These tools and metrics are designed to help AI actors develop and use trustworthy AI systems and applications that respect human rights and are fair, transparent, explainable, robust, secure and safe.
SHAP
About the tool
You can click on the links to see the associated tools
Objective(s):
Lifecycle stage(s):
Usage rights:
License:
Programming languages:
Github stars:
- 18389
Github forks:
- 2777
Use Cases
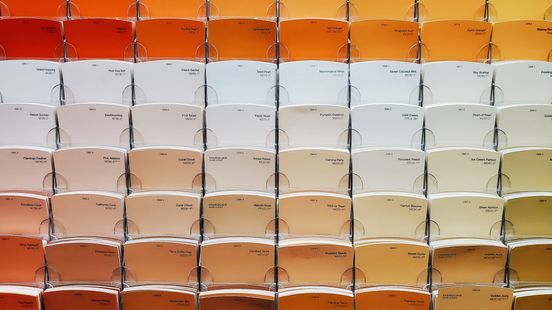
Nvidia: Explainable AI for credit risk management
Would you like to submit a use case for this tool?
If you have used this tool, we would love to know more about your experience.
Add use case