How the OECD’s AI system classification work added to a year of progress in AI governance
Despite the COVID pandemic, we can look back on 2020 as a year of positive achievement in progress towards understanding what is needed in the governance and regulation of AI.
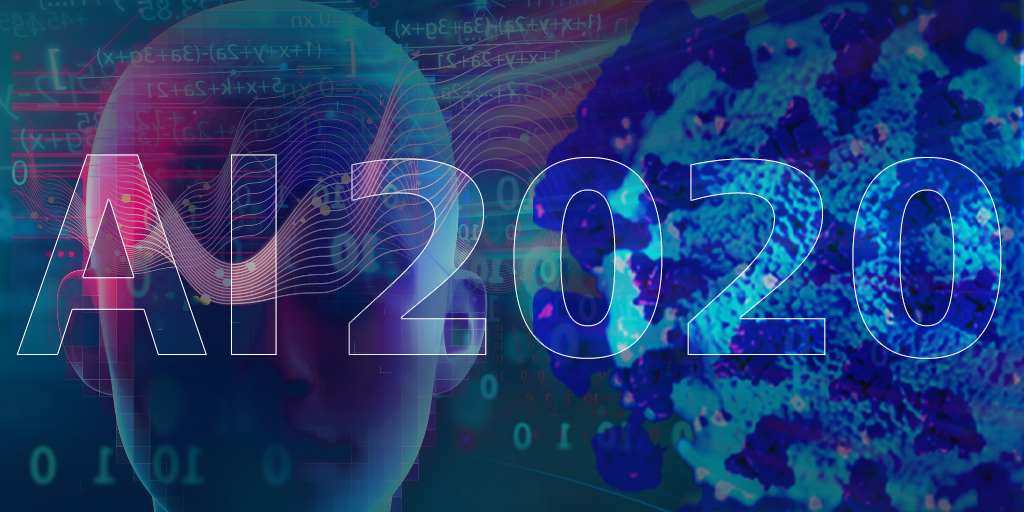
It has never been clearer, particularly after this year of COVID and our ever greater reliance on digital technology, that we need to retain public trust in the adoption of AI.
To do that we need, whilst realizing the opportunities, to mitigate the risks involved in the application of AI. This brings with it the need for a clear standard of accountability.
A year of operationalizing AI ethical principles
2019 was the year of the formulation of high-level ethical principles for AI by the OECD, EU and G20. These are very comprehensive and provide the basis for a common set of international standards but it has become clear that voluntary ethical guidelines are not enough to guarantee ethical AI.
There comes a point where the risks attendant on non-compliance with ethical principles is so high that policy makers need to understand when certain forms of AI development and adoption require enhanced governance or and/or regulation. The key factor in 2020 has been the work done at international level in the Council of Europe, OECD and EU towards operationalizing these principles in a risk-based approach to regulation.
And they have been very complementary. The Council of Europe’s Ad Hoc Committee on AI (CAHAI) has drawn up a Feasibility Study for regulation of AI which advocates a risk-based approach to regulation as does last year’s EU White Paper on AI.
As the EU White Paper said: “As a matter of principle, the new regulatory framework for AI should be effective to achieve its objectives while not being excessively prescriptive so that it could create a disproportionate burden, especially for SMEs. To strike this balance, the Commission is of the view that it should follow a risk-based approach”
They go on to say:
“A risk-based approach is important to help ensure that the regulatory intervention is proportionate. However, it requires clear criteria to differentiate between the different AI applications, in particular in relation to the question whether or not they are ‘high-risk’ . The determination of what is a high-risk AI application should be clear and easily understandable and applicable for all parties concerned.”
The feasibility study develops this further with discussion about the nature of the risks particularly to fundamental rights, democracy and the rule of law.
As the Study says: “These, risks, however, depend on the application context, technology and stakeholders involved. To counter any stifling of socially beneficial AI innovation, and to ensure that the benefits of this technology can be reaped fully while adequately tackling its risks, the CAHAI recommends that a future Council of Europe legal framework on AI should pursue a risk-based approach targeting the specific application context. This means not only that the risks posed by AI systems should be assessed and reviewed on a systematic and regular basis, but also that any mitigating measures …should be specifically tailored to these risks.”
This means not only that the risks posed by AI systems should be assessed and reviewed on a systematic and regular basis, but also that any mitigating measures …should be specifically tailored to these risks.
EU White Paper on AI
Governance must match the level of risk
Nonetheless, it is a complex matter to assess the nature of AI applications and their contexts. The same goes for the consequent risks of taking this forward into models of governance and regulation. If we aspire to a risk-based regulatory and governance approach we need to be able to calibrate the risk. This will in turn determine the necessary level of control.
