A new approach to measuring government investment in AI-related R&D
The field of AI research has undergone a colossal transformation in the past two decades, morphing from a small, relatively niche domain into a sprawling web of ground-breaking ideas and innovations that are rapidly diffused. Mapping and measuring this research explosion – and the public funding underlying much of its ignition – is of prime importance to policy makers and experts, as is encouraging its further escalation on a sustainable trajectory oriented towards the common good.
A mandate to monitor AI R&D investment
The 2019 OECD Recommendation on AI states that governments “should consider long-term public investment, and encourage private investment, in research and development, including interdisciplinary efforts, to spur innovation in trustworthy AI”. Tracking government investments into research and experimental development (R&D) is of particular importance: R&D is by definition boundary-pushing and creative, which distinguishes it from other efforts that receive heavy investments and that may only involve implementing standard AI tools in well-studied contexts, often as business solutions.
No comprehensive method exists by which to track and compare AI R&D funding across countries and agencies. So far, proxy indicators such as scientific publications and patents have been used (also here) to chart the evolution of AI R&D efforts by assuming a direct relationship between inputs, or R&D expenditure, and outputs such as publications.
Read the new OECD study: Measuring the AI content of government-funded R&D projects
A proof of concept for the OECD Fundstat initiative, produced in collaboration with our colleagues Akiyoshi Murakami (OECD) and Izumi Yamashita, now at Japan’s National Institute of Science and Technology Policy, addresses this evidence gap.
This study looks to answer the questions “how much funding do government agencies give to AI-related R&D projects?” and “what types of AI research are most commonly funded?”. It applies a relatively simple method to classify the R&D projects found in the databases of funding agencies as either “AI-related” or “non-AI-related”. In this context, by saying “AI-related” we refer to projects that either contribute to creating or developing AI tools or that use available AI tools to make novel contributions to another field, such as medicine.
To classify or not to classify
AI is one of several R&D domains that are evolving at a breakneck pace. A survey-based approach that must rely on fixed definitions to measure and characterise R&D spending can amass highly valuable data, but it may not always succeed in fully capturing the diverse and fast-moving research landscape.
By the time such a survey is developed, vetted, and sent to R&D performers or funding organisations, important factors in the environment may have changed: research priorities and policy interests may have shifted, new sub-areas of study may have emerged, and the definitions or characterisations that the survey employs may have become obsolete.
As presented in this OECD study, an alternative, complementary approach is to create a list of key terms related to AI and check if those key terms appear in the project abstracts found in various government funding agencies’ databases. A project is then classified as AI-related if its abstract contains at least one core key term (i.e. a term unambiguously associated with AI, like “machine learning” or “computer vision”) or at least two non-core key terms (ambiguous terms with double meanings – “neural network”, which could refer to either an artificial or a biological network).
No public data, no problem
The rich databases of government-financed R&D projects with the information needed to conduct funding comparisons are not always available to the public. This is where the OECD’s unique strengths really come into play. The organisation leverages its close relationship with member and partner countries. It can work with the relevant funding agencies by sharing programming codes or scripts that the agencies can run themselves.
This allows its partners to extract the desired information in a way that does not compromise the required degree of confidentiality. In this case, the exchange involves the number of funded projects containing key terms, but potentially also more complex analyses. This approach has been successfully applied in the OECD microBeRD project for confidential business micro-data under the custody of national statistical offices.
Selecting the key terms
Our OECD project team conceived and implemented the following procedure to select key terms, which is simple and easy to explain and replicate:
- Create a “base” list of key AI terms by combining two pre-existing lists, one lifted from the NIH Medical Subject Headings (a hierarchically organised set of keywords) and one produced by researchers as part of a seminal paper on this subject.
- Transform each term in the base list into a numerical vector (or “word embedding”) using a standard word embedding model (word2vec).
- Vectorise each word in a corpus of AI journals (as tagged by their publishers). Compare the vectorised corpus to the vectorised base list. If a word in the corpus is found to be highly mathematically similar to the words in the base list, it is deemed a good candidate for the extended list of key terms.
- Prune the extended list of key terms, removing overly ambiguous terms.
- Repeat steps 3 and 4, this time extracting new key terms from the R&D project databases of the target funding agencies.
- Divide the newly created list of key terms into core and non-core terms based on ambiguity.
