What do AI systems and cows have in common?
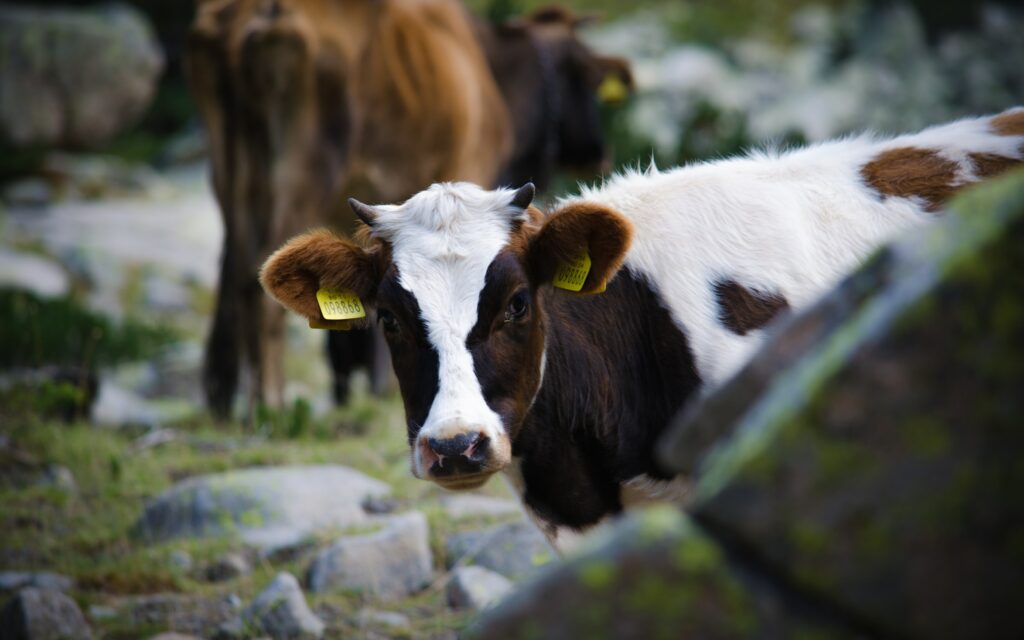
Two weeks ago, we explained what the OECD Framework for the Classification of AI Systems is, and why we need it. Last week, we launched the framework during the OECD’s AI-WIPS Conference. As the project’s leaders, we took on the task of explaining the framework in depth. We were lucky enough to have external partners offer their perspective and provide us with feedback after applying the framework to the United Kingdom’s healthcare industry. That intervention will be the subject of a future blog post, but here, we will sum up what was discussed during the first part of this conference session.
As a reminder, the OECD Framework for the Classification of AI Systems helps policy makers assess and classify different types of AI systems according to the impact they have on the policy areas covered in the OECD AI Principles, including human rights, bias, safety, and accountability. In doing so, the framework makes it easier to link AI’s technical characteristics with policy implications.
The key dimensions of the Classification Framework are linked to different stages of the AI system’s life cycle. Each dimension has its own properties and attributes or sub-dimensions relevant to assessing the policy considerations that each AI system presents. During the conference session, some of the experts who helped to create the framework spoke about its dimensions.
People in the framework
Marko Grobelnik pointed out that the People & Planet dimension is at the centre of the framework thanks to the feedback received from the public consultation conducted in June 2021, when most contributors suggested it be a more explicit focal point.
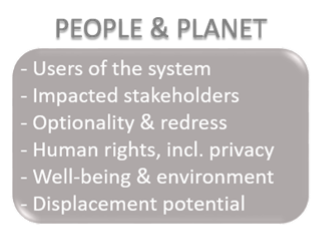
People are users and stakeholders of AI systems, knowingly or unknowingly. Naturally, people have a direct influence on all of the framework’s dimensions – they design AI systems, collect data, tune models and take actions based on AI predictions. People & Planet focuses on human rights, well-being, the environment and the world of work in considering how people use and are affected by AI systems.
