The power of persuading: Can and should we regulate AI algorithms?
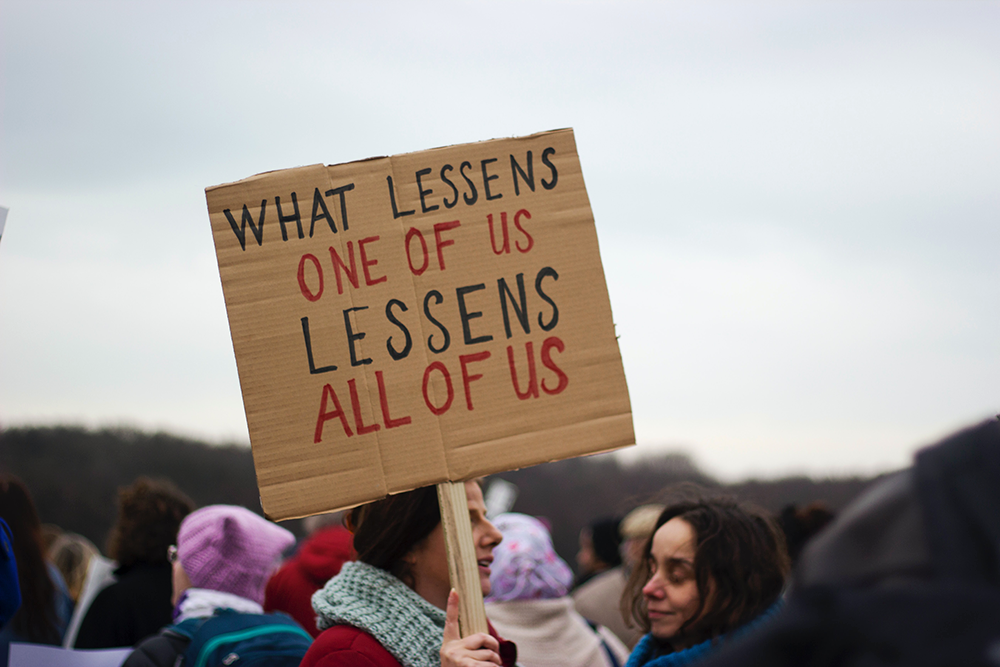
If you’re familiar with journalism, you may have heard the expression “man-bites-dog“, where the information that gets the most attention is the most surprising or unusual but not necessarily the most important. As online media struggle to gain recognition, traffic and ultimately revenues, they often turn to the most sensational and hyperbolic content. It goes without saying that many attention-seeking individuals and politicians also embrace this technique, on Twitter and elsewhere.
Twitter itself and other social media platforms tend to encourage this kind of behaviour. The most visible content on these platforms speaks more to emotional triggers than to objective thinking. In the Netflix-sponsored documentary, The Social Dilemma, Tristan Harris of the Center for Humane Technology presents the situation very well and lays out sound arguments for why there is a desperate need for positive change. He argues that maximizing engagement should not be measured through time spent online or the number of interactions. Instead, it should be an appraisal of the quality of the interactions or time well spent online.
Focusing thoughts on the quality of interactions is all well and good, but how do we establish an objective measure for that? With platforms being financed by advertising, their interests tend to favour maximizing the size and depth of their networks and the number of reactions. This pressures the platforms to design content features that incite users to react quickly, leaving relevant evidence and facts that would foster enlightened decisions out of the picture.
The power of persuading
By now everyone knows that the echo chambers created by recommendation algorithms have had a significant negative impact on politics in countries around the world, leading to calls for regulation. But in fact, they can have an effect on all societal debates. In the context of the global Black Lives Matter movement, while HBO max decided at first to remove the classic film Gone with the Wind, where slaves appear to enjoy their fate, others like Netflix, kept it. In moments of societal tensions, it is easy to see the political and social influences accrued to algorithms that target certain viewers with potentially divisive movies. Other viewers may be targeted with documentaries such asSpeak Up, where Director Amandine Gay films black women discussing the regular discrimination they suffer in France. The implications of such choices made by Netflix or other online media platforms relate directly to the OECD’S Second Values-Based Principle on AI that encourages human-centred values and fairness in the development and use of AI systems.
An argument for regulating algorithms
In addition to its own AI principles, the European Commission has put forward two legislative initiatives: the Digital Services Act (DSA) and the Digital Markets Act (DMA). Together, they aim to ensure that recommendation algorithms are safe and transparent while promoting fair competition and fostering innovation. A key principle behind both the DSA and DMA is to avoid reinforcing “gatekeepers” oversight, i.e., to keep an eye on the very large online platforms that play an entrenched and systemic role in linking individual users to businesses.
Armed with these new legislative tools, national regulatory authorities will now have to decide how to achieve their policy goals and ensure that the “gatekeepers” act for the common good, echoing recommendations laid out in the first OECD AI Principle.
Going back to the Social Dilemma, Tristan Harris suggests that an independent agency could analyse algorithms ex ante via a social impact assessment criteria. Hannah Fry from the University College of London explains in her well-received book how such an agency would then authorize and license algorithms, when appropriate, much in the way that the U.S. Food and Drug Administration with new medicines.
