Updates to the OECD’s definition of an AI system explained
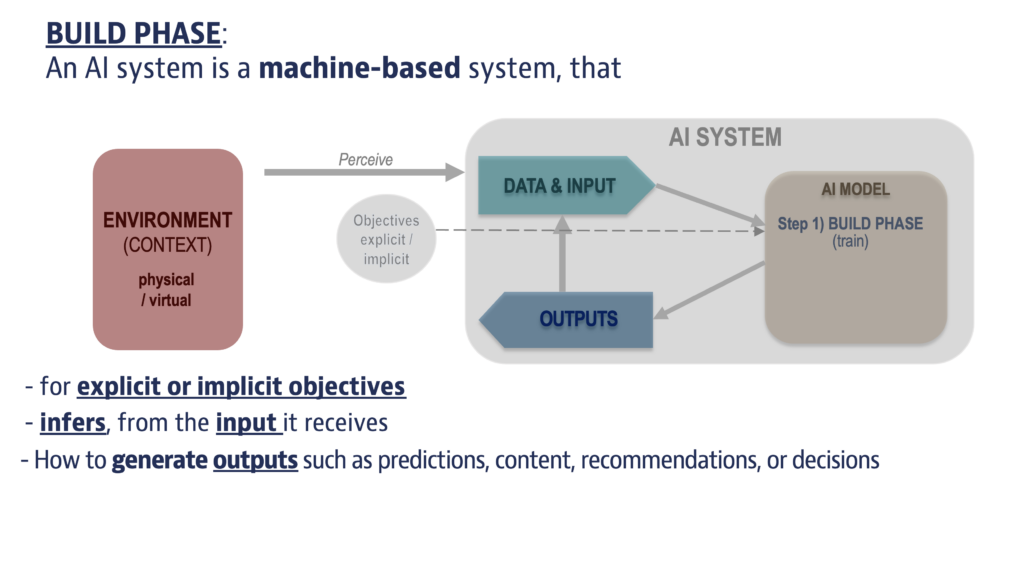
Obtaining consensus on a definition for an AI system in any sector or group of experts has proven to be a complicated task. However, if governments are to legislate and regulate AI, they need a definition to act as a foundation. Given the global nature of AI, if all governments can agree on the same definition, it allows for interoperability across jurisdictions.
Recently, OECD member countries approved a revised version of the Organisation’s definition of an AI system. We published the definition on LinkedIn, which, to our surprise, received an unprecedented number of comments.
We want to respond better to the interest our community has shown in the definition with a short explanation of the rationale behind the update and the definition itself. Later this year, we can share even more details once they are finalised.
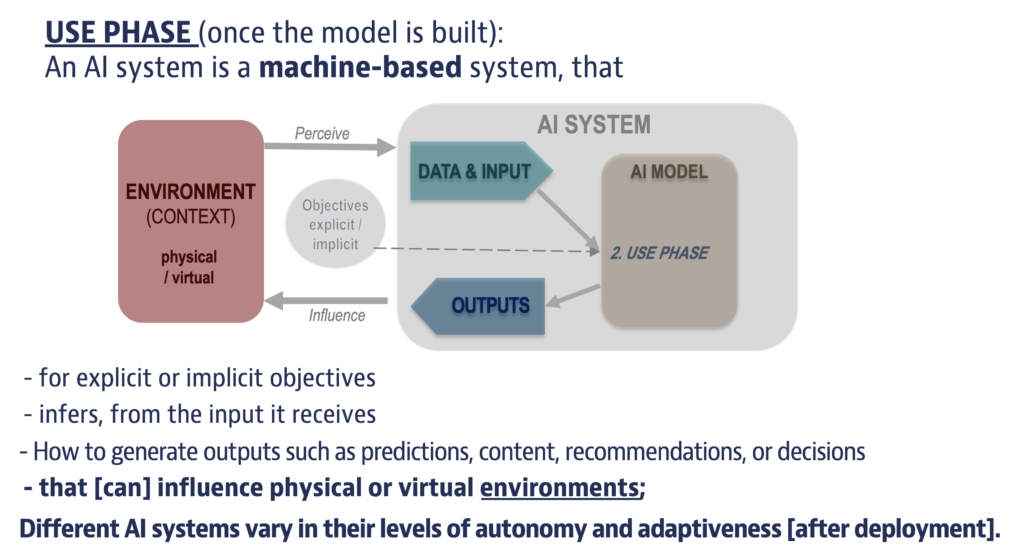
How OECD countries updated the definition
Here are the revisions to the current text of the definition of “AI System” in detail, with additions set out in bold and subtractions in strikethrough):
An AI system is a machine-based system that can, for a given set of human-defined explicit or implicit objectives, infers, from the input it receives, how to generate outputs such as makes predictions, content, recommendations, or decisions that can influenceing physical real or virtual environments. Different AI systems are designed to operate with varying in their levels of autonomy and adaptiveness after deployment
These changes reflect the following observations:
Description of the objectives: These edits seek to reflect the scientific consensus that an AI system’s objectives may be explicit (e.g., where they are directly programmed in the system by a human developer) or implicit (e.g., via a set of rules specified by a human, or when the system is capable of learning new objectives).