How people are affected by AI systems depends largely on the type of users who interact with the systems and the users’ AI competency – from amateurs to trained practitioners like doctors and developers. This matters for many reasons, but most importantly, if something goes wrong, the right people need to be informed or asked about the situation. These individuals then need to explain and possibly be held accountable for any mistakes.
Looking to consumer groups, AI could raise product safety and consumer protection considerations. Consumers must understand their degree of choice when it comes to the outputs of AI systems that could have a negative effect on their lives, and whether they have the right to opt out.
AI outcomes also can impact human rights in areas such as criminal sentencing, where accountability and transparency are critical.
The AI system’s economic context
The economic context represents the sectorin which the system is deployed, such as healthcare, finance or manufacturing. Each will raise sector-specific considerations such as patient data privacy in healthcare, safety considerations in transportation, or transparency and accountability in public service areas such as law enforcement.
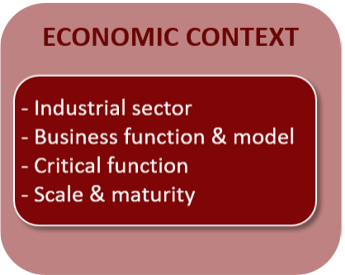
Business function and model is also a criterion: is the system for-profit, non-profit or public service? A very important criterion is the critical (or noncritical)nature of the system: would a disruption of the system affect essential services like energy infrastructure? It is also important to know how widely the system is deployed: is it just a pilot or a system deployed across an entire industry?
The AI model
The model is the core of the AI system. It is the computational representation of the AI system’s external environment. What processes, ideas, or interactions take place in that environment?
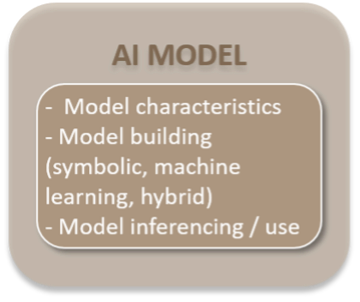
As with machine learning, the type of AI model can be statistical and can evolve over time. It can also be symbolic, or hybrid. How the model is trained or optimized, using data or expert knowledge, is an important factor. And of course, how the model is used is a very important consideration, including its objectives and performance measures.
Key properties of AI models like explainability, robustness, and possible biases depend on the type of model and how the model is built and used. For example, systems using neural networks are often deemed more accurate but less explainable. And understanding how a model was developed and is maintained is critical for assigning roles and responsibilities in risk management processes.
AI system inputs and outputs
During the conference session to launch the Classification Framework, Dewey Murdick led the discussion about the Data & Input and the Task & Output dimensions using an analogy to compare AI systems and a herd of livestock that requires care and feeding (data and inputs) to produce milk or wool (tasks and outputs).
AI systems require quite a lot of sustenance, and it is important to know what kind of food, or data, an AI system consumes. Determining the data’s structure and format, and whether it is standardized or unstructured, is crucial to this understanding. Does the data have a lot of metadata? Does it have a lot of raw data in it? Is it static or dynamic? Is it delivered in one shipment or is there a stream of content to consume? And of course, how much and how often will the AI systems need to be properly “fed”?
When trying to keep a herd of AI systems fit, it is important to know where the data comes from. Do humans or automated sensors collect it, or both? Is it being produced by input from experts or is it derived from a mix of sources, like a credit score? Is the data collected from sources that are expressly feeding this AI system by design, or was it originally created for another purpose or synthetic? Furthermore, what is the quality of the “food” that is being collected? Is it appropriate for the intended use, is it representative, of adequate size, complete, or is the data proprietary or public? The framework characterizes all these factors and more.
Next, consider the outputs of AI systems. Are the cows producing milk and the sheep producing wool? In an AI system the framework starts by asking about core applications domains, such as human language technologies, computer vision, robotics, automation or optimisation. The framework explores the tasks that the AI systems are expected to perform and with what level of action autonomy. Is the system doing goal-driven optimisation or some other task that involves speed and no direct human involvement (high-action autonomy), does a human have the ability to stop an action (medium), is human agreement required (low), or is human action required (none)?