The question remains as to just how effective an ex ante assessment of algorithms would be. There would necessarily be blind spots and systematic errors owing to the unpredictability and shifting nature of human reactions in uncontrolled situations.
Algorithms and externality
Indeed, problems usually stem from the unfactored factors that are not taken into account. But it would be misguided to think that social platforms are indifferent to the consequences of their tools. Nonetheless, the algorithms that they design are conceived for specific purposes and that they generally work reasonably well in the short term. There is little consideration for the fact that an algorithm is a partial recipe that only focuses on a fraction of the possible ingredients.
Take “likes” on social networks for example. When it comes to generating engagement on social networks, the notions of truth and quality are absent from current algorithms. With few exceptions, misinformation goes unchecked.
This is how the algorithm bulldozes through to achieve its short-term goal, but its medium-term effects – polarization of information and communities, a lack of contradictory information and prioritization – fall outside of the scope of its purpose. In economics this is called externality, a notion whereby companies internalize some benefits and externalize the negative consequences such as pollution in an industrial context, leaving society as a whole to deal with the fallout.
The complexity of predicting the effects of algorithmic stimuli
In social contexts, stimuli can modify not only the individual they act upon but also the environment in which they operate. Assume that you analyse specific behaviours of humans and model them via an algorithm: when you try to use this algorithm to influence people, they find themselves in a new setting since someone is trying to modify their habitual behaviour. This in turn generates new reactions that can potentially make the algorithm useless or even counterproductive.
These ideas constitute the basis for the famous Lucas Critique (Robert E. Lucas is the 1995 Economics Nobel Prize recipient). Here is an example. Certain companies introduce price discrimination based on browsing history as recorded by cookies. Some internet users know this and often change their behaviour in order to fool the cookies responsible for the discrimination and obtain better deals. One of the most commonplace examples of this is to manipulate ticket prices offered by airline companies.
Following this example, it is easy to see why any authority’s ex ante control of the tools that artificial intelligence provide would be illusory. Particularly because their medium-term consequences are almost unpredictable given the number of factors involved.
An algorithmic authority modelled on central banks to ensure long term sustainability
So far, we have linked the question of algorithm regulation to regulating drugs and medicines. But we can also draw parallels to the authorities and mechanisms in place to control inflation.
Public authorities have long aimed to avoid the twin pitfalls of inflation that is too high (hyperinflation causing political instability in the 1920s) or too low (the deflation leading to impoverishment in the 1930s). Moderate inflation is optimal, but it is an unstable equilibrium, the result of the individual decisions of millions of individuals and companies, decisions that are themselves dependent upon the perceptions individuals have of their environments (past, present and future) and the decisions of others (competitors, suppliers, customers, etc.).
After thinking that governments in most industrialized economies could directly control prices (e.g., the price of bread in France until the 1980s) or policy tools, the agencies in charge of inflation control – central banks – have been given full possession of the relevant tools and made independent. This does not refer to the tools that directly control individual prices, but those impacting individual decision making (via interest rates) and supervising systemic market operators (banks and financial institutions).
Here, the role of the government is to set the objectives of monetary policy (low inflation and, in some countries such as the US, full employment). Central banks were made independent to convince the population of their sole pursuit of mandated medium-term objectives, away from short-term political considerations (that would come into play around election time).
Similarly, instead of imposing a priori administrative approval by an algorithm security agency, it may be more efficient to design an independent authority that directly supervises AI companies and some of their algorithms. With this approach, the Central Bank of Algorithms (CBA) could reintroduce a focus on the medium term and the evolution of society in accordance with objectives set by governments. In line with the DSA and DMA of the European Commission, the CBA could also monitor the degree of concentration of current Internet platforms, to avoid the emergence of gatekeepers that are “too big to fail” and endanger the whole system.
Its ability to act directly, its independence and its focus on explicit objectives would help foster a certain level of systemic trust, a key element of several of the OECD AI Principles. Injecting confidence through trust would make it possible to better anticipate the reactions of individuals to stimuli by improving reactivity and more easily resolving the issues posed by filter bubbles and misinformation.
What tools for systemic robustness?
What are the tools the CBA should possess to ensure that its ecosystem remains robust? This question can be analysed from the angle of recommendation algorithms that are over-optimized and target our past behaviours, and whose suggestions do not give enough weight to serendipity, or discovering new and unexpected interests.
Controlling the over-optimization of algorithms that present a political impact can protect the diversity of information and the democratic agora. This can be done, e.g., via adjusting how quickly algorithms learn, because speed has a direct impact on induced risk. Inducing a form of friction is often recommended to avoid the spread of fake news on social networks (see, e.g., the recent report by the Forum on Information and Democracy). Such simple mechanisms can improve the system’s plasticity and therefore its robustness, reinforcing the distinction between suggestion and influence. Even though the parallel between algorithm security policy and the mandates of central banks on monetary policy and financial supervision is not exact, many common elements that relate to intermediation and decentralized decision making by individuals based on their beliefs, expectations, and stimuli warrant further analysis. A complete risk analysis cannot be fully performed ex ante so policy makers must focus on deciding which tools they provide to the supervising authorities