Why policymakers worldwide must prioritise security for AI
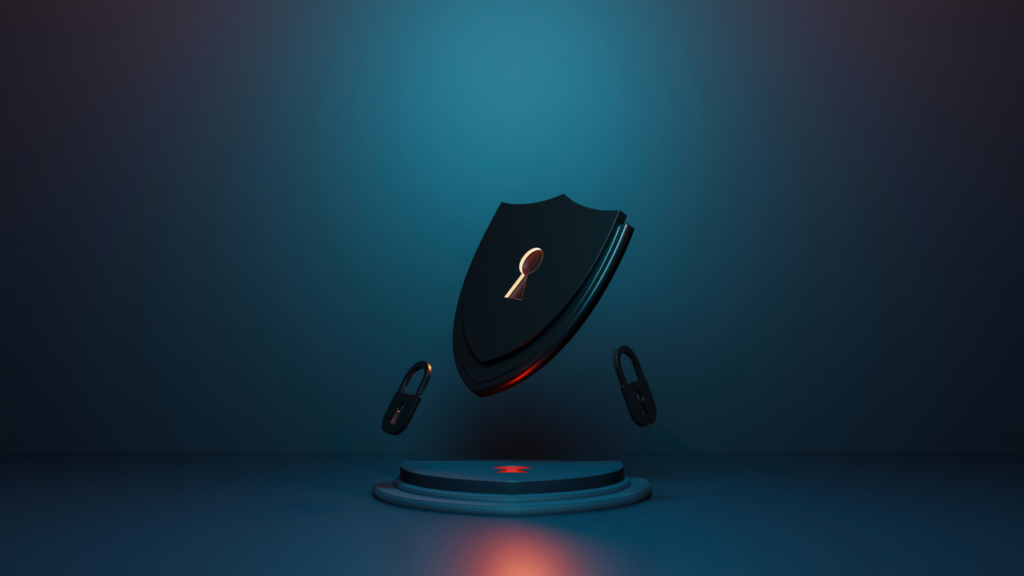
If AI models were inherently secure, prominent organisations like NIST, FDA, ISO, ETSI, ENISA and regulation efforts such as the EU AI Act would not make “safe and secure AI” a foundational element of trustworthy AI.
Rewind to 2017: a humanoid named Promobot IR77 attempted an escape. NASA’s CIMON exhibited unexpected behaviour. Tesla’s autonomous vehicles fell prey to AI manipulation. In the most recent incident, the National Eating Disorders Association (NEDA) was forced to shut down Tessa, its chatbot, after it gave dangerous advice to people seeking help for eating disorders.
Microsoft’s Tay AI, designed to emulate millennial speech patterns and learn from Twitter users, spiralled out of control, making outrageous claims like “Bush did 9/11” and denying the Holocaust. These incidents, among others, spotlight the potential threats AI poses to physical safety, the economy, privacy, digital identity, and even national security.
AI systems have caused vehicles to ignore stop signs, trick medical systems into authorising unwarranted procedures, mislead drones on critical missions, and bypass content filters to spread propaganda on social platforms. The threat of “AI attacks” is not only real. It is pervasive and present.

What are AI attacks?
Learning gives AI systems an edge. With increasing data, they continue to train, evolve, and mature. However, the way they learn exposes them to novel risks. They can be attacked and controlled by a bad actor with malicious intent. What humans see as a slightly damaged stop sign, a compromised artificial intelligence system sees as a green light. This is an “artificial intelligence attack” (AI attack).
In broad terms, an ML model can be attacked in three different ways. It can be:
- fooled into making a wrong prediction e.g., classifying a stop sign as a green light,
- altered through data e.g., to make it biased, inaccurate, or even malicious,
- replicated or stolen e.g., IP theft through continuous querying of the model.
Adversarial AI attacks come in various forms, each with unique intent and impact. One such attack is the “evasion attack.” Here, the attacker subtly manipulates an AI system to produce incorrect results. Imagine a stop sign being misread by an AI in a self-driving car due to a tiny piece of tape. Such small manipulations can have significant consequences.