It is worth noting that like livestock, the AI systems need evaluation to check their health. If there is an accepted industry standard for performing this evaluation, it gets noted in the framework. However, if the evaluation regime is task or context-specific, or if there are no standard methods for evaluation, then that gets noted.
Dr. Murdick noted that the OECD Classification Framework underwent evaluation during its development to make sure that nonexperts could use it. The evaluations demonstrated that the framework was much more effective for specific AI system applications than general ones. For example, the classification of a general facial recognition capability on its own is much less useful than evaluating a system that includes specific information about how an AI system will be deployed, which increases the classification framework’s usefulness.
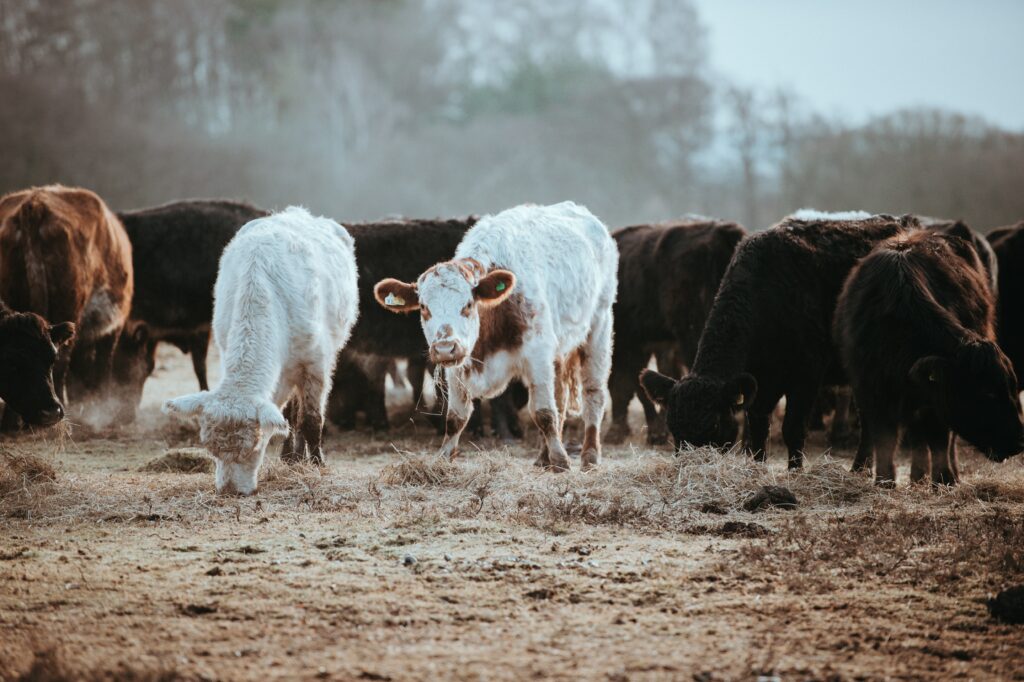
Identifying risks in data collection for better and more transparent systems
Imagine stepping back and looking at your herd of AI/ML systems. The policy maker, regulator, or corporation-turned-farmer can now see where the systems are operating and how they are being fed and exploited.
Let’s say that some cattle are grazing too far away in the pasture for human oversight, as can happen. On its own, this portion of the herd is being fed with automated and highly structured data sources, has high-action autonomy, and is performing forecasting tasks, which are notoriously hard for a data-driven AI systems. Or, consider the portion of your AI system herd that has the biggest privacy implications, or has the most external dependencies due to their use of external and found sources of data, and therefore would need more oversight.
This newly released classification framework offers a very useful approach to characterizing AI systems, especially when executed over larger sets of AI systems. Overtime, the classification framework will be refined and demonstrate its utility. The weak signal risks associated with AI systems will become more obvious across sets of AI systems. This is important for data governance and risk identification, both from a competitive perspective and to ensure that a system does not go off the rails or run off of your metaphorical pasture land.