Is it enough to audit recruitment algorithms for bias?
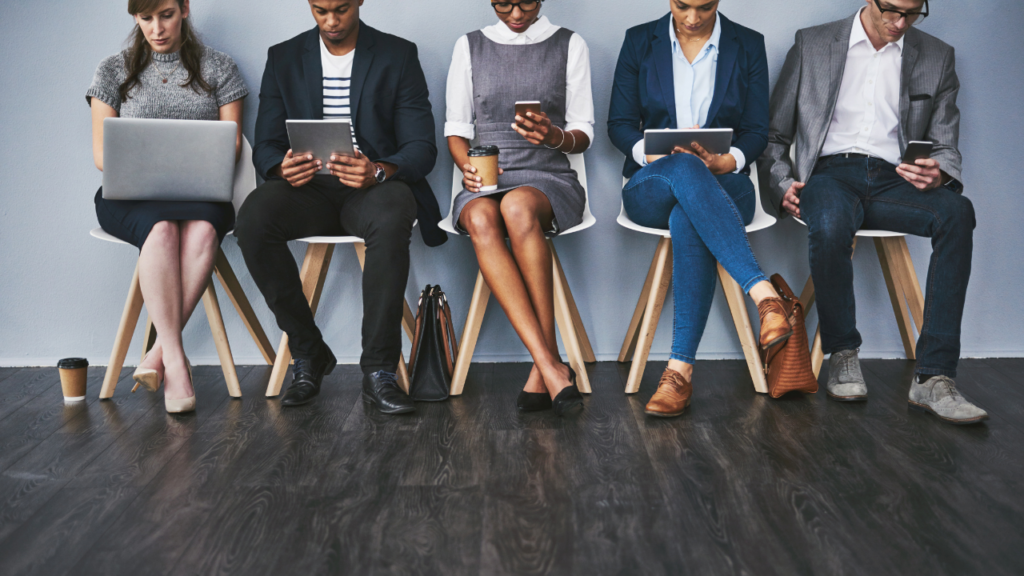
Recent years have seen much progress thanks to an exponential increase in the use of algorithms but risks also come with the benefits of this radical transformation. In particular, we have witnessed high-profile examples of harm with respect to how AI systems perform across demographics. One example of this is racist profiling by policing algorithms.
In the human resources and recruitment context, problems stemming from AI bias have sparked initiatives to ensure that AI systems used to evaluate candidates are trustworthy. For example, Amazon’s AI-driven resume screening tool caused an uproar when it was found to be biased against women, prompting Amazon to take the system off the market. Similarly, LinkedIn’s algorithm for displaying job adverts showed bias against women, resulting in the introduction of an additional algorithm to balance the outcomes.
These concerns spurred an active debate about the challenge of ethics in AI. Local and international efforts led by government, industry, academia, and civil society have produced principles and best practices for trustworthy AI, such as the OECD AI Principles. These initiatives have evolved and matured to the point where policy makers are now taking measures to govern the use of these AI systems.
Emerging AI best practices from national strategies to local mandates
The UK has published a National AI Strategy, Transparency Standard and Roadmap to an AI Assurance Ecosystem to establish best practices for the use of AI. Other jurisdictions are currently debating legislation, including the EU AI Act, the Canadian Artificial Intelligence and Data Act as part of Bill C-27 and the US Algorithmic Accountability Act. Under these legislations, users of automated or AI-driven systems would be required to conduct impact assessments for potential risks.
More targeted pieces of legislation focus on the use of AI and automated decision systems in the context of recruitment. The Illinois Artificial Video Interview Act requires employers to notify candidates when they use AI to judge video interviews, inform the state of the characteristics to make decisions, and report the race and ethnicity of new recruits.
California has also proposed legislation to govern the use of automated decision tools in employment. Proposed amendments to its employment regulation would prohibit discrimination by these systems against protected characteristics unless it is a business necessity. The Workplace Technology Accountability Act would limit workplace monitoring and require impact assessments of any automated decision tools used in the workplace.
New York City requires bias audits for recruitment algorithms
Going further, the New York City Council passed legislation that requires mandatory bias audits of automated employment decision tools used to judge candidates residing within the city limits. Under the New York City legislation, independent and impartial auditors are required to test an automated decision system for disparate or adverse impact against a protected characteristic.
Coming into effect on 1st January 2023, the legislation requires the audit of automated decision systems used to assess candidates residing in New York City. The results must be summarised and made publicly available on the employer or employment agency’s website.
Similar to the Illinois legislation, potential employers must notify candidates that they will use an automated decision tool and which characteristics and qualifications it will use to make their decision. Employers must inform candidates at least 10 business days prior to use with penalties of up to $1,500 per violation for noncompliance. Holistic AI has created a quiz to help employers operating in New York determine if they must comply with this legislation.
The metrics to guide algorithm bias audits
While audits can use multiple metrics to determine whether an automated recruitment tool is biased, and the mandate does not specify which should be favoured, the one most widely used is the Equal Employment Opportunity Commission’s four-fifths rule. It stipulates that an adverse impact occurs if the hiring rate of the minority group is less than four-fifths (80%) of the hiring rate of the majority group.
