How private applied AI R&D labs can make AI work for mid-size businesses
Private applied AI R&D labs are the key to the future competitiveness of European MSBs
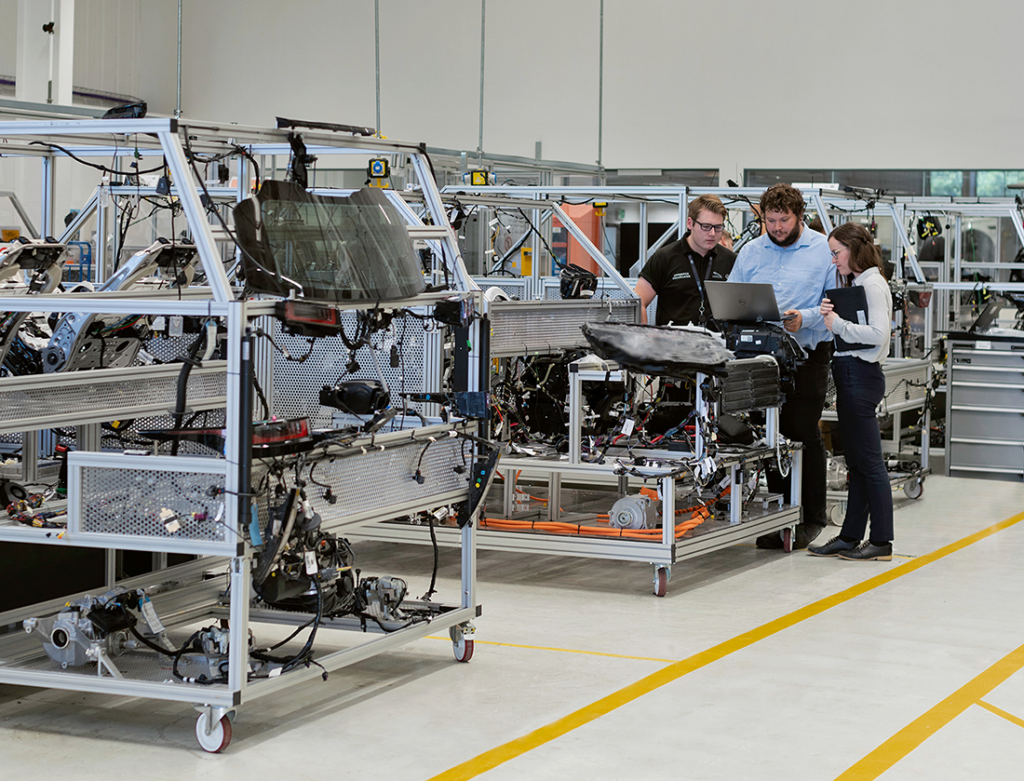
I recently had the opportunity to take a private tour of an industrial equipment company that serves the automotive and manufactured goods industries. It employs 750 people and generates 100 million Euros in revenues. In many ways, it is a typical European industrial Medium Sized Business (MSB). However, it is one of only a few fully vertically integrated businesses of its kind – from components to packaging design, from cables and sheet metal piece manufacturing to whole product assembly. It’s an impressive operation.
Impressive as it was, I found myself focusing on something else. I wasn’t distracted, but my concentration focused less on what they produce than on what they do not produce: data. I could see latent data everywhere. Data that is highly useful yet rarely collected, and even more rarely used. Seeing data led to seeing solutions. I imagined in-equipment data collecting that would allow for predictive maintenance. Beyond the factory floor, I had visions of pooling anonymized usage data that would help all actors in the industry contribute to improving products. I saw the potential for leveraging data across client groups in a way that would help them improve their processes.
While ideas are free, data collecting equipment and skilled personnel come at a cost, and the skills required can be hard to find. So, where can such a company find the relevant skills and experience needed to invest in data collection and harvesting? Because skills and experience are crucial to developing this extraordinary source of value. And where do MSBs find people with the know-how to identify appropriate areas for AI-enabled innovation? To answer these questions, it helps to understand what distinguishes MSBs from other businesses.