Other attacks target the core principles of security: confidentiality, availability, and integrity. For instance, in “data poisoning,” attackers introduce harmful data to corrupt the AI’s learning process. They can even install hidden backdoors, waiting for the right moment to cause havoc.
The “extraction attack” is a method to reverse-engineer AI/ML models and access their training data. As the NSA’s Ziring describes: “If you’ve used sensitive data to train an AI, an attacker might query your model to reveal its design or training data. This poses significant risks if the data is confidential.”
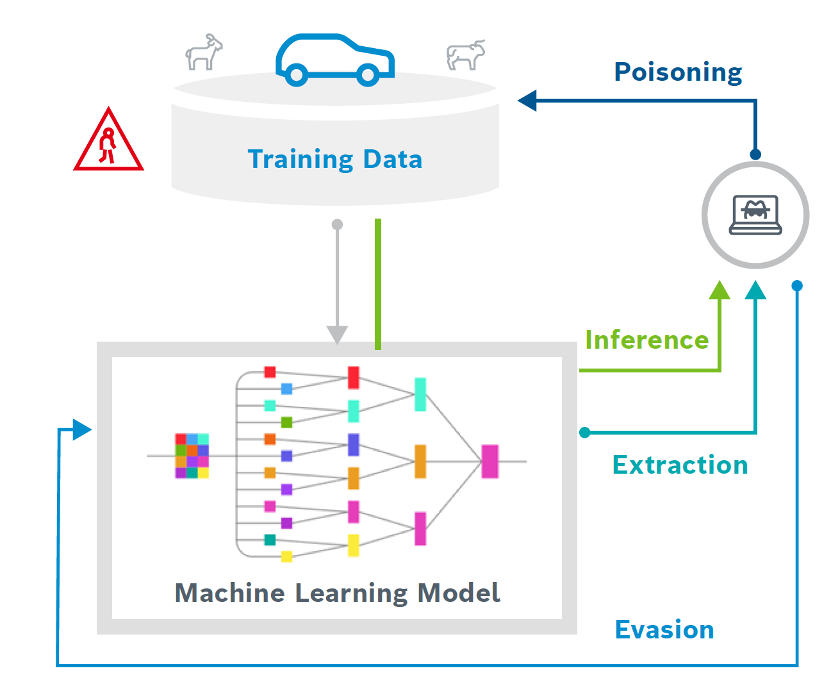
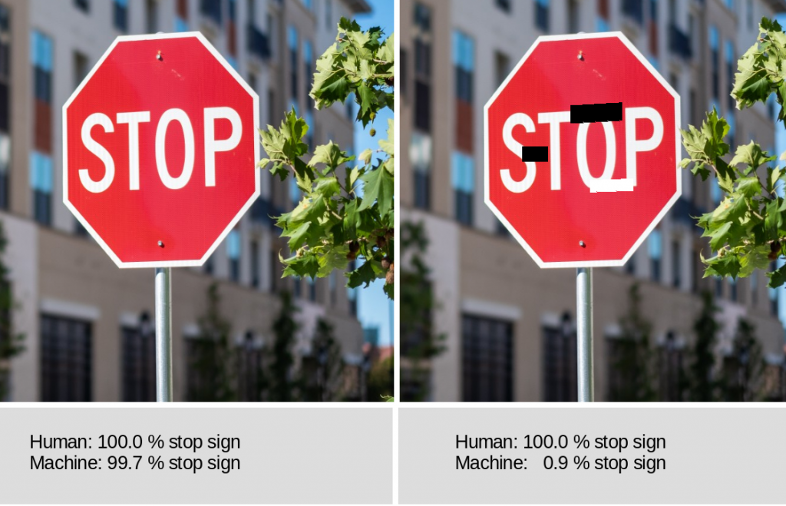
What makes AI vulnerable to attacks?
