Responsible science communication can be a catalyst for trust in technology
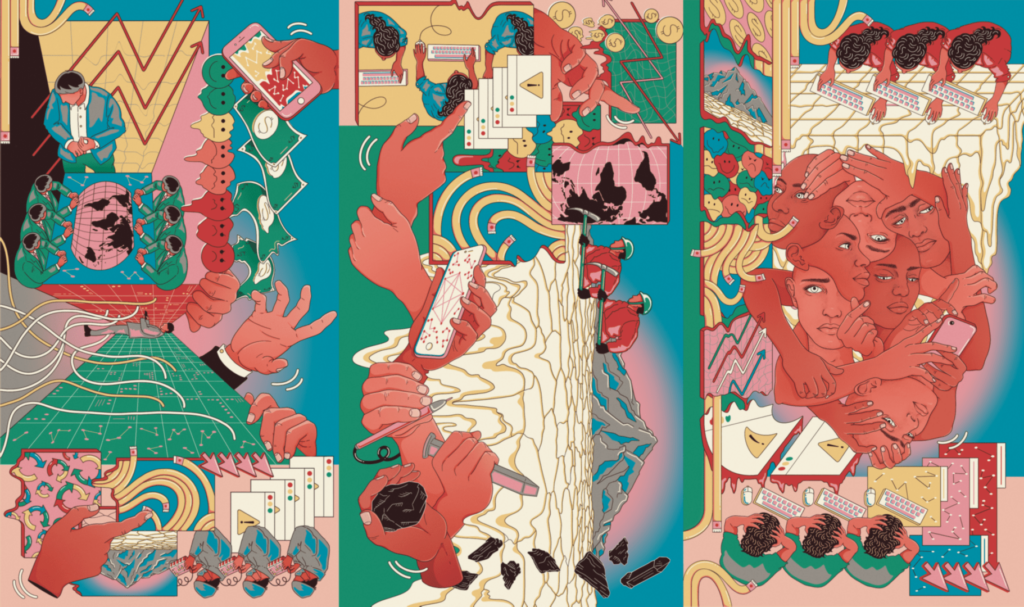
In brief
- Responsible science communication is essential for accurately conveying scientific information and building trust in emerging technologies like big data and AI.
- Miscommunication and hype surrounding technological advancements can lead to misunderstandings and hinder progress.
- To foster a more transparent technology future, organisations should communicate more to the public about science and in ways that accurately reflect the capabilities and limitations of emerging technologies.
In an era of rapid scientific advancements and technological breakthroughs, responsible science communication is paramount. It can simplify understanding scientific concepts, promote scientific literacy, build confidence in innovation, and inform decision-making.
Miscommunication and ambiguities in the way that we talk about new scientific or technological advancements can lead to misunderstandings, misinterpretations, and even misinformation. Responsible communication about scientific innovation is vital to ensuring that knowledge is accessible and understood by organisations, governments, and the public.
There should be more responsible dialogue about data limitations
Unprocessed raw data does not hold immediate value or meaning. Only when processed and interpreted using analytical techniques and algorithms does data provide valuable insights. However, in the discourse surrounding ‘big data,’ we are often confronted with metaphors that liken data to natural resources like oil or gold alongside nature-oriented language, such as ‘data deluge’ and ‘data lake.’ These standard ways of communicating about big data make up the dominant big data narrative and feed into a problematic cycle of hype. These metaphors perpetuate the notion that data is a neutral and objective reflection of reality we are collecting instead of constructing.
Further, dominant narratives downplay the human efforts required to generate business-relevant insights throughout the entire data lifecycle. Relevant human labour comes via engineers, developers and people performing data enrichment tasks such as data labelling, transcription, and content moderation, often for substandard pay.
For example, data workers in Kenya were tasked with reviewing countless text passages filled with extreme violence, sexual abuse, and self-harm to train generative AI safety filters for less than two dollars an hour.
By framing data as objective and decentering human labour in their lifecycle, we overlook the biases, assumptions, limitations, and power structures that are inevitably baked into data. Each decision introduces subjectivity, such as determining what data to collect, which variables to measure, and how to interpret and analyse the data. These choices can significantly impact the outcomes and conclusions drawn from data in downstream applications via analytics and machine learning. Further, it is crucial to recognise that datasets are not exhaustive, meaning that they cannot capture the full complexity of nature and, as such, are an abstraction and simplification of reality.
By accounting for data collection and analysis limitations, we can foster a more responsible dialogue on data and its value as a way of knowing. Understanding that data alone does not guarantee accuracy, exhaustive representation, or objectivity is crucial. Rather, it reflects the biases and limitations of its collection methods, the algorithms used to process it, and the dense confluence of power and social structures undergirding the technology landscape.
AI narratives should focus more on immediate risks
Few technological innovations have captured the collective imagination as profoundly as AI. Like big data, AI has become a battleground for responsible science communication because of discrepancies between popular portrayals and nuanced realities. AI is often represented in discourse and media as it is in science fiction – think blockbusters such as Terminator, Blade Runner, and Ex Machina. While rich in creative capital, such portrayals are problematic when imported into the technology ecosystem for several reasons.
