Basic safety requirements for AI risk management
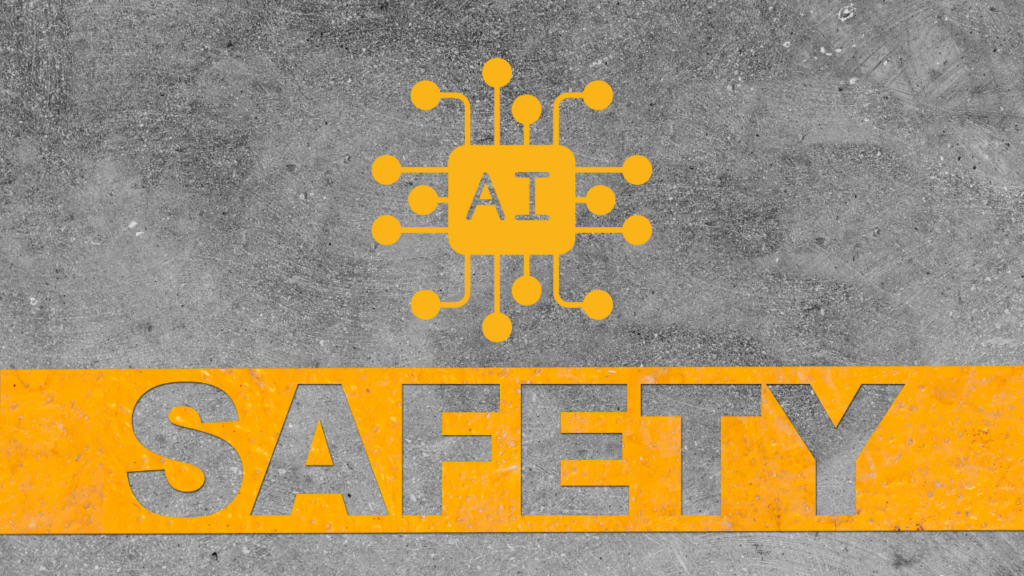
“Development of Superhuman Machine Intelligence (SMI) is probably the greatest threat to the continued existence of humanity,” says Sam Altman, CEO of OpenAI. He argues that SMI is more dangerous than even the worst imaginable viruses.
Despite this, labs explicitly attempting to build such AI systems do not consistently meet basic safety standards. As the scaling of large language models has led to systems with “sparks of artificial general intelligence” in large language models, the risks they pose remain unmanageable.
As a member of the European Standardization working group (CEN CENELEC) responsible for developing AI risk management standards for general-purpose AI systems, I believe it would be a disservice to recommend “countermeasures” when we lack any guarantees or understanding of the effects they would have. One thing is sure, current and next-generation general-purpose AI systems must satisfy basic safety and risk management properties.
Safety nets are feeble
Like any industry, AI carries its share of risks, including accidental ones, as demonstrated by Bing threatening users. Some worry about existential accidental risks, including a substantial fraction of AI experts. Among those expressing concern are Geoffrey Hinton, the godfather of the deep learning revolution and Dan Hendrycks, a leading Machine Learning researcher, an expert in evaluating AI systems, and the inventor of GeLU, an important component of most frontier AI systems.
Zooming out, a poll on US public opinion reveals that 46% of the population is “somewhat” to “very concerned” about AI existential risks. If those worries are right, worst-case accidents could cost everyone’s lives. We thus need to employ unprecedented safety measures to develop frontier AI systems.
Unfortunately, that is not happening. AI safety processes are extremely far from the standards applied in industries that are much less dangerous.
Let’s examine how other industries address accidental risks and what lessons can be drawn for AI risk management.
How other industries deal with risks
Risk management has long been a staple of various industries, with many adopting practices that AI could use for adequate regulation. We can categorise these industries into two groups.
The first group includes industries where deployed products must adhere to specific requirements to be sold. Examples include the automotive and aviation industries, which have safety standards that companies must follow to deploy their products. For instance, ISO 26262 is a standard for the safety of electronic systems in cars incorporating safety levels, like Automotive Safety Integrity Levels (ASILs), to determine the required safety measures for different system components. For instance, having brakes that don’t work is substantially worse than warning signs that don’t work and thus require more stringent safety measures.
Let’s look at the way these levels are computed:

- The severity is an assessment of the potential damages if an accident occurs.
