The AI data scraping challenge: How can we proceed responsibly?
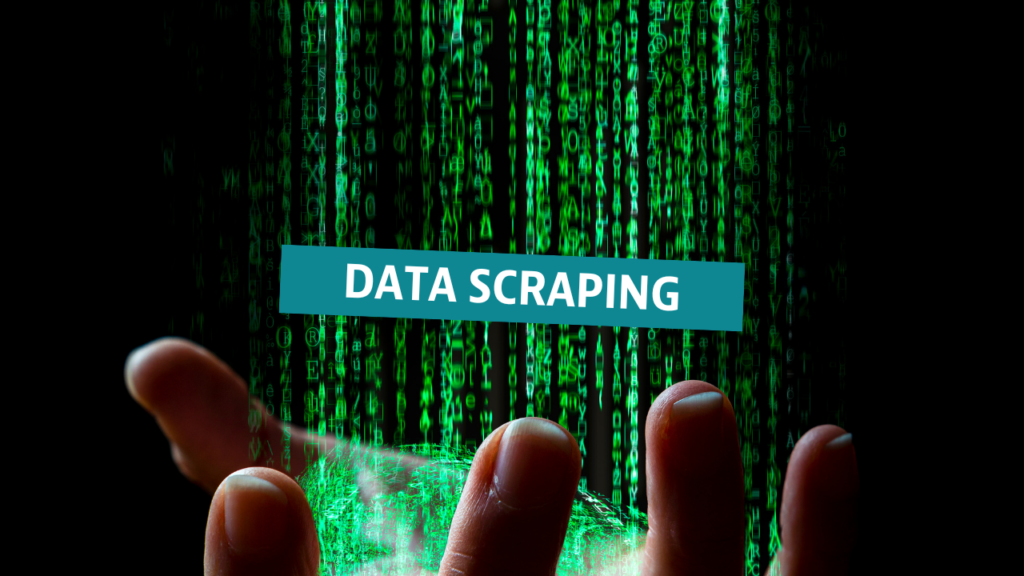
Society faces an urgent and complex artificial intelligence (AI) data scraping challenge. Left unsolved, it could threaten responsible AI innovation. Data scraping refers to using web crawlers or other means to obtain data from third-party websites or social media properties. Today’s large language models (LLMs) depend on vast amounts of scraped data for training and potentially other purposes. Scraped data can include facts, creative content, computer code, personal information, brands, and just about anything else. At least some LLM operators directly scrape data from third-party sites. Common Crawl, LAION, and other sites make scraped data readily accessible. Meanwhile, Bright Data and others offer scraped data for a fee.
In addition to fueling commercial LLMs, scraped data can provide researchers with much-needed data to advance social good. For instance, Environmental Journal explains how scraped data enhances sustainability analysis. Nature reports that scraped data improves research about opioid-related deaths. Training data in different languages can help make AI more accessible for users in Africa and other underserved regions. Access to training data can even advance the OECD AI Principles by improving safety and reducing bias and other harms, particularly when such data is suitable for the AI system’s intended purpose.
Data scraping controversies
Despite these benefits, data scraping has ignited many controversies. This trend will likely continue, particularly with the rise of OpenAI’s Sora and other text-to-video LLMs. Many controversies centre on LLM operators who do not seek affirmative consent or provide compensation for scraped data despite the fact that it may include copyrighted materials, trademarks, or personal information. And some scraped data has reportedly been stripped of its copyright management information (CMI). Compounding concerns, LLM operators often benefit financially from LLM models trained on scraped data.
Outputs resembling scraped data
The challenges escalate further when LLM outputs are similar or identical to scraped training data. For example, the New York Times alleged that ChatGPT unlawfully generates outputs that incorporate its copyrighted materials. LLM outputs have also reportedly replicated third-party brands. In some cases, LLM outputs mimic the style of artists or the likeness of people whose works, images, or voices were used to train LLMs. Sometimes, LLM outputs are used to deceive individuals, such as through misinformation or disinformation, or to falsely portray people, including Taylor Swift, in sexually explicit or other undesired ways. Collectively, these controversies can raise legal claims related to intellectual property, unjust enrichment, unfair competition, consumer protection, publicity rights, breach of contract, and more.
