Will businesses or laws and regulations ever prioritise environmental sustainability for AI systems?
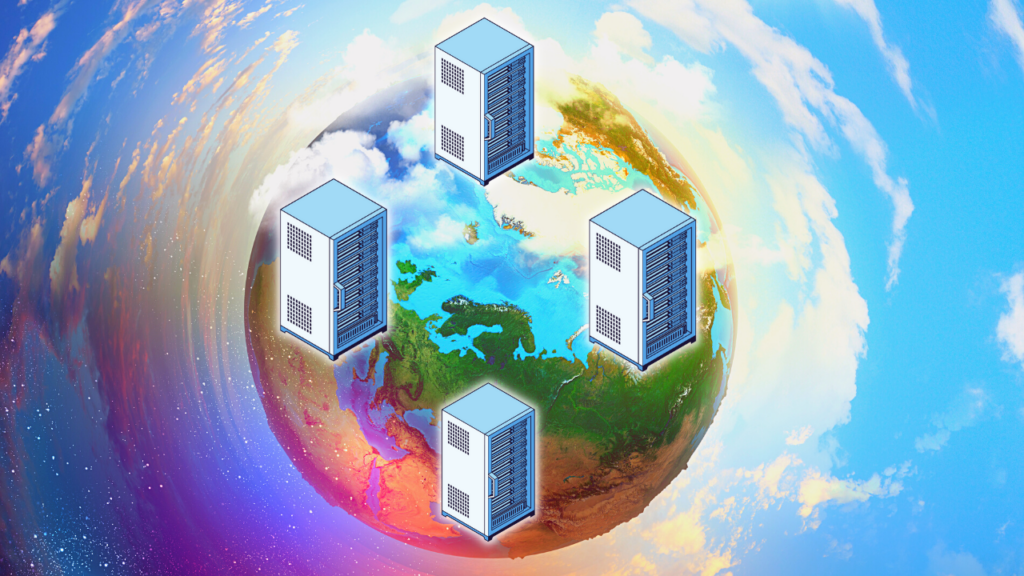
We are in the midst of a climate crisis, and Artificial Intelligence (AI) is being touted as an essential tool to solve some of the causes and effects of climate change. But before relying on it too heavily, we must evaluate the environmental impacts that AI itself has. The OECD and GPAI are working on it, and in light of their findings, it is clear that this is an area where legal and policy fields need to focus more attention. Indeed, the environmental effects of “AI compute” have often been overlooked in the past.
“AI compute” refers to the computational power and resources required to train and use AI models, and there is no doubt that its infrastructure carries carbon impacts. The training phase, where the model learns to recognise patterns based on vast input data, can require hundreds of computer chips. A typical large AI model consumes between 100-200 MWh of energy during training, or 10-20 times the yearly usage of an average single-family home in the United States.
This is why it is important to include the sustainability of AI systems in laws and regulations for AI to mitigate its environmental impact during the model design phase rather than being an afterthought.
There is no environmental regulation for AI
Many countries have published rules and guidelines for regulating emerging AI systems, but few explicitly call for environmental sustainability. Those that do often regard it as a voluntary risk management measure. Few municipal regulations directly address the environmental sustainability of AI compute. Some jurisdictions, such as the European Union, the United States, and the United Kingdom, have drafted preliminary guidelines on how to regulate AI systems. Still, they tend to focus on protecting consumer and business interests and safety.
In May of 2023, Members of the European Parliament requested additional be added to the EU AI Act, to address environmental sustainability. Before, only one paragraph in the creation of codes of conduct addressed environmental sustainability, “which aim to encourage providers of non-high-risk AI systems to apply voluntarily the mandatory requirements for high-risk AI systems […] for example, [commitments] to environmental sustainability.” Now, harm to the environment is classified as a high-risk area, and foundation model providers must abide by transparency and disclosure measures. Without specific environmental regulations like these, companies may not ensure the environmental sustainability of their AI systems.
Tools to measure the environmental impacts of AI compute and support regulation
Companies will need standards and tools to implement new sustainability regulations. Existing tools that measure the environmental impacts of AI compute have their limits. Most estimate the carbon impacts of AI using a form of the following equation:
CO2 Equivalent = Carbon Intensity x Power Consumed
Carbon intensity refers to the amount of carbon dioxide produced per amount of electricity consumed, measured in kg CO2 per kWh of electricity. The power consumed directly measures the electricity usage of the hardware, including the computer’s memory and processors.