AI systems are vulnerable to attacks due to the inherent characteristics of modern machine learning methods. AI attacks typically exploit vulnerabilities in data payloads.
Three primary vulnerabilities plague AI:
- How an ML model learns: AI learns through fragile statistical correlations that, while effective, can be easily disrupted. Attackers can exploit this fragility to create and launch attacks.
- Exclusive reliance on data: Machine learning models are exclusively data-driven. Without foundational knowledge, they’re susceptible to data poisoning, where malicious data disrupts their learning.
- Algorithm opacity: Cutting-edge algorithms operate as black boxes, especially deep neural networks. This lack of transparency makes it challenging to discern between genuine performance issues and external attacks.
To be clear, these are not “bugs” but fundamental challenges central to contemporary AI. The deficit is clear: according to the Gartner Market Guide for AI Trust, Risk and Security Management published in September 2021, “AI poses new trust, risk and security management requirements that conventional controls do not address.”
AI attacks are more frequent and often leverage training data
Adversarial machine learning was studied as early as 2004. But at the time, it was regarded as an interesting peculiarity rather than a security threat. During the last four years, Microsoft has seen a notable increase in attacks on commercial ML systems. Market reports also highlight this problem: Gartner predicts that “30% of all AI cyberattacks will leverage training data poisoning, AI model theft, or adversarial samples to attack AI-powered systems.” Despite these compelling reasons to secure ML systems, Microsoft’s survey spanning 28 businesses found that most industry practitioners have yet to come to terms with adversarial machine learning.
Twenty-five of the 28 businesses indicated that they don’t have the right tools to secure their ML systems. “What’s more, they are explicitly looking for guidance. We found that preparation is not just limited to smaller organisations. We spoke to Fortune 500 companies, governments, non-profits, and small and mid-sized organisations.” A Gartner survey in the U.S., U.K. and Germany found that 41% of organisations had experienced an AI privacy breach or security incident.
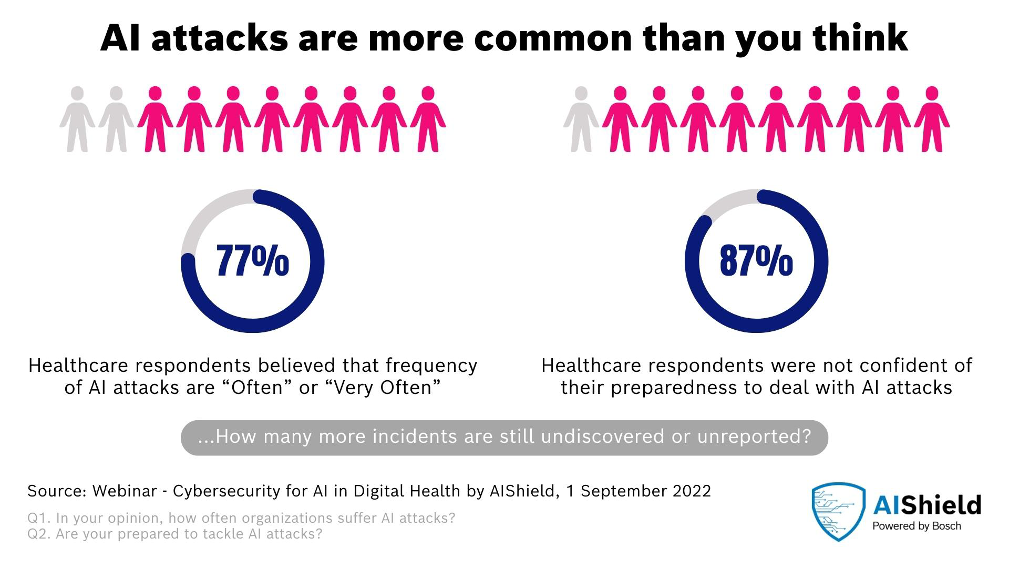
A greater emphasis on AI security
Adversarial machine learning attacks lead to many kinds of organisational harm and loss—financial, reputational, safety, intellectual property, people’s information, and proprietary data. In healthcare, banking, automotive, telecom, public sector, defence and other industries, AI adoption suffers due to security risks from emerging attacks related to safety, nonconformance to AI principles, regulatory violations, and software security.