Personal data and jobs
Data scraping concerns also extend to other realms. For instance, scraping personal information can violate privacy laws and imperil highly sensitive personal information. LLM outputs may also reveal personal information or produce new personal data about individuals. LLMs can also hallucinate, adding to the legal concerns. These types of activities do not align with the 1980 OECD Privacy Guidelines or the OECD AI Principles. To help address these concerns, the OECD formed a new Expert Group on AI, Data, and Privacy, building more bridges between AI and privacy.
Relatedly, twelve Data Protection Authorities recently issued a joint statement (DPA Joint Statement) highlighting cybersecurity risks from data scraping. And last year’s Screen Actors Guild and Writers Guild strikes underscore how data scraping and LLMs can harm workers.
International harmonisation challenges
Complicating matters, LLM and data scraping activities often transcend borders. However, the many laws potentially governing these practices typically vary among jurisdictions, making international harmonisation more challenging.
Growing disputes
Data scraping has a long history of litigation and government enforcement, including protracted cases involving LinkedIn and Clearview AI. Unsurprisingly, with LLMs’ meteoric rise, many new disputes have emerged. For instance, the United States, Italy, France, Canada, and other countries have launched OpenAI investigations, focusing principally on privacy and consumer protection issues. The European Data Protection Board now has a ChatGPT task force. Other data scraping litigation, such as the New York Times case against OpenAI and Microsoft mentioned above, concentrate on intellectual property, breach of contract, and tort claims.
The lawsuits collectively involve many LLM actors, including OpenAI, Microsoft, Google, Meta, Anthropic, Stability.ai, Midjourney, DeviantArt, Runway AI, Bloomberg, and the non-profit Eleuther.ai that allegedly provided unlawful access to copyrighted materials. While litigation may ultimately lead to desired outcomes, it can be expensive, lengthy, and create uncertainty. Many aggrieved parties may also lack the resources to pursue litigation.
Policymakers’ response
Policymakers have responded to the AI data scraping challenge by trying to chart a responsible path forward at both multilateral and jurisdiction-specific levels. At the multilateral level, the G7 Hiroshima AI Process supports this endeavour with guiding principles and a voluntary code of conduct that underscore the need for “appropriate data input measures and protections for personal data and intellectual property.” The OECD also has its Guidelines for Multinational Enterprises on Responsible Business Conduct.
These initiatives provide important avenues for international harmonisation. They also open doors to integrate standard contract terms and technical tools, discussed below, into international codes of conduct, which could increase their impact.
Codes of conduct also may provide opportunities to address the type of data that can be scraped and how scraped data should be maintained and used, including for initial LLM training and possibly fine-tuning. Codes of conduct could also address both commercial and non-commercial applications, privacy-enhancing technologies, prompt practices, transparency, and output displays.
Scraping and the EU AI Act
The landmark EU AI Act has data scraping and intellectual property provisions that will come into effect, adding to existing requirements in the General Data Protection Regulation, Text and Data Mining regimes, the Data Act, and the Data Governance Act. The AI Act will ban AI systems that contribute to facial recognition databases through untargeted scraping of facial images from the internet or CCTV footage. It will also require general-purpose AI systems to comply with EU copyright laws and transparency requirements, including sharing information about training data.
Scraping and US policy initiatives
The US has several data scraping policy initiatives underway. For instance, the US Copyright Office requested public comment on AI and copyright, including AI training data and licensing approaches. President Biden’s recent AI Executive Order directs the Copyright Office to issue recommendations on protecting AI-generated works and using copyrighted materials for AI training. It also calls for greater privacy protections. The Federal Trade Commission (FTC) addressed AI training data in its recent report on General Artificial Intelligence and the Creative Economy. The FTC has also expressed consumer protection and competition law concerns and required the disgorgement of certain AI models trained on data without proper consents. Other US AI policy developments are occurring at the federal, state, and local levels.
