Accelerating science could be the most valuable use of AI
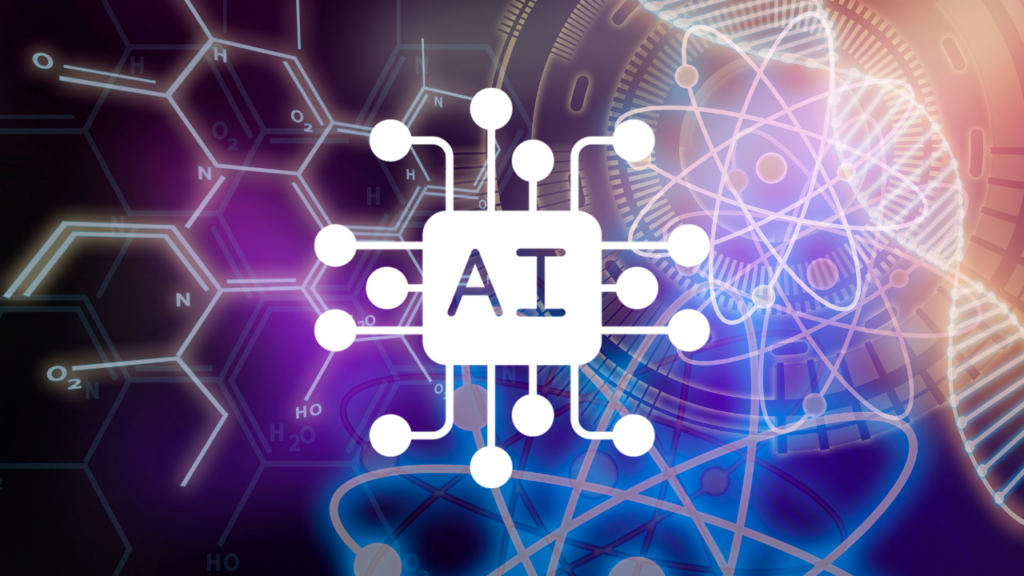
The OECD’s recent publication – Artificial Intelligence in Science: Challenges, Opportunities and the Future of Science – describes how AI is being used in science today, how it might be used in the near future, its impacts and the implications for public policy
Accelerating research productivity could be the most economically and socially valuable use for artificial intelligence (AI). In his recent testimony to the U.S. Senate Energy and Natural Resources Committee, Rick Stevens, from the US Argonne National Laboratory, stated: “Whoever leads the world in AI for science will lead the world in scientific discovery and will have a head start in the translation of discoveries to products that expand our economy and address modern needs, securing the innovation frontier”.
Why the productivity of science is critical
Consider three reasons why more and faster discovery of scientific knowledge matters greatly:
Living standards and growth: A direct relationship exists between innovation, which draws from research, and long-term economic productivity growth. Productivity growth drives increases in living standards. Raising productivity will be increasingly urgent because working-age people are becoming an ever-smaller share of the population. Theory also suggests that growth due to more productive research and development (R&D) is more lasting than that spurred by production automation.
Urgently needed scientific knowledge is lacking: Many fields of science are advancing rapidly. However, many old scientific questions endure, and a faster tempo of discovery is essential. For example, after decades of climate modelling, important uncertainties exist on such issues as tipping points (e.g. inversion of the flows of cold and hot oceanic waters) and when changes could become irreversible (e.g. melting of ice shelves). More than 21 million people in OECD countries suffer from Alzheimer’s disease or other dementias, and these numbers are set to skyrocket as populations age. While studies have identified several risk factors for Alzheimer’s disease – from age to high cholesterol –treatments are missing. There is also alarm that the world is entering a post-antibiotic era. Many antibiotics in use today were discovered in the 1950s, and the most recent class of antibiotic treatments was discovered in 1987.