Examples of systems with implicit objectives include self-driving systems that are programmed to comply with traffic rules (but do not “know” their implicit objective of protecting lives), or a large language model like ChatGPT where the objectives of the system are not explicitly programmed but acquired in part through the process of imitation learning from human-generated text and partly from the process of reinforcement learning from human feedback (RLHF).
Inputs: The addition of “infer, from the input it receives” underscores the important role of input, including rules and data,, which may be provided by humans or machines, in operating AI systems. An AI system is said to “infer how to generate outputs” when it receives input from the environment and computes an output by processing the input through one or more models and underlying algorithms. For example, a visual object recognition system implemented by a deep neural network performs “inference”, i.e., infers how to generate its output (in this case, a classification of the object in the image) by passing its input (the pixels of the image) through the deep network (a parameterised algebraic expression composed of addition, multiplication, and certain nonlinear operations).
Outputs: The addition of the word “content” clarifies that the Recommendation applies to generative AI systems, which produce “content” (technically, a sub-set of “predictions, recommendations, or decisions”) such as text, video, or images.
Environment: Substituting “real” with “physical” clarifies and aligns with other international processes. Furthermore, contrasting real with virtual suggests that virtual environments are not real, which is not the case: they are real in that they accept real actions from the AI system and generate real sensory inputs to the AI system.
Adaptiveness: This reflects that some AI systems can continue to evolve after their design and deployment (for example, recommender systems that adapt to individual preferences or voice recognition systems that adapt to user’s voice) and is an additional characterisation of an important group of AI systems. Also, the previous wording, “operate with varying levels”, might be read as describing a single system whose level of autonomy and adaptiveness might change over time, which was not the intended reading.
In addition to the revised “AI System” definition, the OECD is working on an Explanatory Memorandum to complement the definition and provide further technical background. While the definition is necessarily short and concise, its application in practice would depend on a range of complex and technical considerations. The Explanatory Memorandum will support all adherents to the OECD AI Principles for better implementation.
Background on the OECD AI Principles and the AI system definition
The OECD AI Principles are the first intergovernmental standard on AI, adopted by OECD countries, the OECD Council at ministerial level to be precise, on 22 May 2019. The Principles aim to foster innovation and trust in AI by promoting the responsible stewardship of trustworthy AI while ensuring respect for human rights and democratic values.
Complementing existing OECD standards in areas such as privacy, digital security, risk management, and responsible business conduct, the Principles focus on AI-specific issues and set a standard that is implementable and sufficiently flexible to stand the test of time in this rapidly evolving field.
In its final provisions, the Council instructed the Committee for Digital Economic Policy to “monitor, in consultation with other relevant Committees, the implementation of this Recommendation and report to the Council no later than five years following its adoption and regularly thereafter”.
Accordingly, the OECD and member country representatives have begun working on that report. In the context of their discussions, OECD countries identified a timely opportunity to maintain the relevance of the Principles by updating the definition of “AI System” and agreed to pursue this update urgently before finalising the full report on implementation, dissemination, and relevance of the Principles.
Updating the definition is important substantively to ensure it continues to be technically accurate and reflect important technological developments, including with respect to generative AI.
Strategically, it would be a timely occasion to foster broad alignment between the OECD’s definition of an “AI system” and ongoing policy and regulatory processes internationally, including in the European Union and Japan.
By facilitating such alignment, the proposed update reinforces the OECD’s and the AI Principles’ foundational position in the international AI governance landscape.