Other jurisdictions
Other policymakers are focusing on data scraping, too. For instance, the UK convened a working group to develop a code of conduct for copyright and AI, aiming to increase data mining licenses. While this working group failed to reach an agreement, the UK government continues seeking solutions. Meanwhile, the UK Information Commissioner’s Office is examining data scraping’s privacy implications.
China’s comprehensive general services AI regulations require appropriate sourcing and labelling of training data and compliance with intellectual property and privacy laws. Japan, Israel, and Singapore have more permissive data scraping approaches, although Japan is considering adding more protections. Singapore wants to promote more efficient data sharing through new Collective Management Organizations and licensing arrangements. Singapore also published advisory guidelines on using personal data in AI recommendation and decision systems.
Leveraging contracts
As noted above, several policymakers, including in the US, UK, and Singapore, have highlighted the potential for contracts to help chart a responsible path for data scraping. The GPAI IP Advisory Committee’s responsible AI data and model sharing project supports this too, particularly when standard contract terms are complemented with codes of conduct, technical tools, and education. Given the many data-sharing use cases spanning research and commercial applications, it may be optimal to have various standard contract forms tailored for different situations.
Given the success of Standard Contract Clauses (SCCs) in other contexts, this approach seems promising. SCCs have helped manage cross-border data flows among countries with different privacy laws. Standard open-source and Creative Commons licenses have also helped unlock innovation and opportunities for small and medium-sized enterprises.
Many companies are also turning to contracts to help navigate AI training data rights. For instance, OpenAI announced deals with AP News and Shutterstock, and Google recently entered an agreement with Reddit. The New York Times, X, Zoom, Instacart, and others are addressing data scraping in their terms of service. However, the FTC has warned against unfair and deceptive changes to privacy policies and terms of service. Standard contract terms could help to address such concerns, too.
Finally, standard contract terms can be drafted with policy objectives in mind, helping to translate policies into practice. Policymakers may also choose to reference such standard contract terms in relevant codes of conduct to encourage their use.
Tools and education
As the DPA Joint Statement highlights, technical tools and education can also help address the AI data scraping challenge. This approach seems promising, as the New York Times, the Guardian, Disney, and other sites are already implementing technical measures to prevent data scraping. A new technical tool, Glaze, reportedly can prevent LLMs from imitating an artist’s style. Efforts are underway to develop a “Do Not Train” credential. Promising technical tools can be referenced in codes of conduct or standard contract terms to encourage their use and potentially provide remedies if such tools are circumvented or violated.
Many initiatives already exist to develop standards and tools to support responsible AI, including ongoing work on the US NIST AI Risk Management Framework and implementation standards for the EU AI Act. These important endeavours and similar efforts could provide platforms for advancing data scraping guardrails.
Relatedly, efforts should continue to develop machine-readable contracting mechanisms for data scraping, such as those under consideration with the W3C Text and Data Mining Reservation Protocol. This could make contract terms more understandable and thus facilitate compliance.
Education also plays a critical role, in part by facilitating and encouraging responsible behaviour. Data holders should be empowered with information on how to protect their rights. Equally important, LLM operators and data scrapers must understand the laws and policies pertaining to AI training data and their activities.
A holistic solution
Responsible AI is a global and multi-disciplinary field requiring input from diverse stakeholders and experts to unlock AI’s promise and mitigate its risks. The broad community must come together to address the AI data scraping challenge. Collectively, it should consider a combination of approaches, including laws, codes of conduct, standard contract terms, technical tools, and education. The whole may be greater than the sum of the parts.
Many thanks to my research associates Lea Daun, Nicole De Brigard, and Nargiz Kazimova for their terrific research support.