Everyone must understand the environmental costs of AI
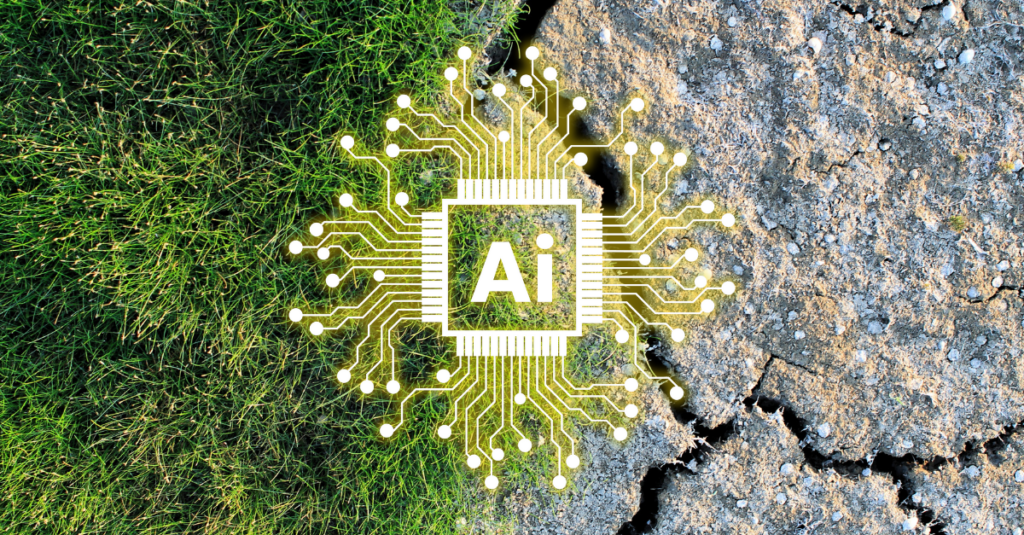
Artificial Intelligence (AI) has a profound effect on societies around the globe. Its application improves the lives of many, but it can also increase inequities. To safeguard against AI’s negative impacts, all actors must develop and deploy AI responsibly.
The responsible use of AI typically focuses on ensuring fairness, transparency, accountability, and safety but rarely on environmental responsibility. This is a significant oversight as there are many critical ecological implications of AI which cannot simply be ignored, including those associated with storing and processing the vast volumes of data many AI models use to train and operate, to perform task(s) and generate output(s) such as building a representation of the global environment. As an illustration, Luccioni and colleagues reveal in their analysis of energy costs between different models that classification tasks require between 0.002 and 0.007 kWh per 1,000 inferences. In comparison, generative tasks require around ten times more energy for the same number of inferences (around 0.05 kWh).
The escalating use of AI increases environmental costs
Much of the recent progress in AI has been achieved through significant increases in data consumption and computing infrastructures.
Data is central to training AI models in creating, selecting and calibrating models and algorithms. It is also crucial to inferences – the process a trained model uses to draw conclusions from brand-new data. The growth of AI is a significant driver for the rapid expansion of global data creation volume. Indeed, it is predicted that by 2025, the world will generate 181 zettabytes of new data, and that will increase to over 2,000 zettabytes by 2035.
The computing infrastructure for AI systems transitioned from general-purpose processors to specialised processors such as Graphics Processing Units (GPUs), Tensor Processing Units (TPUs), and Neural Processing Units (NPUs). However, demand is not slowing. Indeed, training general-purpose AI models now requires around ten billion times more compute than the state-of-the-art model training in 2010.
This reliance on massive scaling brings sustainability issues to the fore. This is likely to get worse as much of the hoped-for progress in AI relies on these inexorable trends continuing. To illustrate, by 2026, global computation for AI is projected to require a similar amount of annual electricity to countries like Austria and Finland.
Given this context, AI’s responsible and efficient utilisation is critical to managing private and public carbon emissions. Only by embedding considerations of digital decarbonisation into AI lifecycle management can we sustainably leverage AI technologies and, in turn, reduce environmental harm into the future.
AI has direct and indirect environmental impacts that are challenging to quantify
Any efforts to manage the environmental impact of AI must account for direct and indirect effects. Direct impacts stem from the lifecycle of AI compute resources and include the production, transport, operation, and end-of-life stages of an AI system. They are resource consumption-oriented and most often negatively impact water supplies, energy and associated greenhouse gas (GHG) emissions, and other raw materials. Indirect impacts result from AI applications and carry more positive impacts, such as smart grid and precision agriculture technologies. They also have some negative impacts, such as unsustainable changes in consumption.
