Can synthetic data enable data sharing in financial services?
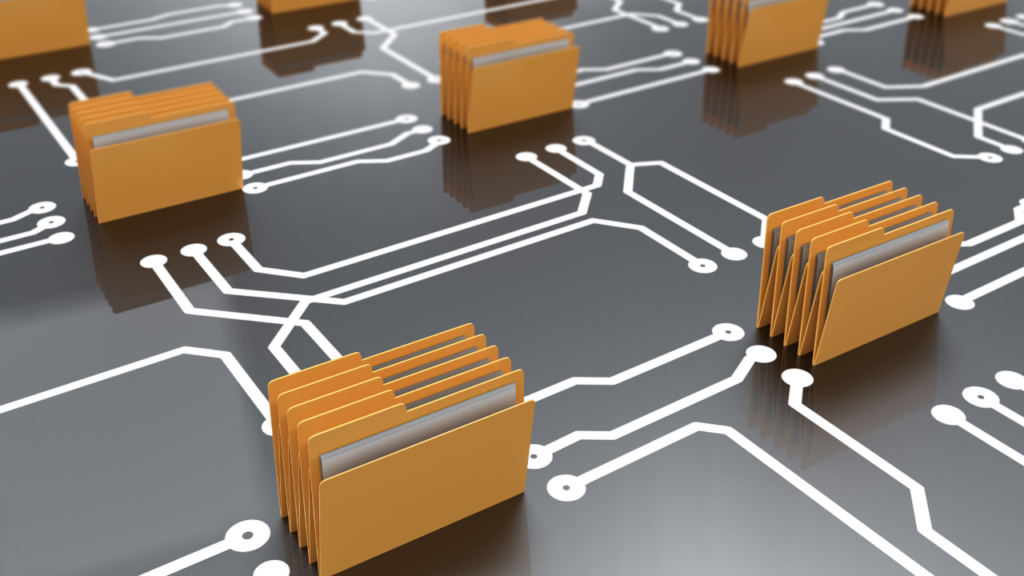
Good data underpins good decision-making. This is as true for humans as it is for computers. But what if the data available are so sensitive that processing them would be unlawful, difficult, unethical, or all of the above?
But what if analysing that data could provide unique insights into critical areas of regulation, such as anti-money laundering? Should the ability to reduce criminal activity be traded off against privacy, and who should decide which is more important?
To put it differently, is it possible to gain valuable data insights in critical areas of regulatory activity while respecting sensitivity and the legitimate need for protection? This question underpins the emerging debate on synthetic data (Royal Society, 2023; Royal Society, 2022).
What is synthetic data?
Gartner defines synthetic data as a class of data that is artificially generated, as opposed to being collected via real-world observation. This synthetic data may be designed to mimic certain properties of real data or may be designed to simulate never-before-seen scenarios. It can also be used to make real data more representative by supplementing them with more diverse data.
Synthetic data sits within a larger bucket of Privacy Enhancing Technologies (PETs). The Information Commissioner’s Office (ICO), the UK’s Data Protection Authority, describes PETs as technologies that can help organisations share and use people’s data responsibly, transparently and securely. Importantly, this involves minimising the data used and encrypting or anonymising personal information.
Work on synthetic data at the UK Financial Conduct Authority (FCA)
The FCA has a synthetic data work programme which aims to explore how the technology can enable data sharing in financial services in a privacy-compliant manner. This programme has three core pillars:
- Industry engagement: Through our TechSprint, Synthetic Data Expert Group and Digital Sandbox initiatives, and the US-UK PETs challenge, we developed our understanding of how innovators practically use synthetic data to develop proofs-of-concept and products.
- Internal exploration: We are developing our own synthetic data generation capabilities through a series of pilots, focusing specifically on how synthetic data can improve our regulatory capabilities and resolve challenges in critical areas of regulation.
- Research: In March 2022, we published a Call for Input (CFI) to gather market views on synthetic data and explore challenges at the cutting edge of synthetic data research. On 9 February 2023, we published the Feedback Statement summarising the responses we received to the Call for Input.
Overall, synthetic data is an area of considerable interest, with significant research and proof-of-concept testing taking place in UK financial markets.
The challenges to accessing and sharing data in UK financial markets
The responses to the CFI highlight the current challenges associated with accessing and sharing quality data in UK financial markets. The challenges mentioned include:
- Real data not being accessible to collect at scale.
- Real data may have inherent imbalances (such as a lack of demographic diversity), raising issues around representativeness.
- The cost. Purchasing datasets can be expensive and impractical for a new market entrant.
- Real data may be inherently sensitive and is rightly privacy protected.
- For ‘edge cases’, the data either does not exist in sufficient quantities for training purposes or does not exist at all.
- Poor data management practices, a culture of operating in data silos and a lack of standardisation between datasets.
Synthetic data techniques provide promising avenues for overcoming or mitigating these challenges. However, this will vary by use case, and these techniques shouldn’t be seen as a panacea.
Our work at the FCA suggests that synthetic data is one promising way of accessing the insights inherent in the data without accessing the sensitive properties in the real data. To borrow language from the ICO’s draft guidance on PETs: synthetic data opens up unprecedented opportunities to harness the power of data through innovative and trustworthy AI.