Science itself may be becoming harder: Claims that science may get harder over time are not new, but attention to this possibility has been renewed by Bloom et al. (2020) and other studies. If science were to become harder and other things unchanged, governments would need to spend more to achieve the existing rates of growth of useful scientific outputs. Timeframes for achieving scientific progress could become longer. And for the equivalent of today’s investments in science, ever-fewer increments of new knowledge would be available to counter harms such as new contagions and novel crop diseases.
Enter AI
A welcome development is that AI and its subdisciplines are spreading to every field and stage of the scientific process. Papers examining how AI will affect science are frequent in leading journals such as Nature and Science. Scientific institutions – from the US National Academies to the United Kingdom’s Royal Society – are exploring AI’s implications for science. Even non-specialised journals, such as The Economist, ask how research might evolve when powered by AI.
The most widely publicised recent breakthrough using AI has been DeepMind’s development of a model – Alphafold 2 – to predict protein folding. Predicting the 3-dimensional shape of proteins from the sequence of their amino acids helps to determine their function. Much depends on solving this problem. Most drug discovery, for example, starts with finding the right protein to target a disease. DeepMind’s achievement has sparked a revolution in molecular biology.
AI’s uses in science go well beyond prediction. For instance, using a variety of approaches, AI is helping to explore otherwise overwhelming numbers of scientific papers. As an example, just the first twelve months of the COVID pandemic saw more than 100,000 scientific articles published on the coronavirus.
A technique known as Literature-Based Discovery (LBD) is being used to uncover links between disconnected areas of research. The whole field of drug repurposing – investigating existing drugs for new therapeutic purposes – was born from LBD. Another example of LBD comes from NASA, which needed to create lighter, more compact, and easy-to-fold-away solar panels for spacecraft. LBD found that the ancient art of origami could help to create the right folding structures.
AI is also revolutionising microscopy, for instance finding just the most important parts of an image of delicate biological structures within cells, avoiding exposing them to too much harmful light. In materials science, AI can reliably enhance cheaper, low-resolution electron microscopic images into otherwise more expensive high-resolution images.
AI in science creates efficiencies
AI can suggest the most efficient experiments for a given problem. It can make data acquisition more efficient by prioritising data gathering where data is uncertain. And AI can save money by identifying which parts of a data set reveal most about all the data, allowing researchers to use expensive compute time efficiently.
An exciting area of progress for science is in robots. For some years, robots have helped to automate routine laboratory processes. But AI-driven laboratory robots can now go beyond this mechanical task. They can execute cycles of testing, hypothesis generation and renewed testing. And they can do this within closed loops, with minimal human involvement (figure 1). Such systems can also automatically record experimental procedures – the exact steps taken when performing an experiment – which saves money and is important for reproducing research.
Figure 1. An autonomous laboratory robot at the University of Liverpool
This robot chemist developed at the University of Liverpool moves about the laboratory guided by Lidar and touch sensors. An algorithm lets the robot explore almost 100 million possible experiments, choosing which to do next based on previous test results. The robot can operate for days, stopping only to charge its batteries.
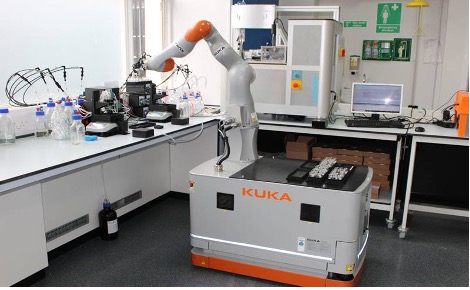
This robot chemist developed at the University of Liverpool moves about the laboratory guided by Lidar and touch sensors. An algorithm lets the robot explore almost 100 million possible experiments, choosing which to do next based on previous test results. The robot can operate for days, stopping only to charge its batteries.