Alternatively, bias audits of automated systems might use the two-standard deviations or Empirical Rule to examine the difference in the observed and expected pass rates of different subgroups, or Cohen’s d to examine the effect size of the standard difference in selection rates. Although not explicitly stated in the legislation, tools that violate the chosen test for adverse impact will need to be redeveloped to mitigate the identified bias before they can legally be deployed.
While the scope of New York’s mandate is within the city limits, the impact that it will have is likely to be much wider and we are likely to see employers voluntarily opting to commission bias audits, even if they are not hiring candidates from New York City. The legislation is expected to have a major impact on the AI ethics and HR ecosystems and to trigger other similar legislation in other jurisdictions.
Bias audits, yes, but what about transparency, safety and privacy?
Although the New York City Council has yet to endorse a standardised approach to conducting bias audits, Holistic AI previously proposed an auditing framework for automated recruitment tools in which we suggest that bias is just one aspect of a system that can be audited. Indeed, we suggest auditing three more verticals in addition to bias:
- Transparency – This refers to governance decision-making and a system’s explainability. This includes documentation for key methods and outcomes, standardising decision-making practices, and explainability.
- Safety or robustness – This refers to a model’s accuracy when applied to unseen data, or applicants other than the ones the model was trained on.
- Privacy – This is about the risk of data leakage and minimisation principles to protect sensitive data.
Employers using automated employment decision tools can, therefore, voluntarily choose to extend the audit that they commission to include one or more of these additional verticals. This would result in greater assurance of the system, and consequently greater trust towards its use.
Why do we need transparency?
When audits assess the transparency of a system, there are two key areas that they can focus on: how well the decision-making process can be explained to relevant stakeholders (explainability), and whether the capabilities and purposes of a system are communicated and users are explicitly told that they will be interacting with an automated decision-maker (communication).
Although they are not required as part of the bias audits, the NYC mandate does touch on these elements of transparency by requiring employers to inform candidates that an automated tool will be used to assess their application and the characteristics that the tool will consider. This is requirement is echoed in the Illinois legislation. Providing this information to candidates allows them to give more informed consent for interacting with and being judged by the automated decision tool, particularly if this information is given in advance.
One study that found that the background items and attire worn during video interviews affect the personality score computed by the algorithm shows the importance of examining an AI system’s transparency. In this example, even if candidates were informed about the features used to make the personality score judgements, the system could still lack transparency since it is unclear why attire and background affect personality scores. This is often the case with black box models, where the inner workings of the model are either not known or are uninterpretable, which is the case for many automated decision tools. Therefore, auditing for transparency should help to ensure that appropriate documentation procedures, justified decision-making, and communication system specifications make it to all relevant stakeholders.
What about robustness?
Audits for robustness are important to ensure that a system has appropriate measures in place to prevent hacking and malicious use of a system as much as possible and that the system is reliable, particularly when applied to datasets other than the ones used for training. A system should work beyond the training data and perform well for different subgroups to be considered robust.
Again, there are high-profile examples that highlight the need to include robustness in audits. For example, the Gender Shades project found that gender recognition tools are the most accurate for lighter-skinned males, and the least accurate for darker-skinned females since the models were trained on an unrepresentative dataset and did not generalise well to unseen data. Amazon’s scrapped tool mentioned previously is also an example of limited robustness since the algorithm was biased against females since it was trained on a predominantly male dataset and consequently lacked robustness when applied to female applicants.
Robustness audits would also cover comprehensive safeguards against hacking and malicious use. Specifically, auditors can examine whether there are measures to monitor and track usage and if there are mechanisms in place to mitigate any risks, including unanticipated ones. Robustness or safety audits can also assess the representativeness of the datasets used to train decision systems, and whether there are rigorous procedures to assess model performance on appropriate test data.
How can audits help protect users’ privacy?
Public and political demand for the respect of personal information is what drives the need for privacy audits. While there are specific rules and regulations for protecting consumers’ privacy, such as GDPR, algorithms present unique challenges that need to be assessed and addressed accordingly. A key component of protecting the privacy of users is informed consent for the use and storage of data by the system, not just consent for interacting with the system. Privacy audits can also assess the data stewardship practices associated with the system, including the data management processes associated with the collection, pre-processing, tracking, and analysis of data and whether these processes appropriately anonymise and secure all data used by the system.
In addition, privacy audits can consider the data minimisation principles used in relation to the system. Specifically, to protect users’ privacy, systems should collect and use as little personal data as possible to meet the intended purpose of a given AI system. There are three dimensions to this that can be examined during an audit:
- adequacy of the data for meeting a specific purpose;
- relevancy of the data for the specific purpose of the system; and
- how necessary the data is – whether the data is limited and does not exceed what is necessary for the specific system purpose.
Conducting audits of these practices can protect users from malicious use of their data, allow them to make more informed decisions about how their data is used, and minimise the risk of data breaches.