The need for innovation in the detection of financial crime
When asked about the highest priority use case for synthetic data, 48% of respondents to the CFI indicated that financial crime detection, including fraud and anti-money laundering, is the most urgent use case. This is followed by the use of synthetic data for AI model development for 36% of respondents and, thirdly, data sharing both with third parties and cross-border, 18% of respondents.
The respondents who highlighted the importance of synthetic data for the detection of financial crime also identified a range of unique potential benefits:
- Enrich real datasets with known fraudulent typologies to improve fraud detection.
- Help developers to evaluate fraud algorithm performance.
- Assist firms in automating the process of user identity verification using AI trained on synthetic data, including Know-Your-Customer, or KYC checks.
For anti-money laundering in particular, respondents identified the benefit of synthetic data in assessing cross-border money laundering risks and testing the effectiveness of financial transaction monitoring systems. The findings of the members of the AI Public Private Forum support this view.
What these responses indicate is that synthetic data can make an essential contribution to much-needed innovation in the detection of financial crime.
Synthetic data and the broader debate on artificial intelligence in financial services
The FCA’s research suggests that synthetic data raises the following issues:
Access to data. Accessing and sharing data in financial services is a challenge, inhibiting the exploration of synthetic data and algorithmic models as potential regulatory tools. This can, for example, restrict competitive entry by smaller incumbents or create barriers to innovation.
Data quality. Poor quality data can potentially compromise any decision-making processes that rely upon those data (whether real or synthetic data). The ways in which data are sourced and aggregated can impact intended outcomes, which is a fundamental aspect of data integrity. This is true for all types of data analytics, not just synthetic data or algorithmic processing.
Data augmentation. Synthetic data can enrich data quality in various ways, like fixing structural deficiencies in real data, developing large-scale datasets where labelled data is scarce, or balancing skewed or biased data. However, how data is augmented raises profound questions about the reliability and provenance of that data, its purpose and techniques, what typologies it is based on and how it is integrated into the data set, including governance.
Governance. Data governance can be summarised as principles and practices that ensure quality and integrity throughout the data lifecycle. The ICO’s draft guidance on PETs, for example, provides a framework to think about data governance from a data protection perspective. This issue is also discussed in the joint Bank and England and FCA AI Discussion Paper and the AIPPF’s final report.
Bias risk. Synthetic data can replicate biases found in real data if they are not addressed before generation. At the same time, synthetic data can be designed to remove biases in existing datasets and retrospectively test and re-train algorithms that are proven to be prone to bias, suggesting that fairness considerations can be built into the data generation process.
Data fidelity. Synthetic data generation can lead to inaccuracies, especially when real data does not exist, and assumptions are made when generating the synthetic data.
Data utility. Validating synthetic data requires access to real data, which can be challenging if access restrictions exist or the firm purchases the synthetic data from an external source. In addition, there are no clear benchmarks on what comprises ‘good performance’ for synthetic data generation methods. Ultimately the appropriate metrics to assess utility will depend on the synthetic dataset’s purpose and the use case.
Ethics. Using synthetic data for decisions that impact consumers and markets in the real world is not uncontested. For example, if the synthetic dataset contains biases or is of poor quality, firms could make incorrect decisions resulting in consumer harm, discrimination or financial exclusion. There are also profound questions about the right to challenge systems and decisions based on synthetic data. The absence of transparency and explainability in how synthetic data is generated and used in real-world applications can create significant ethical challenges, potentially leading to a loss of public trust.
Data security and operational resilience. Many of the principles, expectations, and requirements for operational resilience may provide a useful basis for the management of certain risks posed by the use of advanced technologies, including AI. Financial services firms are required to meet applicable operational resilience requirements and expectations irrespective of whether a technology is developed in-house or by third parties.
These, and other areas, are discussed in the FCA’s and Bank of England’s AI Discussion Paper. The paper notes that safe and high-quality data and strong governance and accountability underpin responsible AI adoption in UK financial services. Similarly, the increasing volumes of data involved in the use of AI mean that data security and related issues such as data protection and privacy are ever more critical to ensuring safe and responsible AI (see also, for example, the European Data Protection Supervisor).
Responsible use of synthetic data at the service of consumers and markets
Setting higher standards and positive change that put consumers’ needs first are key commitments for the FCA. As part of that commitment, synthetic data can facilitate beneficial innovation and research in financial markets.
By enabling organisations to share and collaboratively analyse sensitive data in a privacy-preserving manner, synthetic data opens up new routes to data analytics where users can harness the power of data. Synthetic data is not a silver bullet for data protection compliance and needs to be processed in a manner that is lawful, fair and transparent. Provided this requirement is met, synthetic data can also make measurable improvements in anti-money laundering and other critical regulatory regimes and contribute to beneficial innovation in UK financial markets.
The FCA will continue to work with industry, academia, regulators, and other stakeholders to ensure that synthetic data is adopted responsibly and serves the interests of consumers and markets. To this end, we have established the Synthetic Data Expert Group (SDEG) as a practical framework for collaboration and exploration.