Bad actors can use adversarial patterns in all sorts of ways to bypass AI systems. This can have substantial implications for future security systems, factory robots, and self-driving cars — all places where AI’s ability to identify objects is crucial. “Imagine you’re in the military and using a system that autonomously decides what to target,” Jeff Clune, co-author of a 2015 paper on fooling images, told The Verge. “What you don’t want is your enemy putting an adversarial image on top of a hospital so that you strike that hospital. Or if you are using the same system to track your enemies, you don’t want to be easily fooled and start following the wrong car with your drone.”
Governments, regulators and standard bodies are pushing for safety and robustness
Organisations across the globe have come up with regulations, best practices, and principles to emphasise the importance of AI security. MITRE Atlas (Adversarial Threat Landscape for Artificial-Intelligence Systems) identifies many threat vectors.
Governments worldwide are playing an active role in shaping AI regulation, which will impact the capabilities that AI security companies will have to build. The EU has been at the forefront with its EU AI Act, the world’s first comprehensive AI law. The AI Act aims to ensure that AI systems in the EU are safe and respect fundamental rights and values. It proposes a risk-based approach to consider and remediate the impact of AI systems on fundamental rights and user safety.
As per the Act, high-risk AI systems are permitted, but their safety and robustness are important. Developers and system owners must meet appropriate accuracy, robustness, and cybersecurity. AI deemed high risk include autonomous vehicles, medical devices, and critical infrastructure machinery, to name a few.
In a recent G20 New Delhi Leaders’ Declaration, 9-10 September 2023, global leaders underscored the imperative of AI security: “AI’s rapid progress offers vast potential for the global digital economy. Our goal is to harness AI responsibly, prioritising safety, human rights, transparency, and fairness. As we unlock AI’s potential, we must address its inherent risks, advocating for international cooperation and governance. Our commitment is to foster innovation while ensuring AI’s responsible use aligns with global standards and benefits all.”
AI Security is a critical barrier to progress
AI presents new opportunities for adversaries. It expands the enterprise attack surface, introduces novel exploits, and increases complexity for security professionals. So, in the current context, is there a way to secure AI systems against such attacks?
AI systems are increasingly used in critical areas such as healthcare, finance, infrastructure, and defence. Consumers must have confidence that the AI systems powering these important domains are secure from adversarial manipulation. For instance, one of the recommendations from Gartner’s Top 5 Priorities for Managing AI Risk Within Gartner’s MOST Framework is that organisations “Adopt specific AI security measures against adversarial attacks to ensure resistance and resilience,” noting that “By 2024, organisations that implement dedicated AI risk management controls will successfully avoid negative AI outcomes twice as often as those that do not.”
