Advancing accountability in AI
This report provides an overview of how risk management frameworks and the AI system lifecycle can promote trustworthy AI.
GET THE FULL REPORT “Advancing Accountability in AI: Governing and managing risks throughout the lifecycle for trustworthy AI”
Trustworthy AI calls for each AI actor to be accountable for the proper functioning of their AI systems in accordance with their role, context, and ability to act. To achieve this, actors need to govern and manage risks throughout their AI systems’ lifecycle – from planning and design, to data collection and processing, to model building and validation, to deployment, operation and monitoring. This report:
- Presents research and findings on accountability and risk in AI systems by providing an overview of how risk management frameworks and the AI system lifecycle can promote trustworthy AI;
- Explores processes and technical attributes that can facilitate the implementation of values-based principles for trustworthy AI; and
- Identifies tools and mechanisms to define, assess, treat, and govern risks at each stage of the AI system lifecycle.
To do so, this report leverages OECD frameworks such as the OECD AI Principles, the AI system lifecycle, the OECD Framework for Classifying AI Systems, and the OECD Due Diligence Guidance for Responsible Business Conduct.
Beyond the OECD, it uses recognised risk management and due-diligence frameworks like the ISO 31000 Risk Management Framework and the US National Institute of Standards and Technology’s AI Risk Management Framework.
High-level AI risk management interoperability framework: Governing and managing risks throughout the lifecycle for trustworthy AI
Risk management approaches applied throughout the AI system lifecycle can identify, assess, prioritise, and resolve situations that could adversely affect a system’s behaviour and outcomes. Four steps to manage AI risks while ensuring respect for human rights and democratic values can be identified based on NIST’s AI risk management framework, the ISO 31000 risk management framework, the OECD Due Diligence Guidance and others:
- Define scope, context, and criteria, including the relevant AI principles, stakeholders, and actors for each phase of the AI system lifecycle and the lifecycle itself.
- Assess the risks to trustworthy AI by identifying and analysing issues at individual, aggregate, and societal levels and evaluating the likelihood and level of harm (e.g. small risks can add up to a larger risk).
- Treat risks to cease, prevent, or mitigate adverse impacts commensurate with the likelihood and scope of each.
- Govern the risk management process by embedding and cultivating a culture of risk management in organisations; monitoring and reviewing the process in an ongoing manner; and documenting, communicating and consulting on the process and its outcomes.
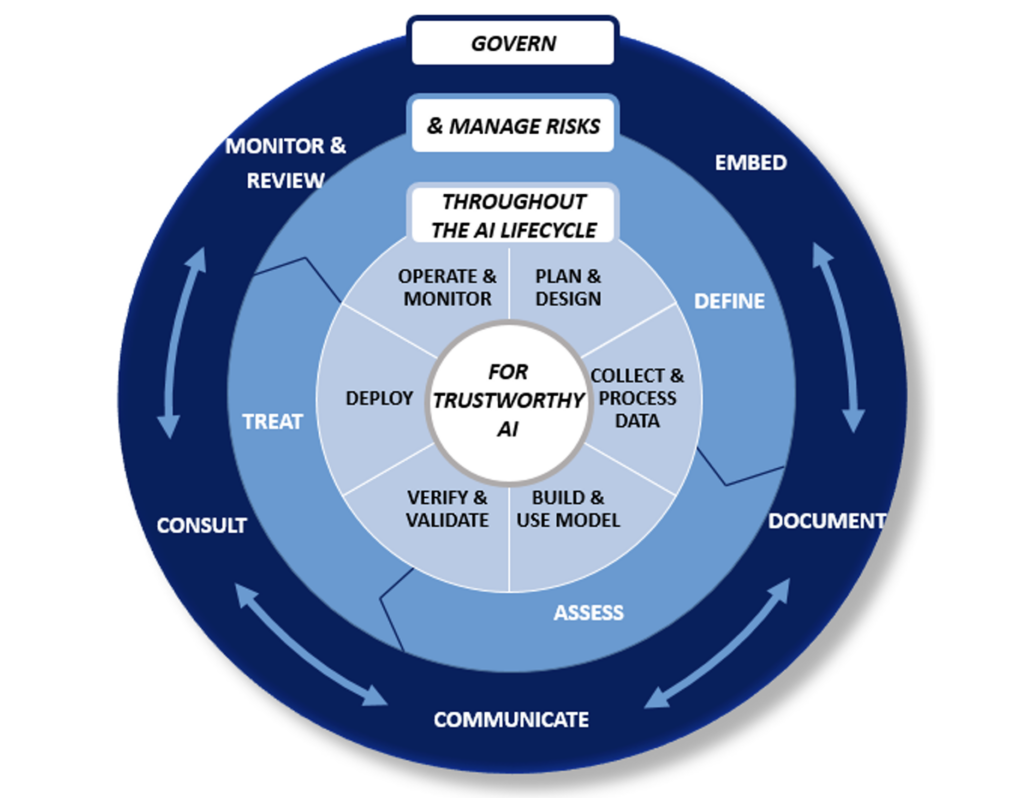
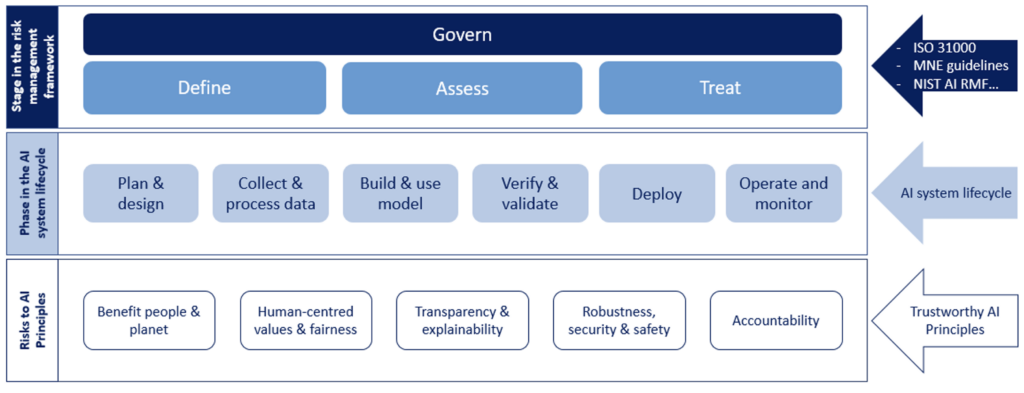
Providing accountability for trustworthy AI requires that actors leverage processes, indicators, standards, certification schemes, auditing, and other mechanisms to follow these steps at each phase of the AI system lifecycle. This should be an iterative process where the findings and outputs of one risk management stage feed into the others.
This publication contributes to the OECD’s Artificial Intelligence in Work, Innovation, Productivity and Skills (AI-WIPS) programme, which provides policymakers with new evidence and analysis to keep abreast of the fast-evolving changes in AI capabilities and diffusion and their implications for the world of work. For more information, please visit www.oecd.ai/wips. AI-WIPS is supported by the German Federal Ministry of Labour and Social Affairs (BMAS) and will complement the work of the German AI Observatory in the Ministry’s Policy Lab Digital, Work & Society. For more information, visit https://oecd.ai/work-innovation-productivity-skills and https://denkfabrik-bmas.de/.