More work is needed to quantify the sustainability of AI systems, especially regarding indirect impacts, which are much harder to quantify than direct ones. The area of cloud computing is probably the most advanced in this regard, with many major providers reporting on measures such as carbon intensity, hardware emissions, cloud usage over time and emissions by service and data centre region. Many cloud providers are also signatories of the Climate Neutral Data Centre Pact, a self-regulatory initiative with the shared commitment to make data centres in Europe climate-neutral by 2030.
However, there still needs to be more transparency across the industry as a whole, and the measures adopted to date do not differentiate between AI-specific and more general usage. This measurability issue is further complicated by the lack of an agreed-upon standard on accounting for the energy consumption of AI-related compute, including storage and network energy costs. Consequently, we need to find a way to determine the current environmental impact of AI systems.
Responsible data management is crucial to AI sustainability
Responsible data management practices can play a pivotal role in minimising unnecessary data storage, which would reduce the environmental impact of AI. Extensive datasets for training AI systems and inefficient storage practices can harm the environment through heightened energy consumption. One particularly harmful practice is synthetic data. Typically generated by computer simulations, synthetic data helps to overcome limited data availability for training. However, it threatens the environment because it accelerates global data creation. Beyond environmental concerns, synthetic data may amplify biases and lead to undesirable behaviours in AI models.
We can significantly decrease AI’s environmental footprint by adopting management strategies prioritising data minimisation, efficient storage, and responsible data disposal. Recent research illustrates this point well: up to 80% of organisations’ on-premise primary business data might be dark data or redundant, obsolete, and trivial data, equating to thousands of terabytes.
Part of this endeavour is adequately characterising the environmental impact of training AI models. It is rarely discussed, yet experts say “training remains orders of magnitude more energy- and carbon-intensive than inference.” Luccioni and colleagues provide an excellent, more encompassing analysis focused on different energy requirements for AI models. They compare the ongoing inference cost of ‘task-specific’ AI models, i.e. fine-tuned models that carry out a single task, and ‘general-purpose’ models trained for multiple tasks. In comparing “the most efficient” of task-specific models versus general-purpose models for extractive question answering, they observed that the former emits 0.3g of 𝐶𝑂2𝑒 compared with the latter models that emit 10g for the same volume of inferences. This highlights the importance of using the correct AI model for the task at hand to avoid a huge disparity in the negative impact on the environment.
The Trade-offs of AI development
The environmentally responsible use of AI requires a broad consideration of design choices and trade-offs. For instance, when considering different AI models, an essential step is critically assessing the necessary level of generality. While adopting the largest AI models may be tempting, evaluating whether such models genuinely enhance performance or if simpler, less resource-intensive models might suffice is crucial.
Moreover, if adopting general-purpose models, consideration should be given to how such models are, or have been, trained. For instance, open-source datasets help limit the acceleration of new data creation, thereby reducing the amount of GHG emissions associated with training. Reducing the prevalence of ‘dark data’ generated from training sets is another key consideration to avoid “the proliferation of unnecessary, redundant or overlapping data infrastructure”.
There are opportunities to integrate environmental efficiencies into all levels of training. For instance, the OECD Digital Economy Outlook 2024 highlights how individual users can help co-create AI models by training them using data on their own devices, then transferring the data to a central server to be combined into an improved model and then redistributed to the user community. In turn, this maximises existing training data rather than being dependent on creating new data, generating environmental efficiencies.
Raising awareness is a first step toward more sustainable AI
Responsible AI means balancing optimised performance with principled concerns like transparency, accountability and environmental impact. As AI’s performance improves via scalability, its growing energy consumption is unsustainable for several reasons: (i) finite compute and data resources are bottlenecking; (ii) energy consumption associated with general-purpose AI models is growing faster than the capacity of renewable energy sources and (iii) data-related CO2 emissions are growing, making AI models a significant part of global emissions.
AI development and operation sustainability must become a priority to resolve these challenges. By aligning AI growth with the broader goals of digital decarbonisation, we can ensure that AI’s integration across society is sustainable and responsible. This will require a collaborative effort between policymakers, researchers, and industry leaders to establish and enforce sustainability-focused guidelines. Raising awareness among AI practitioners about the environmental consequences of their work is the first step to cultivating a sustainability-minded culture within the AI community and driving meaningful progress.