When AI generates work, standard contractual terms can help generate value and clarity
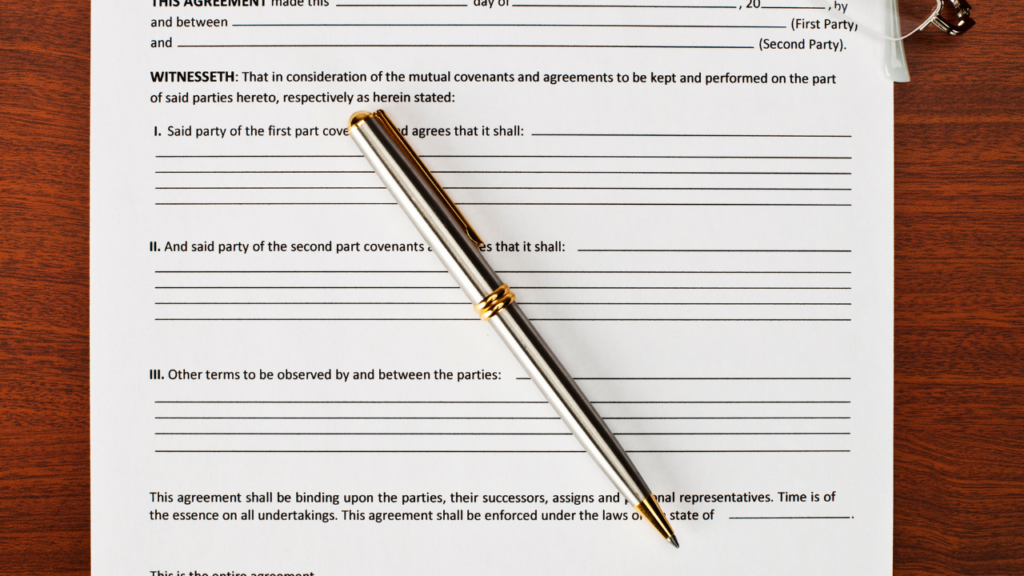
Works generated by Artificial Intelligence (AI) can have tremendous economic value. Back in 2018, Christie’s sold an AI-generated painting for $432,500, far exceeding the sales price of authentic Warhol and Lichtenstein works displayed in its gallery. Since then, AI-generated art has blossomed, sparking debates about whether Intellectual Property (IP) laws should protect AI-generated works and related developments and, if so, how such rights should vest.
The questions raised by AI-generated works and related developments extend well beyond art and impact many facets of society. Journalism, advertising, music, biotech, and other sectors face similar challenges. For instance, AI has improved drug discovery, stimulating further AI adoption and investment in this industry. AI produces significant amounts of computer code. It can also help design computer chips, industrial parts, and materials and generate synthetic data, which is critical for certain Privacy Enhancing Technologies (PETS). These AI-generated outputs can have significant economic value, as can the underlying AI models, the individual data sets used to train, test and validate such models, the aggregate training, testing and validation data sets, and the prompts used to query such models.
While the adoption and valuation of large language models (LLMs) and other AI developments continues to soar, IP laws do not clearly answer critical questions, such as who owns and has the right to use such works. The questions become even more complicated when multiple organisations contribute significant expertise and resources to create “co-generated AI works.” This uncertainty can deter responsible innovation because it can make the return on investment much less predictable. It also increases litigation risks.
Fortunately, contracts can help clarify the ownership and permitted uses of AI works and reduce risks, even when underlying IP laws remain uncertain. Contracts already fill this gap in many circumstances. However, to optimise their benefits, standard contract terms are needed. As explained in a recent report published by the Global Partnership on AI (GPAI), standard contract terms can reduce transaction costs, provide greater certainty for more organisations, and address an array of other issues. Developing common contractual definitions would advance this work.
IP challenges with co-generated AI works
Policy makers worldwide have encouraged responsible AI innovation, and many organisations are combining resources to create co-generated AI works. While expanding AI development, this raises novel and complex IP issues. For instance, an organisation might produce an AI model to help design automotive brakes. The model may include the organisation’s proprietary code as well as third-party code. To train the AI model, the organisation might rely upon its own data, proprietary third-party data, and publicly available data, at least some of which are scraped from third-party platforms. It may then use different third-party data to test and validate the trained model. The organisation may invest significant resources in performing data hygiene, developing APIs, and otherwise aggregating and preparing the data sets for use with its AI models.
Once trained and validated, the AI model may be licensed to the organisation’s customers, who use prompts to query the model. The customers may experiment with their own prompts as well as third-party prompts. “Prompt engineering” has emerged as a new field. Queries based upon these prompts may then result in patentable brake components.
This hypothetical highlights the challenges of identifying the inventors and owners of co-generated AI works. To what degree should the organisation, its code and data suppliers, its customers, and their respective third-party prompt engineers have a stake in the resulting patentable brake components? What interests should they have in the trained AI model or the aggregate data sets used to train, test, and validate the AI model? What happens if two or more entities produce the same prompts or the same patentable results? How might trade secrets or copyright laws protect AI prompts? How might trademarks and trade secrets be leveraged to enhance protection? AI value chain participants will want answers to these questions as they decide how best to engage in AI innovation.