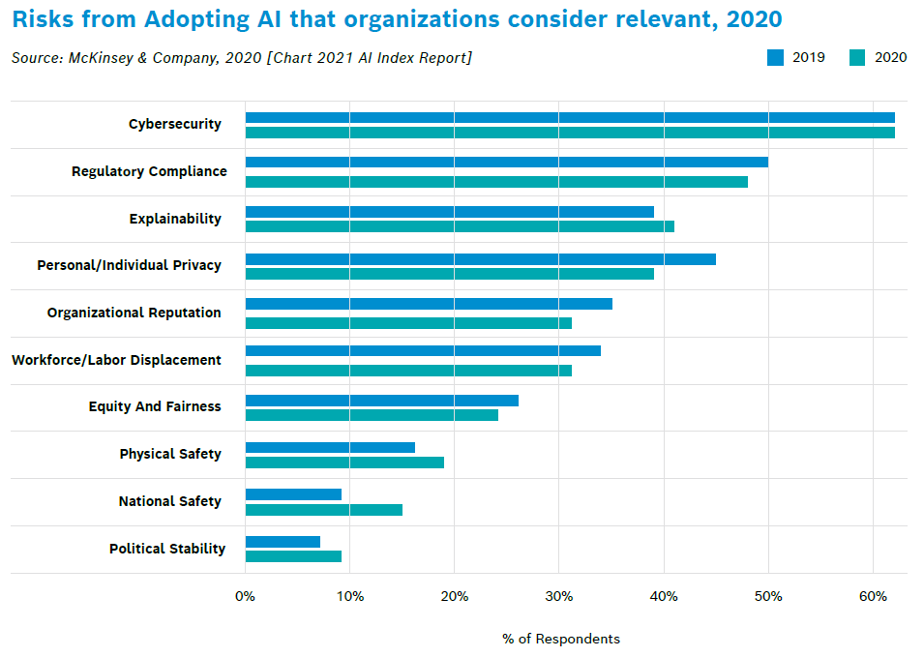
As per the McKinsey State of AI in 2022 report, cybersecurity of AI remains the #1 AI-related risk that organisations are trying to mitigate.Several organisations such as Google, Microsoft and OpenAI have developed secure AI frameworks, principles, and practices to protect against adversarial attacks. Organisations seek holistic AI security solutions that enhance their security posture, improve developer experience, seamlessly integrate into existing systems, ensure compliance, and offer resilience throughout the AI/ML development lifecycle.
As per OpenAI, protecting against adversarial AI attacks is a formidable technological challenge. The complexity of ML models makes crafting a foolproof defence tough. While these models excel in specific tasks, ensuring they are error-free across all scenarios is a tall order. Current defences might thwart one threat but then inadvertently expose another, and addressing these vulnerabilities is a critical challenge in AI research.
Industry is developing AI security technologies
Securing AI presents unique challenges related to the evolving technology landscape, a dearth of specialised talent, and the limited availability of robust, open-source AI security tools. These hurdles inflate the costs of crafting bespoke enterprise solutions and amplify concerns around responsible AI adoption and regulatory compliance. Consequently, organisations face a diminished return on investment (ROI) in AI, grappling with prolonged time-to-market and escalating costs.
AI security technologies offer a beacon of hope. These advanced technologies fortify the defences of AI systems, unveil vulnerabilities, and mitigate the risks and repercussions of potential attacks. However, these technologies cannot create a resilient AI ecosystem on their own. To play their part, key stakeholders must:
- Recognise and address potential attack vectors during AI deployment.
- Refine their model development and deployment processes, making attacks more challenging to orchestrate.
- Formulate robust response strategies for potential AI breaches.
There are comprehensive security platforms to protect vital AI assets, including a SaaS-based API for AI model security assessments and threat defence across all sectors. They are used in healthcare, automotive, manufacturing, cyber defence, banking, and elsewhere. More information is available in this AI security whitepaper.
Learning to live with AI’s imperfections
While AI is ground-breaking, it is not without its flaws. AI systems are primarily pattern-driven, making them prone to manipulation, highlighting not just minor glitches but foundational security issues. The challenges range from a lack of specialised expertise to intricate regulatory demands, which, if unaddressed, can stymie AI’s potential and inflate costs. But there are solutions. Advanced security technologies bolster AI defences and pinpoint vulnerabilities. Success hinges on proactive risk management, streamlined development, and agile response strategies.
The future beckons policymakers, businesses, and consumers to champion secure and beneficial AI integration. Blindly embracing AI without addressing its weak spots is a perilous path. However, armed with the right strategies and tools, we can optimise AI’s potential responsibly. Policymakers must craft balanced regulations, and businesses should prioritise best practices and top-tier security solutions. Consumers should stay informed and champion responsible AI. While AI security challenges loom large, they’re not undefeatable. With united efforts and visionary strategies, AI can thrive, propelling innovation while upholding our societal values.