The entrepreneurial nature of MSBs
MSBs are different from large corporations on many levels. At the risk of oversimplifying, one could argue that MSBs leadership is entrepreneurial in nature, as opposed to managerial. This is an important factor because it can lead to resistance to change, especially when it comes to core business activities.
To be convinced to invest in AI, MSB management needs to see clear and timely returns on investment. That kind of convincing comes easier to AI practitioners who have experience in the MSB’s core industry and speak the same language.
Quite unsurprisingly, funding can also be an issue. Capital is not always readily available in amounts that would support core transformation with artificial intelligence. This means they have to look elsewhere. More on that later.
Access to data plays a role in every sector and at every level. In industrial MSBs in businesses like energy, manufacturing, pharmaceuticals, food and beverage production, construction, transportation, etc., data isn’t readily available the way it is in more dematerialized businesses such as trade or finance. In industries that are not driven by data, it needs to be produced, harvested, and normalized before it can be used for machine learning or other AI techniques. Besides, the data a company needs to harvest depends heavily on what the AI technology will do. The Economist recently published a piece that highlights this issue.
An acute skills shortage could hinder AI adoption
SMBs often find it very difficult to attract the high-level skills that they need to achieve their core business AI transformation. This is mostly because the relevant talent is scarce, and the job market for AI practitioners is highly competitive. A recent study shows that there are only 300,000 AI practitioners worldwide, and half of them are still undergoing training. A different study found that there are only 25,000 AI PhDs worldwide, with 1,500 in France, one of the most prolific AI education nations in Europe.
In a recent article about AI funding for Mid-Sized Businesses, or MSBs, I pointed out that there will soon be an acute shortage of AI skills on the market. The biggest shortage will be in deep, multi-disciplinary, and technological skills that businesses need to tackle difficult industrial problems. To quote the McKinsey Institute in 2018: “The biggest pain for artificial intelligence in the coming ten years is the need for business executives in low-tech companies to access AI in an easy-to-use way that still gives them customized solutions from well-known or tier-1 technologies and the world’s best AI talent.”
Public funding: understandably far-sighted
The EU and European governments have recognized that AI is strategically important for the European economy to remain competitive. That is why they have committed billions in funding to boost and sustain their economies’ AI transformation. Despite that, there is a significant gap in these measures that results in MSBs being poorly covered and at risk of losing their competitiveness. I see several reasons for this:
- Policymakers, by nature and culture, think of “macro” solutions that leverage and maximize invested capital’s efficiency.
- The MSB fabric’s structure does not enable efficient institutional and government lobbying, and that undermines good information sharing and communication.
- MSB activities are highly diversified and quite fragmented, serving every nook and cranny of the economy. This makes it hard for policymakers to devise anything but super-generic support policies.
In this context, finding external funding for MSBs’ AI transformation is complex:
- Venture capital funding for AI bypasses MSB AI transformation. It is mostly designed for product and platform start-ups, whose businesses are designed around the return objectives of venture capital investment.
- National or EU-level programs are designed for large corporations, whether in the form of subsidies or economic incentives. The assumption is that the industrial ecosystems organized behind these large corporations will ultimately benefit from the subsidies and incentives through a top-down AI transformation strategy that corporations initiate.
- Public AI funds mostly go to public R&D labs that work on pluri-annual, long-term projects. Sometimes they work with corporations, and occasionally with startups. More often than not, they aim to further AI science broadly. They do not focus on solving or improving precise, industry-specific problems, something that would help MSBs. High-level R&D programs tend to favour large-scale, long-term, and often transnational industrial corporations. Those actors tend to do very little to fertilize the economy beyond integrated, vertical ecosystems such as defence and, to some extent, energy.
