The hidden costs of AI: Unpacking its energy and water footprint
IEEE and the OECD are pleased to invite you to attend this event on the margin of the French AI Action Summit. Conversations will delve into the environmental costs of AI, specifically in relation to energy usage and water consumption.
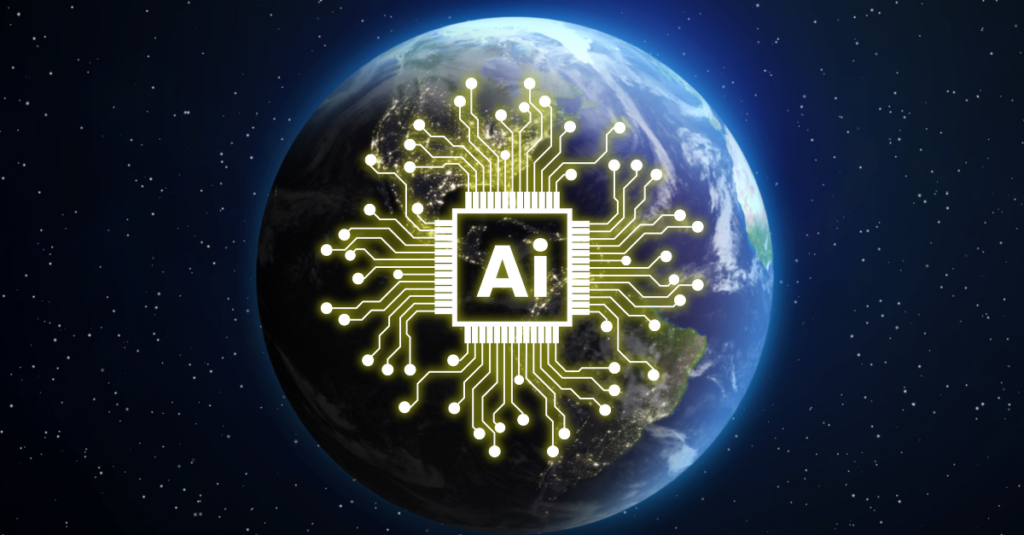

📅 This event was a side event to the French AI Action Summit and took place on 12 February 2025, at the OECD Conference Centre in Paris
As AI continues to reshape industries and our daily lives, its environmental impact is an increasingly important consideration. While much focus has been placed on AI’s energy consumption, its impact on other vital resources, such as water, is often overlooked.
Opening remarks 💠 Clara Neppel, Senior Director, European Business Operations, IEEE 💠 Karine Perset, Acting Head of Division, Artificial Intelligence and Emerging Digital Technologies, OECD |
Noman Bashir, MIT Climate and Sustainability Consortium (MSC) and MIT Computer Science and AI Lab (CSAIL) – Online |
The environmental cost of inference As AI models transition from experimental stages to widespread industrial deployment/production, the environmental cost of inference—where trained models are executed to provide outputs in real-time applications—has grown very significantly. With the increasing adoption of large language models (LLMs) and other AI models in everyday services like search engines, voice assistants, and recommendation systems, inference now represents a substantial and often overlooked portion of AI’s environmental footprint. Unlike training, which is a one-time expense, inference scales with user demand and is often embedded within complex AI pipelines, including filters, guardrails, and system prompts. This highlights the critical need to optimize not only individual models but also entire systems for energy efficiency and sustainability, ensuring that scaling deployments do not exacerbate environmental impacts. Addressing these challenges requires innovation in hardware, software design, and regulatory frameworks to balance functionality with sustainability. Guiding questions: • How do the scale and frequency of inference tasks contribute to its cumulative environmental impact, and what strategies can reduce this footprint? •What advancements in AI-specific hardware, such as energy-efficient processors, and overall compute trends like edge computing address inference energy consumption, and how can these innovations be accelerated? • How can model architectures (e.g., quantization, sparsity, or distillation) reduce inference costs without significantly compromising performance (e.g. quality and accuracy of predictions)? • How should this inform policymaking, regulation, and standards-setting, given current/future market incentives? • How can AI developers balance the choice between deploying large-scale models and smaller, more specialized models (SLMs) to meet use case requirements while minimizing energy consumption? • What role do model architectures, system-wide design, and use case-driven optimizations play in avoiding computational overkill and fostering frugality without sacrificing effectiveness? • How can industry collaboration foster best practices in inference optimization, and what role should governments and standard-setting bodies play in promoting environmentally friendly AI operations? Moderator: 💠 Nicolas Miailhe, Co-founder and CEO, PRISM Eval Speakers 💠 Théo Alves Da Costa, Head of AI for Sustainability, Ekimetrics 💠 Ana Paula Nishio de Sousa, Chief of Digital Transformation and AI Strategies, UNIDO 💠 Rosie Hood, Lead Data Scientist EMEA, LinkedIn 💠 Thierry Danse, Software Engineer, CO2 AI |