The advent of ChatGPT and large language models
Within days of its release, ChatGPT spurred a flurry of commentaries in the scientific press asking how Large Language Models (LLMs) might affect research and if they would require new guidelines in scientific publication and research governance. Concerns sprang from the prospects of LLMs facilitating fraud, plagiarising, and confabulating in research papers, and the consequences of LLM being used in research review. The debates are ongoing, and their terms will shift as LLMs acquire new capabilities and scientists learn exactly how they work. While some concerns are legitimate, they must be weighed against LLMs’ benefits to science. For instance, large savings in scientists’ time could come from using increasingly capable LLM-driven research assistants. GPT4.5 has been shown to provide research reviews comparable in quality to human reviewers. And LLMs can help non-native speakers present scientific work better.
Policymakers and actors across research systems can accelerate the uptake of AI in science
Artificial Intelligence in Science: Challenges, Opportunities and the Future of Research (OECD (2023) describes a broad suite of actions open to policymakers to help deepen AI’s contribution to science and society. To consider just a few:
Governments can support multidisciplinary programmes that bring together computer and other scientists with engineers, statisticians, mathematicians and others to solve challenges using AI. Specialists in different fields of science who want to use AI in their research are often separated from the wider community of AI specialists. Programmes could be organised around global challenges and long-term impacts. Only about 6% of LBD research papers address any Sustainable Development Goals today. Ambitious multi-disciplinary challenges could inspire collaboration and coordination in science, drive agreement on standards and attract young scientists.
Policymakers and universities can rethink curricula. For example, students could be taught how to search for new hypotheses in existing scientific literature using already-proven AI-enabled techniques. The standard biomedical curriculum provides no such training. Education in science could raise awareness of what AI-enabled robot systems can do and how they are developing. Research software engineers who translate between research and software engineering need career paths. There is no career path for software developers in most universities today.
Policies can help improve access to computation. For academics aiming to use state-of-the-art AI, computing resources from commercial cloud providers are usually unrealistically expensive. Nationally funded laboratories using supercomputers could collaborate with industry and academia to support AI ecosystems for universities. Tutorial-accessible step-up guides could be developed so that students and practitioners can begin on personal computers or small-scale cloud resources but advance to larger cloud or institutional-scale resources and then on to national-scale systems.
Public R&D can target areas of research where breakthroughs could deepen AI’s uses in science and engineering. Funders could focus on projects that explore new techniques and methods separate from the dominant approaches based on large datasets and high-performance computing. For instance, funders could help develop tools to enhance collaborative human-AI teams and integrate them into mainstream science. Combining the collective intelligence of humans and AI is important, not least because science is now carried out by ever-larger teams and international consortia. Investment in this field of research has lagged other topics in AI.
Knowledge bases organise the world’s knowledge by drawing on information from many sources and mapping connections between different concepts. Governments could support an extensive programme to build knowledge bases essential to AI in science, a need that the private sector will not meet.
Industrial robotics has developed rapidly, but not always in ways that meet the needs of science. Collaborative research programmes and centres could help to bridge these needs by bringing together scientists and roboticists.
Interest in AI in science will only grow
The attention of policymakers and scientists and perhaps the public will be increasingly drawn to AI’s uses in science. There are several reasons why:
Some OECD governments are funding new programmes to harness AI for science. China for instance has announced plans for far-reaching public investments in measures to achieve prominence in AI for science.
Many policy, economic and socially important outcomes are also uncertain. For instance, some OECD countries with relatively small scientific bases indicate uncertainty on where they should best allocate resources and concerns they might be left behind; the consequences for science in the developing world are unclear; and concerns may grow that large tech companies, which are investing in AI R&D at much larger scale and more quickly than public bodies, will steer research agendas.
Indicative of the current state of flux and uncertainty, a survey of 1,600 researchers conducted by Nature found that ‘scientists are concerned, as well as excited, by the increasing use of artificial intelligence tools in research’. Indeed, there are many AI technologies, and they are evolving quickly. These changes, which are to some degree unforeseeable, could have far-reaching implications. There are still questions about how to approach some emerging challenges in research governance, such as how to manage the increased ability of AI to semi-autonomously design dangerous molecules.