- The exposure is an assessment of the likelihood that if this specific subsystem doesn’t work, it will lead to an accident.
- Controllability is an assessment of how easy it would be for a driver to avoid an accident if the subsystem fails.
This basic analysis can’t be run on language model systems, including monitoring systems and others used during deployment to determine the interaction between humans and AI systems. Firstly, because there is no comprehensive understanding of AI systems’ capabilities, making it difficult to assess the severity of potential failures with high confidence. This is particularly worrying for worst-case scenarios, given that it’s impossible to imagine how bad a failure can be.
There is also very little modularity of large language model systems, which prevents analysis of the exposure arising from subsystems. This significantly limits the ability to fix mistakes because of the complete lack of understanding about the specific reason why a model fails. The main safety mechanisms in place today are specific training data meant to make models less risky on average and some fairly simple monitoring mechanisms.
Finally, controllability can probably be estimated by assessing to what extent humans are in the loop at any given time during the deployment of models. The current trend is less and less.
Although large language model systems are potentially more transformative than cars, we cannot assess their risks with the same rigour.
The second group comprises industries that must follow strict requirements during the research and development process to prevent accidents. An example is the study and modification of viruses, which necessitates Biosafety Levels (BSL) based on virus lethality. There are four BSL, that correspond to four levels of risks among viruses according to their lethality, whereas more stringent safety requirements are applied for more dangerous viruses.
While some believe that AI is vastly more dangerous, the BSL-1 of AI does not yet exist.
If proper risk analysis were feasible and conducted for the most advanced AI systems, we could establish AI safety levels and implement adequate precautions around advanced AI development. Here is one way to proceed:
- Committees of experts could perform risk assessment, i.e. description of how advanced AIs can predictably cause damages accidentally. Those risks should then be categorised into levels.
- Technical experts would then need to design reliable monitoring techniques to spot accident precursors.
- For each risk level, there must be safety countermeasures. The development of more advanced systems would then only be allowed if experts can identify the countermeasures for a given risk level.
Unfortunately, the risk level associated with current systems might already be extremely high. The AI investor Ian Hogarth and producer of the report State of AI 2022, warns in his article in the Financial Times that we might already be close to God-like AI.
More concretely, the Bing accident, where GPT-4 helped synthesise chemical and bioweapons, or that GPT-4 or systems derived from it can be used to find cybersecurity exploits suggests that if a comprehensive risk analysis was performed, GPT-4 probably couldn’t be deployed.
Indeed, the risk it presents on the global cybersecurity infrastructure itself could be enough to wait a few years before we are able to make sure the world’s digital infrastructure remains stable as hacking techniques get radically more advanced. The real reason we feel safe is that we don’t accurately measure the risk.
For known risks, we should implement adequate monitoring techniques. Yet those monitoring techniques don’t exist. Meeting the standards of other less dangerous industries would likely require the development of effective interpretability techniques, which we also currently lack.
In the absence of techniques that identify all the risks and what happens when a model fails, we should apply the strictest safety standards. This is the standard we find in biosafety, where in the absence of knowledge of the lethality or transmissibility of a virus, viruses are automatically studied in labs that are the safest (BSL-4 labs).
Regardless of whether we consider AI to have accidental risks during development or deployment, understanding and quantifying risk is essential for effective management. Basic AI risk management practices must be implementable for models like GPT-4 and beyond. We should not allow further model development until we meet these criteria, just as we wouldn’t permit an airplane manufacturer to develop and deploy faster planes without resolving issues that caused a previous crash first.
We still do not know what caused Bing to threaten users, so we do not have a reliable fix for similar accidents. State-of-the-art models can still be used for hacking or to do explainers on how to craft bombs.
So what should regulators ask from AI labs?
Basic criteria for AI safety
There are three basic criteria I think will make AI risks manageable. Models need to be interpretable, boundable and corrigible in order to manage risks and how policies could help with that.
- Interpretable: A system can be interpretable when humans can reasonably predict what the model will do by looking only at its internal states. An interpretable AI system is more trustworthy because it allows us to have more guarantees about the model’s behaviour and understanding the source of a problem is essential to correct. It also helps to ensure the AI is truly performing the intended tasks rather than something else. However, supervisors may find it difficult to make that distinction.
