, which is approximately equivalent to driving a car around the equator 19 times. This highlights the environmental impact of AI development and emphasises the need for sustainable practices in the field.
Daily use of AI models in production is also very energy-consuming. Each user request triggers a complex operation. While a conventional API request only initiates a simple database lookup, a query to an AI model results in a computationally expensive operation where billions of parameters are activated to produce an output. These processes create a surge in data centre energy consumption globally. In fact, some suggest the increased usage of AI could result in a doubling of energy demands in the near future. With this kind of growth, all actors must examine the environmental implications of AI deployment to gain better control of the situation.
Assessing AI’s environmental impact
AXA sees working with precise metrics as crucial to addressing AI’s environmental impact and acting efficiently. Only by measuring and understanding the carbon footprint of our AI use can we anticipate the impact of its future use at scale.
Our data science teams began estimating AI models’ energy consumption and carbon emissions while collecting and analysing any available emissions data. However, this is challenging because most cloud providers, including those Axa uses, do not provide data on CO2 emissions explicitly related to generative AI services. Until data is available, we base our projections on expert estimates. Studies are usually extrapolated from smaller open-source models where data is available.
In addition to the architectural details of the model, it is essential to consider the carbon emission factor of the region where the service is operated. The emissions intensity of electricity generation can vary significantly from country to country, and accordingly, these differences can significantly impact the results. Our latest scenarios for GPT-3 indicate an energy consumption of 8–51 Wh per request. This corresponds to emissions of 3-8 g CO2e per request, depending on whether we assume the servers are in France or the Western United States. In comparison, a traditional Google search query was reported to cause 0.2 g CO2, representing a factor 40 maximum.
These findings are still rough estimates, and we expect cloud providers to become transparent about their footprint to improve our calculations. AXA primarily relies on conventional AI, which produces specific outputs such as decisions or values, as seen in underwriting or pricing. This type of technology consumes much less power compared to Generative AI. However, our approach is a first step in anticipating potential impacts and guiding our decisions to mitigate the carbon footprint of AI operations.
Balancing usage and environmental sustainability
Ultimately, there needs to be a balance between the use of this technology and environmental responsibility. As AI use grows, organisations must consider environmental implications and make informed decisions that align with sustainability goals. Enhanced transparency about the environmental footprint of AI-related services by cloud providers is vital for strategic planning. By fostering transparency and accountability, we can collectively work towards minimising the environmental impact of AI.
AXA is putting effort into raising awareness internally and promoting a well-considered and balanced use of AI to do its part in reducing the environmental impact of AI. We provide educational training programs for employees on the responsible use of Generative AI, which covers its environmental impact. We believe AI should not be the technology choice by default. We keep track of the latest advancements in chip architecture and conduct research to understand how models can achieve high performance for specific tasks while becoming less computationally demanding. Additionally, the performance of large general-purpose models should be compared to that of smaller, specialised models. Employing pre-trained off-the-shelf general-purpose models avoids training costs. However, such models consume much more energy in operation than customised smaller models when used at scale.
Embracing artificial intelligence responsibly
The widespread adoption of AI presents opportunities and challenges, especially regarding its environmental impact. Organisations should strive to minimise their carbon footprint while leveraging AI’s potential for positive outcomes. Acknowledging the importance of balancing technological advancement and environmental responsibility is critical. A proactive approach is necessary to address environmental implications, so efforts should be made to estimate the energy consumption and carbon emissions associated with AI. By following this path, organisations can promote a sustainable and ethical approach to technological innovation.