Over the past few years, Europe has given birth to several “applied” research institutes that work on AI. However, they basically bypass MSBs because few can afford to invest resources in the cooperation structure mandated by these research institutes. They are publicly financed institutes but often built on a hybrid model of a private-public partnership (PPP). Companies wishing to engage in R&D projects with such institutes need to be able to commit important financial and human capital resources. Then and only then are the private funds matched by public funding.
Time is also an issue. R&D institutes usually focus their work over long periods, whereas MSB time constraints run considerably shorter. Their time horizons are usually shorter than the time it takes for institutional R&D projects to be committed and funded, so before any work actually gets started.
European MSBs need private applied AI R&D labs
MSBs need a new kind of partner if they are going to successfully transform their core business in a way that incorporates AI. They need accessible actors that can bridge the gap between public research and the industries they operate in. This means deep, state-of-the-art, multidisciplinary AI skills. It also requires experience in applying AI to industrial solutions. Needless to say, this all has to happen in a collaborative model that is flexible, reactive, and affordable.
Actors must meet five requirements:
- Have the expertise to tackle the data production and harvesting challenges that MSBs face when they consider implementing an AI solution;
- Understand AI data quality and production-level performance, not just scientific proofs-of-concept;
- Be experienced in the MSB’s target industry sector;
- Be able and willing to engage in project timelines and revenue models that are compatible with MSBs culture and resources;
- Passionately embrace the ethical and trustworthy implementation of AI, and be just as passionate about educating their clients to do the same over time.
In short, only private applied AI R&D labs meet these requirements. Since they are small to medium in size, these labs should:
- Have leadership that understands MSB leadership;
- Be attractive to AI PhDs and vertical industry experts alike by offering competitive salaries;
- Be spread across EU territories so that they match regional MSB dissemination.
How policymakers can make private applied AI R&D labs happen
The OECD’s AI Principles 2.1, 2.2, and 2.3 encourage policymakers to actively support people who want to create these kinds of labs, both at EU and national government levels.
Policymakers can design policies to help these labs financially and ease the bootstrap or initial phase (seed funding, sponsored hiring programs out of university labs, R&D residency grants, for instance). They can also deploy proactive marketing strategies that connect labs with MSBs across regions (exceptions to public R&D contests entry rules, sponsored no-cost invitations to events, mentions in public communications, etc.).
Some policymakers already acknowledge this and have started taking action. Programmes such as InvestEU and Horizon Europe have identified MSB innovation and digital transformation as key priorities and allocating significant funds to this segment of the European economy.
To be efficient, European governments that implement these programmes must avoid the pitfalls of previous programs. Government administrations need to identify precisely the mechanisms and players at the regional level that will leverage these funds for the benefit of European MSBs.
A bright future ahead
It shouldn’t be surprising to hear that the entrepreneur in me could not see the opportunity for private applied AI R&D labs in Europe without starting one. Seeing this opportunity to make a difference in a somewhat AI policy-forsaken part of the EU economy is what led me to move back from Silicon Valley in 2019 and start my Paris-based lab, www.indust.ai.
Within a few months, my co-founders and I assembled a strong team with multiple PhDs (from Oxford, McGill, Ecole Polytechnique), application engineers, and entered into contracts with prestigious clients. The team started publishing articles in R&D publications, just one testimony to the depth of our AI skills. We finished 92nd worldwide in Facebook’s 2020 “fake News detection” contest, which received over 2,000 qualified submissions. We were also the top-ranking private European entity.
Unfortunately, the Covid-19 pandemic has given our seed investor cold feet, and our clients have frozen AI spending. These factors have led us to the difficult decision to stop indust.ai. However, we hope to resume activity as soon as the context settles, or we can access public funding.
My main take-aways from this short indust.ai experience are that private applied AI R&D labs are needed (I cannot stress this enough), can be attractive to top AI talent if they have the right leadership team, and could benefit from public support in their impact mission.
Looking to a not so distant future, I am betting that more private applied AI R&D labs will see the light of day. That will lead to more and better competition and a more innovative and prosperous future for MSBs.