Three habits to cultivate when converting ethical AI principles into ethical AI practice
Here are three habits we found can be helpful to guide the process of going from ethical AI principles to ethical AI practice.
Over the past year, we have learned a great deal at Gradient Institute about the practical implementation of ethical AI principles. Our experience comes from working with industry and government agencies alike.
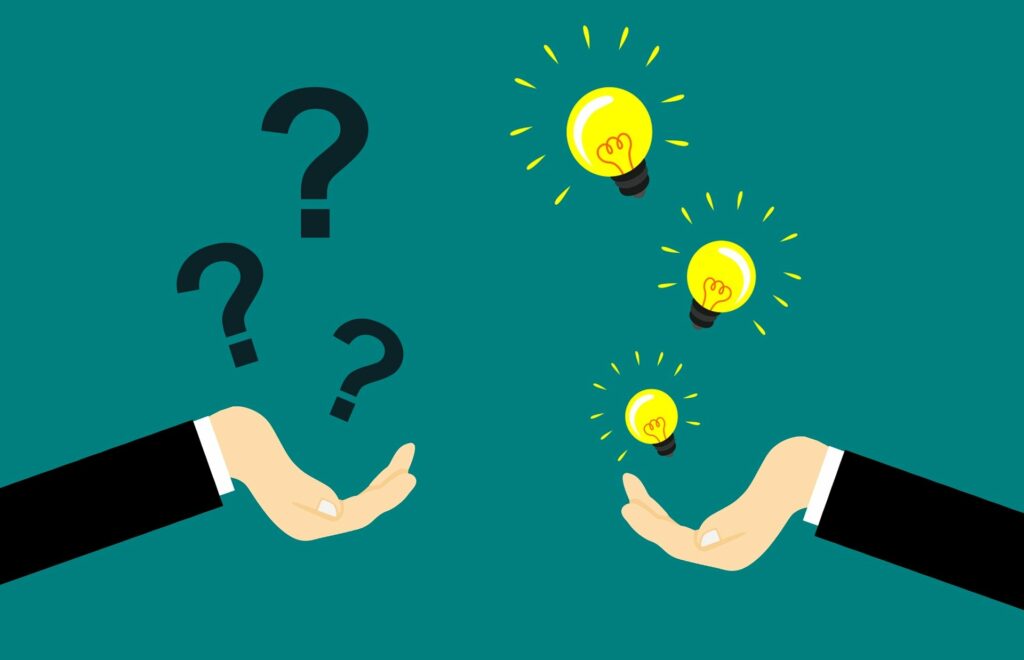
Image by mohamed Hassan from Pixabay
- Prioritise across and within principles to maximise impact. Ethical AI principles, including the OECD principles, focus on aspirational properties for AI. In practice, some principles will be more impactful than others upon implementation in any given circumstance. Not only that, but different ways of implementing the same principle can have dramatically different impacts. This means that when thinking about how to operationalise the principles in a particular context, it is important to develop the habit of prioritising both across principles as well as across ways of implementing principles with a view towards maximising impact.
For example, consider prioritising across ways of implementing the principle of fairness. In our work, we have observed that organisations trying to implement this principle sometimes focus too much on “algorithmically” balancing overall accuracy and fairness objectives, which means purposefully making the algorithm less accurate for privileged groups (thus reducing the disparities across groups). This is not the only way to reduce disparities – how about improving the accuracy for underprivileged groups? That can be done by developing a deeper understanding of what a population’s vulnerabilities are in relation to the decisions of a given AI system, or by improving the quality of the data collected, particularly for more vulnerable groups. These actions are harder than tweaking an algorithm to reduce its performance in a privileged cohort, but the right thing to do is rarely the easiest.
Such a deep understanding of different groups’ needs can be as or more important than the AI expertise itself. This includes having a broader understanding of the socio-technical context within which the AI system is integrated. More generally, this means that in order to establish the right priorities we must consult not only the AI experts but also the social scientists, domain experts and other experts who are often in a better position to assess the real impact that interventions of an AI system will have on the wellbeing of different groups within the affected population.
- Make it specific, but no more than necessary. There is an urgent need to translate AI Ethics principles into practical artefacts such as practice guidelines, codes, standards, policies, regulation and legislation. Yet, what is achieved by applying a principle often comes with a loss because the resulting artefact is not easily transferable to other cases. This suggests that a practical artefact should only be specific enough to allow its consumer to implement the principle with sufficient accuracy. Practicing this habit makes artefacts more transferable and lowers costs for applying them to other domains.
- Instead of making lists, ask questions. We noted stakeholders prefer receiving a list of good questions to ask when procuring, operating or developing an AI system to receiving specific instructions, or a “to do” list. On a human level this makes sense, since a question is an invitation for reflection and engages stakeholders in a more active and positive way than a set of instructions does.
Questions such as the ones below compel the designers, users and operators of AI systems to think carefully about a situation and come up with solutions that perhaps wouldn’t emerge through the mechanical execution of a to-do list.
- “What is the worst thing that can happen to the most adversely affected person as a result of deploying this AI system?”
- “Are the people most likely to be negatively affected by this system already disadvantaged compared to others?”
- “What is the worst thing that an AI system can do that still satisfies the goal the designers/operators have set for it?
To be clear, this is not an argument against checklists as a whole. There will always be circumstances where prescriptive checklists are the most appropriate type of artefact, such as security and risk management scenarios where they can be very powerful tools. Rather, it is an argument against the inappropriate use of overly specific approaches to solutions in situations where stakeholders would yield better results by exercising valuable discretion through their own thinking.
About Gradient Institute
Gradient Institute is an independent, not for profit technical research institute devoted to creating and disseminating ethical AI systems. Announced in December 2018, the Institute conducts research, consulting and training, and provides policy advice on ethical AI. To realize our vision of a world where all systems behave ethically, we collaborate with industry, government, the not-for-profit sector and academia. As a registered charity and an approved research institute, it has a deductible gift recipient status.
In a recent whitepaper, Gradient Institute describes the high-priority challenges that we want to address to make ethical AI the rule, not the exception. For details on that, please follow the link to the whitepaper.