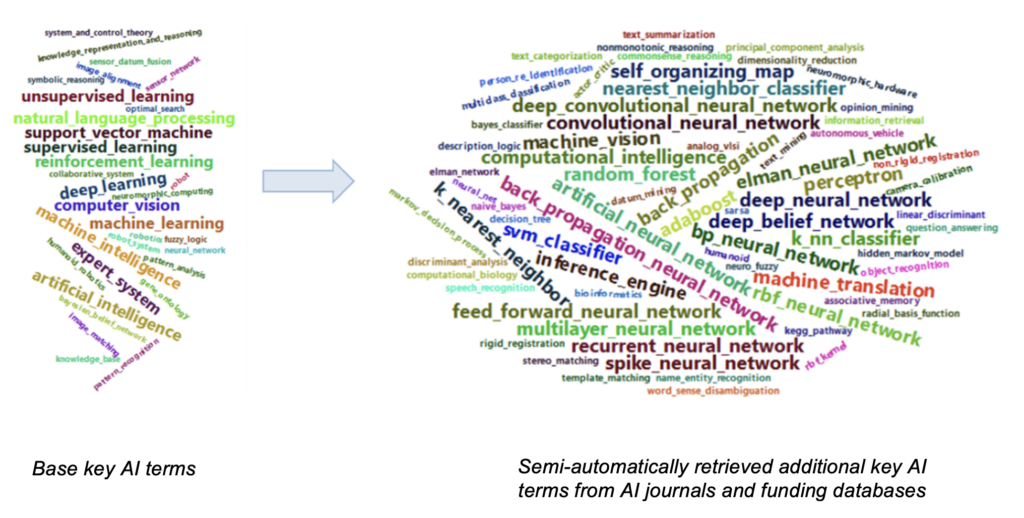
Our project team applied this methodology to funding databases belonging to a diverse set of agencies, ranging from the medical to the natural science and engineering-focused, and hailing from eight countries plus the European Commission. Funding streams for the following 13 agencies and bodies were examined: the Australian Research Council (ARC), the Canadian Institutes of Health Research (CIHR), Canada’s Natural Sciences and Engineering Research Council (NSERC), the programmes under the Spanish National Plan for Scientific and Technological Research and Innovation (PlanEst), France’s National Research Agency (ANR), the UK Research and Innovation’s Gateway to Research (GtR) for Research Councils and GtR for Innovate UK, Japan’s Agency for Medical Research and Development (AMED) and Japan’s Database for the Grants-in-Aid for Scientific Research Program (KAKEN), the Dutch Research Council (NWO), the US’s National Institutes of Health (NIH) and National Science Foundation (NSF), and the European Commission’s funding programmes registered under the Community Research and Development Information Service (CORDIS).
How much total funding do government agencies dedicate to AI research?
The total volume of AI-related R&D funding identified through this work in those funding streams skyrocketed from USD 207 million in 2001 to almost USD 3.6 billion in 2019, a seventeen-fold increase. However, this slightly overstates true growth because data were not available for all funding streams for the entire 2001-2019 period. For the group of agencies with data available over a common and sufficiently long period (i.e. excluding Canada’s NSERC, Spain’s PlanEst, Japan’s AMED, and the Netherland’s NWO), the total volume of AI-related R&D project funding increased from USD 525 million in 2008 to USD 2.2 billion in 2018.
Much of this surge is concentrated in more recent years, with EU funding doubling in 2019 compared to the previous year. In fact, aggregate reductions in funding occurred in 2006-7, explained by cuts in EU funds, and in 2014, when NIH AI R&D funding fell.
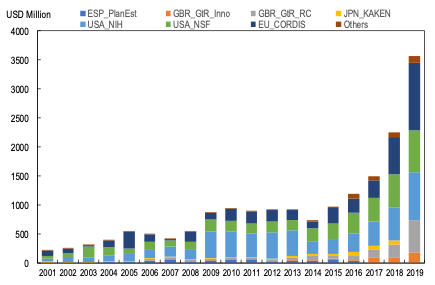
While this represents a very large amount, public funding for AI R&D may be dwarfed by business R&D investment, especially if one considers that a single heavily AI-reliant company like Alphabet reported USD 20 billion worth of total R&D expenses in 2018. The United States National Center for Science and Engineering Statistics provided a conservative estimate of business AI R&D investment at USD 9 billion out of over USD 160 billion worth of total software R&D.
As in many countries more AI PhDs choose to take jobs in private industry rather than in academia, it is important to put effective mechanisms in place to compare public and private funding and performance of R&D, from basic research to experimental development, using all tools and data sources available.
Which government agencies fund AI-related research?
Each of the 13 government funding agencies examined by the OECD invests in this “superstar” technology; however, some invest more heavily than others. When comparing sheer amounts of AI-related R&D funding for each one of these streams, the funding mechanisms covered by the EU’s Community Research and Development Information Service (CORDIS) have very recently become the single largest source, followed closely by the US’s National Institutes of Health (NIH) and National Science Foundation (NSF). These two US agencies account for over three-quarters of the cumulated AI R&D funding that we have documented in this exercise.
A different hierarchy emerges when examining the percentage of each agency’s total R&D spending that is accorded to AI research, an indicator of AI R&D funding intensity. By this measure, the leading agencies are NSF, the UK Research Councils, the Dutch Research Council (NWO), and Innovate UK, each of which dedicated in 2019 between 10% and 15% of total R&D funding to AI projects.