However, it is a challenge to estimate power consumed if it is not directly measured. Tools such as CodeCarbon and Carbon Tracker, use software-based tools to estimate power consumption. While these are cheap and easy to install, they are generally less accurate than hardware power consumption measurements. Other tools like Green Algorithms and ML CO2 Impact estimate power consumption using information about the hardware, runtime, location, and type of platform used for AI training.
Another approach is to consider the size of the model. Larger models with more parameters tend to have higher power demands. Some practitioners use the model size as a proxy for power consumption when they do not have information about the hardware and training time. However, this is not a direct relationship. Figure 1 shows an example of the variation in carbon production between models of similar sizes.
For existing tools to effectively compute the carbon footprint of AI, the industry has to establish standard reporting practices for environmental metrics. A good starting point would be to report the hardware used for training, the power consumed by the hardware, the duration of the training process, and the location.
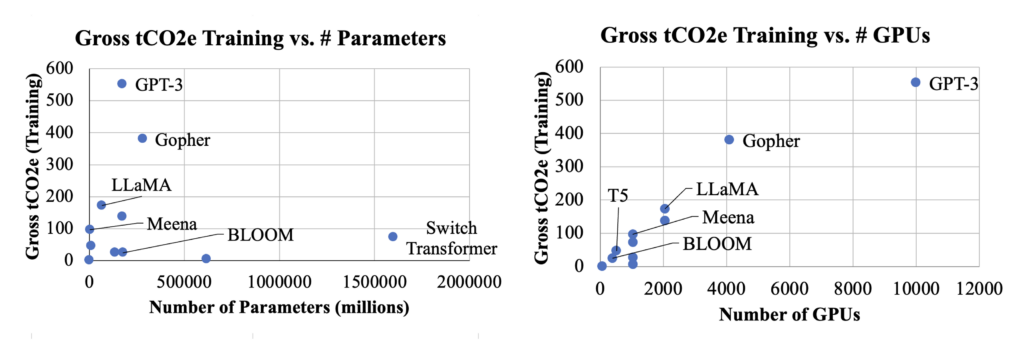
Data centre regulations can moderate AI energy consumption
As policies and tools to address AI’s environmental impacts take shape, non-AI sustainability initiatives can serve as references. Data centres that host computing infrastructure for servers, storage, and networking are key components of the AI compute stack. They attracted regulatory attention due to their large energy consumption. The construction industry also reckoned with its high greenhouse gas emissions via sustainability certification processes, a model that could be applied to AI compute. Here are a few initiatives by key industry players that have taken action even without official policy.
Data centres consume energy and produce greenhouse gases. In its 2023 Environmental Report, Google said that its data centres produced up to 2.5 million tons of CO2 equivalents. Some countries and cities have imposed legislation for data centres to respond to high energy demands and negative environmental and economic impacts.
Some governments have introduced data centre moratoriums. In July 2022, Frankfurt, Germany, announced a plan that limits data centre building for commercial developments to certain specified areas. In June 2022, the town Planning and Zoning Commission in Groton, Connecticut, voted to place a one-year moratorium on large data centres, giving the commission time to decide on the best way to develop new regulations for businesses. City officials are considering “limiting data centres to light industrial zones and regulating data centres that are 10,000 square feet or larger.” Other jurisdictions previously imposed moratoriums, including Ireland, Amsterdam, and Singapore.
Pauses on data centre growth may not be a realistic long-term solution. It also remains difficult to separate AI-specific measurements from general-purpose compute, making data centre energy use only a rough proxy for the environmental impact of AI. Still, the examples above illustrate regulatory precedent and citizens’ demand for accountability. Data centre regulations deserve continued attention as AI compute is expected to drive up the cost and energy consumption of data centres.