The evolving IP legal landscape
Unfortunately, today’s IP laws are not well-equipped to provide definitive answers to these critical questions. As a threshold matter, countries differ on whether and to what extent AI-generated works qualify for IP protection. This can have important commercial implications, given the value typically associated with IP.
With respect to patents, many jurisdictions currently require human inventors, including the United States, Australia, the United Kingdom, the European Patent Office, Germany, Brazil, and Israel. South Africa, in contrast, recognises AI patent inventors. Even with this guidance, the patent legal landscape remains unsettled. An AI inventorship case is pending before the UK Supreme Court. In the US, the Patent and Trademark Office launched a series of public engagements on AI and inventorship.
The legal uncertainty also extends across the copyright landscape. Several jurisdictions, including the United States, Korea, Australia, and the European Union, require human authorship of copyrighted works. However, the level of required human involvement for copyright protection remains somewhat unsettled. For instance, the US Copyright Office recently denied copyright protection for AI-generated images in the comic book Zarya of the Dawn, but confirmed that the human author has copyright protection for the text as well as the “selection, coordination, and arrangement of the work’s written and visual elements.”
As part of its recently launched AI Initiative, the US Copyright Office issued guidance on the level of human authorship required to obtain copyright protection. Among other things, this guidance indicates that when an AI model receives a directional prompt, the resulting work will not have copyright protection if it lacks sufficient human creative control. The US Copyright Office plans to seek further public input soon. Meanwhile, other jurisdictions, such as Canada and India, have entertained copyright co-authorship for works developed jointly by humans and AI.
Contracts can increase certainty
Parties entering AI collaborations typically want clarity upfront on their ability to own and control the fruits of their creative efforts. Fortunately, contracts can reduce some uncertainty presented by IP laws, which is important given the dramatic rise of AI-generated works. Specifically, contracts can help organisations structure collaborations to include sufficient human involvement, reducing the risk of forgoing IP protection. They can also include confidentiality and other provisions to help secure trade secret protection, which may be preferred, particularly when patent protection seems too improbable or expensive. Additionally, contracts can override some default rules and ambiguities under current IP laws with clearer and mutually agreeable terms. This practice is commonplace in many technology transactions, including some AI terms of use agreements. For instance, Open AI’s Terms of Use state:
“You may provide input to the Services (“Input”), and receive output generated and returned by the Services based on the Input (“Output”). As between the parties and to the extent permitted by applicable law, you own all Input. Subject to your compliance with these Terms, OpenAI hereby assigns to you all its right, title, and interest in and to Output.”
Creating standard AI contract terms
Developing standard contract terms can extend greater certainty and other benefits to more organisations, including Small and Medium-Size Enterprises (SMEs). Importantly, it can help address bargaining power inequities by offering organisations a menu of diverse options for contractually allocating rights, responsibilities, and risks. Additionally, it should lower transaction costs by reducing the need to develop novel and often complicated contractual terms that typically lead to lengthy negotiations.
Standard contract terms can also potentially increase open data sharing, like Creative Commons and Open-Source standard licenses have facilitated royalty-free content and software sharing. These benefits should enhance competition by decreasing barriers to entry, another important societal goal. They can also advance policy efforts to foster greater data sharing, such as the US Federal Data Strategy, the EU Data Governance Act, and the New Zealand Net-Zero Data Public Utility.
Definitions
As discussed in the GPAI Report, to advance standardisation, attention must increase on developing common contractual definitions. The definitions should take into consideration various elements of the “AI co-generation” value chain, including the following.
- Original input data. A contractual term may be needed to refer to data in the form first made available for the AI collaboration. Parties may want to have different terms to distinguish between original data provided for AI model training and original input data provided for other purposes.
- Processed data set. One or more terms may be needed to define the data set developed using original input data. As explained in the GPAI Report, this could potentially encompass: (i) a cleansed data set developed by performing data hygiene on original input data and (ii) any data compilation, database, insights, or metadata developed using original input data, either in its original form or after undergoing data hygiene.
- Untrained model. Parties may want to have a definition for an AI model that has not been trained.
- Trained model. Parties may want to have a definition for a trained AI model and/or specific parameters, such as weights.
- Trained model outputs. This definition could refer to the outputs of the trained AI model, such as the patentable automotive brakes discussed above.
- Prompts. This definition could refer to prompts created to query the trained AI model.
Multi-stakeholder collaboration
Developing standard contractual definitions and other terms requires multi-disciplinary collaboration. This will help ensure that the resulting legal terms align with the desired technical, business, and ethical approaches. To foster fairness, the drafting process should also integrate the viewpoints of different stakeholders, including SMEs and historically under-represented people. Where relevant, it also should draw upon existing legal practices and emerging policy developments, such as the OECD Framework for the Classification of AI Systems and potentially government procurement rules. This inclusive process should lead to better results and quicker adoption.
Stakeholders should get involved
Given the rapid pace of AI developments, there is no time to waste in developing standard contract terms that can lower barriers to entry and provide more certainty for AI innovators seeking to unlock the benefits of responsible data and AI. However, much work remains to be done, including with respect to standardising definitions. Organisations that are interested and have relevant experience or perspectives should contribute to the process. The GPAI Report provides important information on how to engage. This work also has the potential to help inform policy makers about societal needs as the IP legal landscape continues to evolve.
Thanks to Alban Avdulla, a recent LLM graduate of Duke Law School, for his research assistance.