Building trust in AI: A Practical approach to transparency
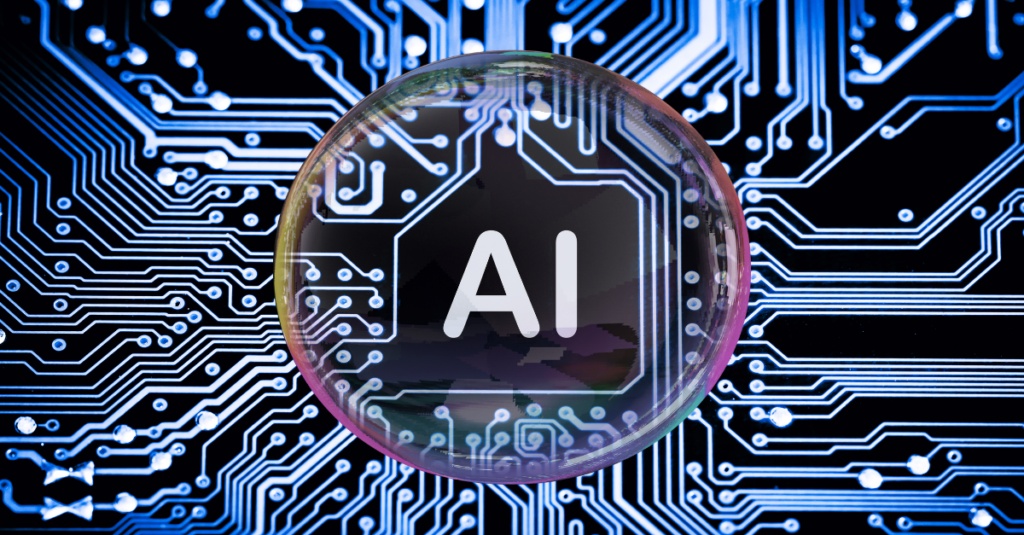
As AI systems become increasingly sophisticated and integrated into our daily lives, there’s a growing demand for clear insights into how these systems are built, tested, and safeguarded. While transparency is emerging as a key tenet across voluntary commitments and developing regulations, there are no widely recognised standards or best practices. Approaches like Anthropic’s Transparency Hub and standardised reporting through the OECD framework represent promising paths toward making complex AI development information accessible to diverse stakeholders while streamlining industry reporting requirements.
Anthropic’s Transparency Hub demonstrates one approach to meaningful transparency. It provides centralised information about model development processes, capability and safety evaluations, and platform detection and enforcement metrics. By structuring this information in accessible formats, users can better understand how AI systems are developed, tested and deployed.
Why transparency matters in AI development
The rapid evolution of AI capabilities necessitates transparency about how companies implement development and safety practices to reveal both responsible approaches and potential shortcomings. Transparency on model development, capability evaluations, and platform usage helps address these concerns by providing insight into the work happening behind the scenes. While transparency reporting is an established practice in the tech industry, it takes on heightened importance in the AI ecosystem, particularly where formal regulations are still developing.
Transparency in AI development and deployment offers several key benefits:
- It enables meaningful comparisons across industry
- It facilitates knowledge sharing and adoption of best practices
- It builds and maintains public trust by creating accountability of AI technologies
- It provides empirical examples of responsible practices that can inform technically feasible regulations
Historically, proactive transparency has informed tech regulation in meaningful ways. Early tech company transparency reports on government data requests eventually became industry standard practice. The voluntary implementation of privacy tools and controls ahead of regulation helped shape the practical frameworks later codified in laws like GDPR. These precedents underscore how today’s voluntary AI transparency efforts may similarly inform tomorrow’s regulatory frameworks, creating a need for thoughtful, standardised approaches to AI transparency reporting.
The challenge of meaningful transparency
Implementing substantive transparency in AI development involves navigating several challenges.
- 1. Making technical information accessible
The first challenge is determining what information is genuinely useful to different audiences (versus what might create information overload) and effectively tailoring it. Through the development of transparency resources and engagement with diverse stakeholders, we have observed that standard model cards, while technically comprehensive, often fail to communicate key information to non-specialists. In response, Anthropic developed a simplified “model report” on its Transparency Hub that highlights essential facts and evaluation results, making it easier for non-specialists to understand model development practices and capabilities and make comparisons across models. - 2. Streamlining reporting requirements
A second challenge involves establishing coherent transparency standards across numerous voluntary commitments, international frameworks, and emerging regulations that enable meaningful industry comparisons. The proliferation of reporting requirements creates a patchwork of overlapping expectations. For example, the G7 Hiroshima AI Code of Conduct and Seoul Summit commitments ask for similar information, such as safety evaluation methods, but in inconsistent levels of detail, making it difficult for stakeholders to compare practices across companies effectively. This fragmentation ultimately undermines transparency’s core purpose: giving the public clear visibility into AI development practices. The OECD G7 Hiroshima AI Process Reporting Framework begins to address this need by creating a standardised structure for AI companies to report their practices, enabling more effective reporting while ensuring comprehensive coverage of key transparency elements. - 3. Adapting to evolving technology
The third challenge is that transparency frameworks need flexibility to adapt as technology and models evolve. For instance, through developing and updating its Responsible Scaling Policy, Anthropic observed that model evaluation methodologies can quickly become outdated as the field’s research and understanding deepen. What constitutes meaningful transparency for today’s models may not capture the relevant dimensions of tomorrow’s systems. Therefore, reporting frameworks should maintain sufficient flexibility to accommodate the fast-changing nature of AI development.
Standardising transparency for responsible AI evolution
Transparency in AI development is an ongoing process that must evolve alongside the technology itself. Ultimately, transparency should serve diverse stakeholders while establishing practical standards that can inform future regulations and build the trust necessary for responsible AI development.