Given this kind of calibration, there is a clear governance hierarchy to follow, depending on the rising risk involved. Where the risk is lower, actors can adopt a flexible approach such as a voluntary ethical code without a hard compliance mechanism. Where the risk is higher, they will need to institute enhanced corporate governance using business guidelines and standards, with clear disclosure and compliance mechanisms.
Then we have government best practice, such as the AI procurement Guidelines developed by the World Economic Forum and adopted by the UK government. Finally, and, as some would say, as a final resort, we introduce comprehensive regulation, such as that which is being adopted for autonomous vehicles, which is enforceable by law.
In regulating, developers need to be able to take full advantage of regulatory sandboxing which permits the testing of a new technology without the threat of regulatory enforcement but with strict overview and individual formal and informal guidance from the regulator.
There are any number of questions which arise in considering this governance hierarchy, but above all, we must ask ourselves if we have the necessary tools for risk assessment and a clear understanding of the necessary escalation in compliance mechanisms to match.
As has been well illustrated during the COVID pandemic, the language of risk is fraught with misunderstanding. When it comes to AI technologies, we need to assess the risks such as the likely impact and probability of harm, the importance and sensitivity of use of data, the application within a particular sector, the risk of non-compliance and whether a human in the loop mitigates risk to any degree.
AI systems classification framework at the OECD
The detailed and authoritative classification work carried out by the OECD Network of Experts Working Group on the Classification of AI systems comes at a crucial and timely point.
The preliminary classification framework of AI systems comprises 4 key pillars:
- Context: This refers to who is deploying the AI system and in what environment. This includes several considerations such as the business sector, the breadth of deployment, the system maturity, the stakeholders impacted and the overall purpose, such as for profit or not for profit.
- Data and Input: This refers to the provenance of the data the system uses, where and by whom it has been collected, the way it evolves and is updated, its scale and structure and whether it is public or private or personal and its quality.
- The AI Model, i.e. the underlying particularities that make up the AI system – is it, for instance, a neural network or a linear model? Supervised or unsupervised? A discriminative or generative model, probabilistic or non-probabilistic? How does it acquire its capabilities? From rules or machine learning? How far does the AI system conform to ethical design principles such as explainability and fairness?
- The Task and Output: This examines what the AI System actually does. What are the outputs that make up the results of its work? Does it forecast, personalize, recognize, or detect events for example?
Within the Context heading, the framework includes consideration of the benefits and risks to individuals in terms of impact on human rights, wellbeing and effects on infrastructure and how critical sectors function. To fit with the CAHAI and EU risk-based approach and be of maximum utility however, this should really be an overarching consideration after all the other elements have been assessed.
Also see: A first look at the OECD’s Framework for the Classification of AI Systems, designed to give policymakers clarity
The fundamental risks of algorithmic decision-making
One of the key questions, of course, is whether on this basis of this kind of classification and risk assessment there are early candidates for regulation.
The Centre for Data Ethics and Innovation created in the UK two years ago recently published their AI Barometer Report. This also discusses risk and regulation and found a common core of risk across sectors.
They say “While the top-rated risks varied from sector to sector, a number of concerns cropped up across most of the contexts we examined. This includes the risks of algorithmic bias, a lack of explainability in algorithmic decision-making, and the failure of those operating technology to seek meaningful consent from people to collect, use and share their data.”
A good example of where some of these issues have already arisen is the use of live facial recognition technologies which is becoming widespread. It is unusual for London’s Metropolitan Police Commissioner to describe a new technology as Orwellian (in reference to his seminal novel “1984” where he coined the phrase “Big Brother”) as she did last year talking about live facial recognition but now they are beginning to adopt it at scale.
In addition, over the past few years we have seen a substantial increase in the adoption of Algorithmic Decision Making and prediction, or ADM, across central and local government in the UK. In criminal justice and policing, algorithms for prediction and decision making are already in use.
Another high-risk AI technology which needs to be added to the candidates for regulation is the use of AI applications for recruitment processes as well as in situations impacting employees’ rights to privacy.
Future decision-making processes in financial services may be considered high risk and become candidates for regulation. This concerns areas such as credit scoring or determining insurance premiums by AI systems.
AI risk and regulation in 2021 and beyond
The debate over hard and soft law in this area is by no means concluded. Denmark and a number of other EU member states have recently felt the need to put a stake in the ground with what is called a non-paper to the EU Commission over concerns that AI and other digital technologies may be overregulated in the EU’s plans for digital regulation.
Whether in the public or private sector, the cardinal principle must be that AI needs to be our servant not our master. Going forward, there is cause for optimism that experts, policy makers and regulators now recognize that there are varying degrees of risk in AI systems. We can classify and calibrate AI and develop the appropriate policies and solutions to ensure safety and trust. We can all as a result expect further progress in 2021.