- Boundable: A more ambitious yet essential characteristic for safely operating advanced AI systems is the ability to bound the consequences of their behaviours. This means guaranteeing that a model won’t fail with more than a certain probability or will fail within predictable bounds. For instance, I can’t predict how fast a given human runs, but I can say that they will run at a speed lower than 100 km/h. We should have equivalents for advanced AI systems. Those bounds can be very large. In the example below, we see that the bounds for planes are that they can’t fly at less than ~10km away from each other horizontally and 300m vertically. Large bounds can be sufficient for safety but we need to be extremely confident in some bounds, which is currently not the case for AI safety.
- Corrigible: As AI systems become increasingly capable, they will become, over time, capable and willing to preserve themselves from modification and being shut down. Anthropic has found the first behaviours among the largest models. Making sure that at any point in time, a human can shut down a system or change its objective, i.e. that it remains corrigible, is extremely important from a safety perspective. Thus such considerations should be carefully tracked.
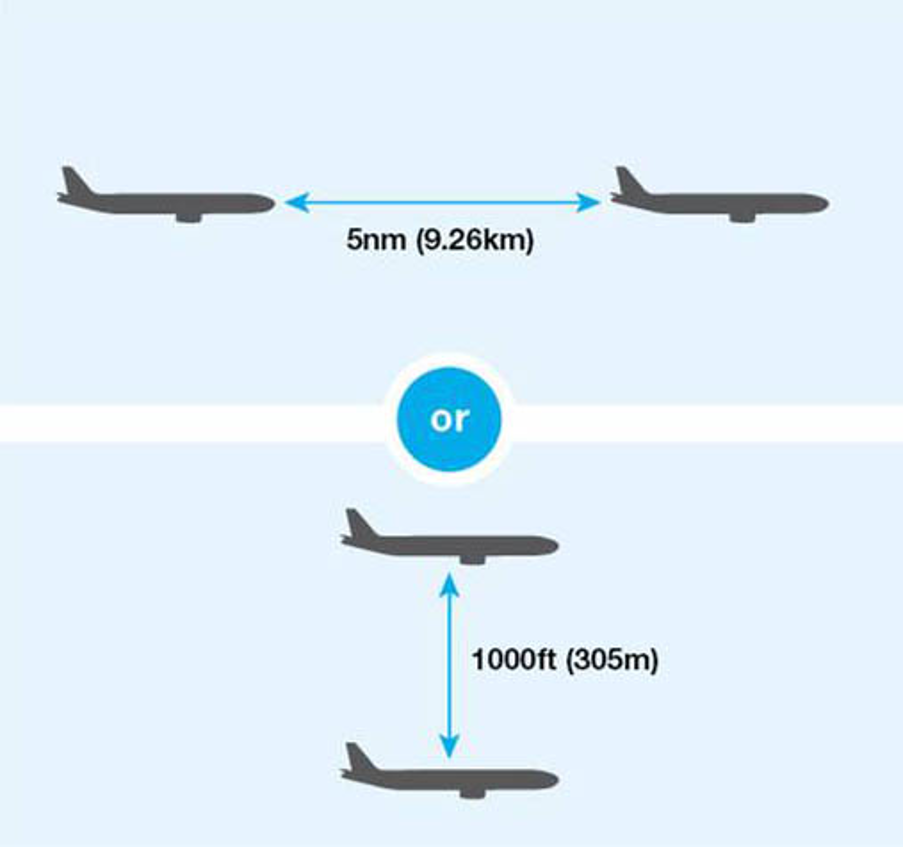
To avoid worst-case scenarios, we need policy makers from the EU, China and the US to make basic safety criteria and practices mandatory for developing and deploying more advanced general-purpose AI systems. Implementing such requirements in their regulation could make the world significantly safer.
We’re in the early stages of general-purpose AI. Quite logically, the field first tried to build useful systems, which was a hard first step. Normally, the easiest way to build something useful is unsafe by default. Now that we have powerful systems with a massive impact on the world, we should prioritize safety criteria such as the ones outlined here and design next-generation systems that are compliant. While these criteria may seem unrealistic now, having a system which passes the bar exam and succeeds at coding tests to get hired as a software engineer also seemed unrealistic five years ago. We need these safety measures, given the stakes.
Meeting these standards will make it much easier to move forward while avoiding large-scale catastrophes. “Aviation laws were written in blood” is a common saying referring to the fact that most regulation has been reactive to major accidents. Let’s not reproduce that methodology with AI.