Impact of data centres on the electricity grid Data centres play an increasingly central role in powering the digital economy, especially with the rise of AI. However, the rapid expansion of these facilities raises important questions regarding their impact on electricity grids. Data centres consume vast amounts of energy, leading to concerns about grid reliability, sustainability, and the environmental footprint of their operations. As global data consumption grows, balancing energy efficiency, renewable energy integration, and grid stability has become a critical challenge for policymakers, energy providers, and the tech industry alike. Guiding questions: • What technological innovations in data centre design (e.g., cooling systems, energy-efficient hardware) could help mitigate their energy demands? • What role can renewable energy play in powering data centres? • How can the design and location of data centres influence their energy efficiency and impact on the grid? • In what ways can grid operators and energy providers collaborate with data centre operators to ensure grid stability while supporting the growth of digital infrastructure? • What policies or incentives could help accelerate the transition of data centres to cleaner, renewable energy sources? Moderator: 💠 Arti Garg, Chair of the P7100 Working Group, IEEE Speakers 💠 Brendan Reidenback, Policy Analyst, IEA 💠 Tom Jackson and 💠 Ian Hodgkinson, Professors at Loughborough Business School, Loughborough University – Online 💠 Alexis Normand, CEO & Co-founder, Greenly 💠 Maud Texier, Global Director of Clean Energy and Decarbonization Development, Climate Operations, Google |
AI Water Usage AI environmental impact extends beyond energy consumption to include natural resource use—specifically, water. AI technologies, particularly those relying on large-scale data processing and cloud computing, require significant amounts of water for cooling purposes in data centres. As the demand for AI-powered services grows, so does the pressure on water resources, particularly in regions already facing water scarcity. The challenge lies in balancing the benefits of AI with the responsible management of water resources, ensuring that the technology’s potential for innovation doesn’t come at the cost of sustainability. Guiding questions: • How significant is water consumption in AI-related infrastructure, especially in data centres, and how does it compare to other industries regarding resource use? • What are some innovative cooling technologies that can help reduce water usage in AI data centres, and how feasible are they to implement at scale? • How can the AI industry collaborate with local communities and governments to ensure that AI deployment doesn’t exacerbate regional water challenges? • What role can policymakers play in setting regulations or incentives that encourage water-efficient practices in the development and operation of AI infrastructure? • Are there examples where AI companies have successfully minimized their water usage, and what can others learn from these practices? Moderator: 💠 Irene Kitsara, European Standardization Initiative, Director, IEEE Speakers 💠 Shaolei Ren, Associate Professor of Electrical and Computer Engineering, University of California – Online 💠 Masheika Allgood, Founder, AllAI Consulting – Online 💠 Colin Herron, Senior Water Resources Management Specialist, Water Solutions for SDGs, Global Water Partnership – Online 💠 Jeremy Tamanini, Founder, Dual Citizen 💠 Simon Gosset, AI and Sustainability Director, Capgemini Invent France |