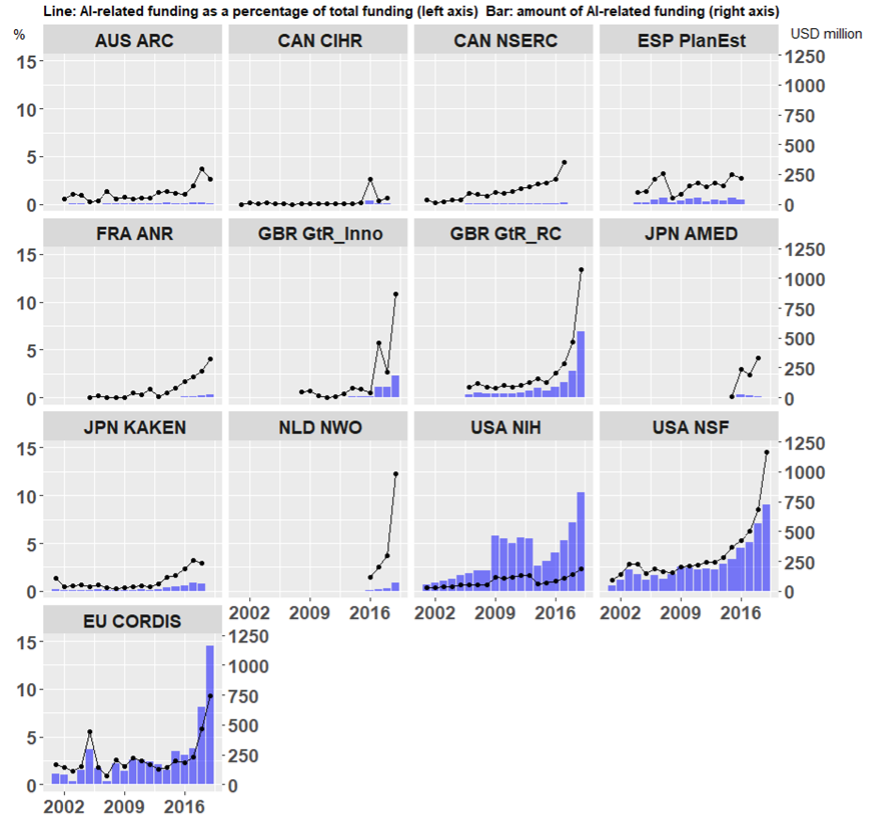
What types of AI R&D are being funded?
AI plays a role in R&D in different ways, as articulated in our working definition or AI relatedness. We have investigated this in more detail by exploring the topics of selected projects using topic modelling analysis. We conducted Latent Dirichlet Allocation (a popular statistical technique) to first identify agency-specific topics within their AI R&D portfolios and assign projects to them. After manually assessing commonalities across topics, we ended up with a total of 21 distinct topics that allowed us to compare different agencies. To simplify the presentation, we organized the topics under five larger themes:
- General AI techniques, incorporating the likes of machine learning
- AI prerequisites and impact (such as education and training and social impact).
- AI fields (such as computer vision and natural language processing)
- Medical AI applications, and
- Non-medical AI application areas (such as business and social sciences).
As shown below, six of the 13 funding streams most frequently financed AI projects that focused on particular fields or techniques (e.g. computer vision), while the other half tended to fund projects concerned with specific applications of AI, either medical or non-medical, depending on the agency’s domain of action.
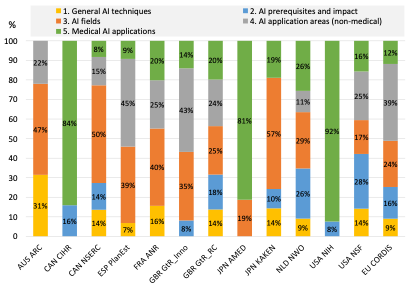
Towards a more comprehensive view of R&D funding: AI and beyond
As the field of AI forges ahead, maturing and expanding into myriads of subfields, mapping the funding that underlies this superstar technology will continue to be of utmost importance to policymakers and researchers alike. Through projects like this, and further collaborations with its member countries, the OECD can play an important role in developing and implementing high-quality mechanisms by which to measure and compare government funding, combining the strengths of official statistics and new data sources and methods. This pilot study will serve as a prototype for extending our analysis to more countries and more agencies, adding to the range of measurement tools and infrastructures provided by the OECD under the aegis of its Working Party of National Experts on Science and Technology Indicators.
AI, however, is far from being the only research field that evades easy definitions but whose emergence remains critical to track. We see this study functioning as a blueprint for creating broader mechanisms capable of assessing government contributions to a host of fields, including pandemic resilience and research related to the UN Sustainable Development Goals.