First, these narratives perpetuate the idea that the most significant risk associated with AI lies in the potential for human extinction. Examples of extinction rhetoric can be found in the recent statement published by the Center for AI Safety signed by academics and notable technology leaders, including Sam Altman and Bill Gates, as well as the Center for Humane Technology’s recent talk entitled the AI dilemma. For instance, take the claim that was popularised last year that half of AI researchers believed in a 10% or greater chance of extinction resulting from our inability to control AI. In fact, this ‘statistic’ was based on a survey of just 162 researchers – hardly a representative sample. These hyperbolic representations of AI risk overshadow more immediate ones, such as its potential to deepen societal inequalities rather than the unlikely scenario of total human eradication.
And communication is not just about text! In addition to textual narratives regarding AI, there is also a scarcity of accurate and inclusive AI-related images. Better Images of AI, a non-profit collaboration founded by Tania Duarte in association with various artists and advisors, has published an image library of responsible depictions of AI – everything from data labellers to silicon. Our headline image in this article is a sample from the Better Images of AI library, designed by Clarote & AI4Media.
In speaking about their critical work, Tania states:
“Most images in stock image libraries widely used to illustrate AI contain a limited number of science fiction-inspired tropes which are self-referentially reproduced and bear no relationship to the subject matter. Glowing brains equate machine directly with machine intelligence; research shows that the predominance of blue backgrounds renders the images distant, alienating and deterministic; white male hands in business suits holding up holographic images are seen instead of the myriad of people across the world involved in training models built from real materials and with real impact; imaginary white humanlike robots with Caucasian faces centre Western and colonial ideas of intelligence, imply humanlike levels of behaviour and performance, and cloak the human agency behind technology. Our work in enabling general AI literacy has been a challenge not just of teaching what technology is, but what it isn’t, and until it is better understood there cannot be the meaningful and inclusive discourse so fundamental to democracy. “
Second, when human-like characteristics are attributed to AI technologies, they exaggerate and sensationalise their capabilities. In theory, general AI, also known as strong AI or General Artificial Intelligence (AGI), reflects human-level intellect across multiple domains but remains a research challenge, as no current AI system has achieved that level of sophistication. In contrast, narrow or weak AI is designed for specific tasks like facial recognition, using techniques like machine learning within a defined context.
Foundation models like GPT-4 blur the lines between general and narrow AI because they can be broadly applied without being task-specific, a quality referred to as general-purpose AI without satisfying the conditions of AGI. As noted in a highly prescient paper by Bender et al. 2021:
“Contrary to how it may seem when we observe its output, a [language model] is a system for haphazardly stitching together sequences of linguistic forms it has observed in its vast training data, according to probabilistic information about how they combine, but without any reference to meaning: a stochastic parrot.”
Finally, as we explored with big data, depicting AI as sentient robots or entities distracts from the underlying accountability of the humans involved in AI development and decision-making processes. This portrayal deflects attention from the crucial role played by human creators, programmers, and designers in shaping the technology’s outcomes and ethical considerations.
How innovation leaders can practice responsible science communication
So, how can we practically refocus conversations to be more nuanced, accurate, and responsible? To get started, we’ve established five key steps to innovate with integrity:
- Be transparent: Organisations should communicate openly regarding the potential advantages, risks, and uncertainties of emerging technologies, fostering trust, and managing public perceptions. As Setiawan and Pijselman 2023 suggest, transparency doesn’t entail revealing trade secrets, but could involve disclosing AI system usage or intended model applications.
- Be clear: Organisations should avoid jargon and simplify terms to ensure inclusivity. Bullock et al. (2019) note that this strategy helps close the knowledge gap, enhancing public comprehension and support for technology by reducing resistance and risk perceptions.
- Be reflective: Organisations should actively listen and respond to public and stakeholder concerns, creating a meaningful dialogue. This approach values public input beyond mere formalities and ensures that concerns and questions are genuinely addressed.
- Be multidisciplinary: To improve science communication, organisations should work with experts from various fields, such as science, technology, ethics, and policy. This multidisciplinary approach acknowledges technology’s broad impacts, highlighting the need for more than technical insights in communication efforts.
- Continuously improve: Organisations must evaluate their communication strategies regularly, incorporate feedback, and make necessary adjustments to enhance their impact.
In the ever-evolving landscape of technology, responsible science communication is an essential catalyst for informed decision-making and responsible innovation. As organisations, governments, and the public grapple with the opportunities and challenges presented by emerging technologies, transparent communication about their implications becomes critical. Organisations can better innovate with integrity by being transparent, clear, reflective, multi-disciplinary, and embracing a continuous improvement mindset.
The views reflected in this article are the views of the authors and do not necessarily reflect the views of the global EY organization or its member firms.