Industry leaders could expand climate initiatives to artificial intelligence
The expansion of big data and cloud computing in the 2010s pushed big tech companies to address the carbon impact of data centres. Google, Amazon, and Microsoft addressed sustainability through commitments to be carbon-free or negative by 2030. Although they still use fossil fuel energy in the data centres, the companies offset their carbon impacts through renewable energy credits. Google went a step further by promising to power its business with carbon-free energy 24/7. However, these promises do not address operations related to AI. Generally speaking, they report on how they are using AI to address the climate crisis (flood forecasting, cataloguing environmental data, etc.) but admit that “predicting the future growth of energy use and emissions from AI compute in [their] data centres is difficult.”
Standardised reporting impels companies to acknowledge the environmental impact
Some companies are reporting the carbon footprint of their AI models in technical publications. One report calculates detailed energy usage and carbon footprint estimates on four in-house Google AI models and GPT-3 while carefully documenting the methodology and assumptions. Meta directly acknowledges the large carbon impact of its recent foundation language models, LLaMA, and published a table of hardware energy consumption and estimated carbon emissions (equivalent tons CO2). One of the best examples of carbon reporting was live-tweeting the training process behind “BLOOM” (BigScience’s Large Open-science Open-access Multilingual Language Model), which published detailed environmental-impacts metrics.
This type of energy reporting may expand further, which would be welcome, but may not gain traction until suitable regulations are in place.
Sustainability certification in other fields can serve as inspiration
Currently, no institution or process exists for a company to certify AI model sustainability standards. In the construction industry, the Leadership in Energy and Environmental Design (LEED) program encourages sustainable construction through green building certification. LEED, created by a non-profit organisation, serves as a tool to help owners and operators evaluate their designs’ efficiency. A similar rating system for AI models could help embed sustainable thinking into the design phase of AI systems. The rating could consider the model architecture, the computing hardware, the data centre location, and the model training strategy.
Industry leaders such as NVIDIA or Google could spearhead a certification process. Figure 2 shows the popularity of Google’s Tensor Processing Unit (TPU) and Graphics Processing Units (GPUs) from NVIDIA for training over a dozen large AI models, including GPT-3, LLaMA, and BLOOM. If key industry players developed or adopted a sustainability certification process, it could be used as a standard across the field.
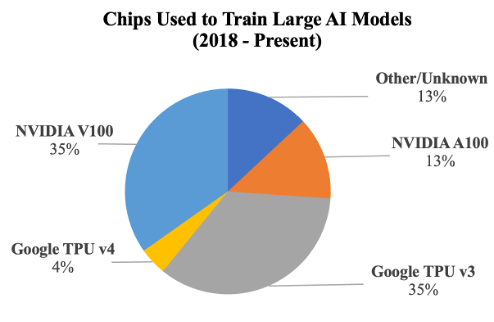
In the meantime, companies can still improve their standards and transparency
Although AI laws and regulations have been promulgated with little to no mention of environmental sustainability, countries and organisations must revise their own standards and create new regulations to take their carbon footprint into account. A lack of tools and gaps in measurement standards for AI compute make this task challenging. Despite the gaps, some big industry players have committed to carbon neutrality and reporting on the environmental impacts of their AI models. Governments and organisations can look to those positive examples to inform broader regulations. Since the ultimate goal is to introduce sustainability into new AI regulations, there is an opportunity to take advantage of the momentum around global climate change. All actors should explore how individual movements and efforts can bolster support for environmentally sustainable AI.
This blog post comes from a partnership between the Duke University Initiative for Science & Society and OECD.AI as part of the Ethical Technology Practicum.
The Ethical Technology Practicum is a course designed and taught by Professor Lee Tiedrich at Duke University through the Duke Science and Society Initiative. Students in the Law and Public Policy Programs collaborated with OECD.AI to research the use of AI in the workplace. This blog post and students’ work are provided for informational purposes only and do not constitute legal or other professional advice.
Figure sources and methodology
We conducted a survey of approximately 20 large AI models, contingent on the availability of information, to study trends in model size, energy consumption, and environmental impact using publicly available data. While the survey includes many popular AI models, this data is not comprehensive and does not attempt to draw conclusions across the entire field of foundation models.
Some models have been trained by multiple parties with different hardware or training strategies. The figures use author-published and/or official sources. Other configurations may lead to different training times, energy consumption, or equivalent tCO2 production. The sources below directly quote all statistics shown in the figures above.