From myth to metrics: Communities can leverage the real costs of generative AI when negotiating for data centres
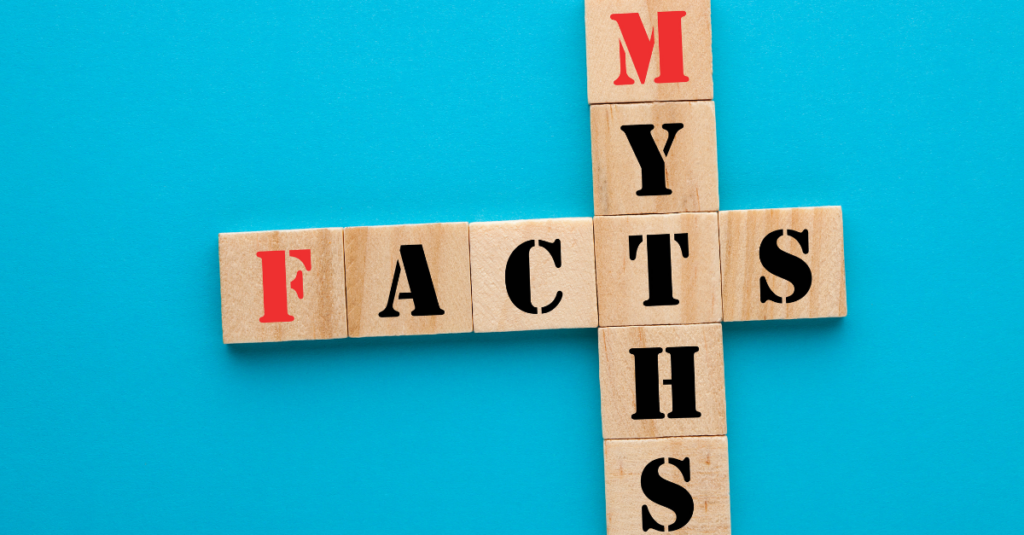
Accurate forecasts of power consumption for AI should be available following OECD AI Principle 1.1: “Inclusive growth, sustainable development, and well-being.” However, these details are often closely guarded as corporate trade secrets.
Due to the difficulty in obtaining details, analysts have been compelled to rely on outdated and incomplete sources. For instance, Goldman Sachs, the Washington Post, and the International Energy Agency have occasionally used a rough 10x power estimate for so-called ‘generative AI.’ This estimate suggests that ‘generative AI’ requires more or less 10 times the cost, electrical power, or computational performance of traditional AI or machine learning.
I refer to this as the 10x Myth, in which neither ‘cost’ nor ‘power’ is defined precisely. As the name implies, the 10x Myth is unlikely to accurately represent the actual power costs for the entire lifecycle of the AI software or significant water use and pollution costs.
The good news is that more recent data sources use metrics that are more significant on a larger scale across industries and regions. For instance, the IEA Electricity 2025 and Energy and AI reports avoid the 10x Myth. Equipped with better and more pertinent information, certain state and local communities may now have more leverage than ever—at least in the short term—in the intense competition for high-performance AI. They can leverage this position to set pricing for power and water consumption for AI and to demand concessions that help reduce costs for the public. In the realm of AI, knowledge of power—i.e., energy, water, and pollution—is power.
The 10x Myth and the importance of checking sources
Here is one use of the 10x Myth by Goldman Sachs: “On average, a ChatGPT query needs nearly 10 times as much electricity to process as a Google search.”
The 10x Myth can be traced back to a Reuters news report from February 2023: “In an interview, Alphabet’s Chairman John Hennessy told Reuters that engaging with AI, known as a large language model, likely costs 10 times more than a standard keyword search, though fine-tuning will help reduce the expense quickly.” Earlier in the same article, Reuters reported: “The wildly popular chatbot from OpenAI, which can draft prose and answer search queries, has ‘eye-watering’ computing costs of a couple or more cents per conversation, the startup’s Chief Executive Sam Altman has said on Twitter.”
It is difficult to know precisely what anyone quoted in the article meant by ‘cost’ or ‘computing costs.’ On its face, the Reuters interview was not well-suited for rigorous calculations. Nevertheless, likely due to the challenge of finding accurate sources, in October 2023, a Ph.D. candidate at the VU Amsterdam School of Business and Economics provided a rough estimate of power use based on the Reuters interview as follows: “Alphabet’s chairman indicated in February 2023 that interacting with a Large Language Model (LLM) could ‘likely cost 10 times more than a standard keyword search.’ A standard Google search reportedly uses 0.3 Wh [Watt-hours] of electricity, suggesting an electricity consumption of approximately 3 Wh per LLM interaction.” To his credit, De Vries cited his sources, the February 2023 Reuters interview and a 2009 Google blog post. Neither source was particularly robust, but he used what he had.
Like the myth that people use only 10% of their brains, the 10x Myth appears to have gained force by virtue of repetition and the ‘illusory truth effect.’ The 10x Myth appeared again in May of 2024, repeated by Goldman Sachs. In June of 2024, the Washington Post repeated the 10x Myth. The 10x Myth has spread widely. Everyone must be alert for the 10x Myth in any analysis of ‘generative AI.’
How to define ‘generative AI’?
The second foundational problem with the 10x Myth is that the term ‘generative AI’ lacks a rigorous definition. ‘Generative AI’ may refer to a brand of AI software (such as ‘ChatGPT’) or certain types of models (‘Large Language Model,’ ‘Large Reasoning Model’). Given this definitional problem and other challenges, the electricity use for a particular brand or type of AI for an ‘average’ consumer’s use might be less relevant to global power infrastructure forecasts and decision-making than data centre metrics at scale.
Time to retire the 10x Myth in the new ‘Age of Electricity’
The 10x Myth is imprecise and unreliable. Whatever ‘costs’ or ‘compute costs’ are, they should reflect the full AI lifecycle. Costs for model development should include training models to handle not only ‘average’ queries but also the most demanding queries, with 24/7 availability year-round if applicable. Costs should also include costs for water use (direct and indirect) and expenses for public health impacts of pollution.
Many AI companies will understandably continue to protect their detailed data concerning power, water, and pollution related to AI. Furthermore, it is unlikely that any new laws and regulations can be enacted and enforced effectively in the next two years. Nor should we expect lawsuits like those in Hawaii and North Carolina will be resolved quickly. Fortunately, near-term options are available to regional, state, and local communities reckoning with AI’s voracious demands.
Know your worth: Communities in desirable locations for AI data centres may have more negotiation leverage than they realise
Most countries already regulate power generation and land and water use. Armed with more accurate information about the true costs of AI data centres, communities can make better-informed decisions and seek to achieve “[i]nclusive growth, sustainable development and well-being” under OECD AI Principle 1.1. Communities may still wish to make concessions to attract AI-related investments. At a minimum, a more accurate understanding of AI data centres’ near and long-term costs can help them weigh such costs against proposed benefits and plan accordingly.
In addition, any local community needs to understand how their negotiation leverage may have changed based on the needs of high-performance AI and the growth of global power demand. In the Electricity 2025 report, the IEA predicts “[s]trong growth in electricity demand is raising the curtain on a new Age of Electricity, with consumption set to soar through 2027. Electrification of buildings, transportation and industry, combined with a growing demand for air conditioners and data centres, is ushering a shift toward a global economy with electricity at its foundations.” The IEA estimates that “a conventional data centre may be around 10-25 megawatts (MW) in size. A hyperscale, AI-focused data centre can have a capacity of 100 MW or more, consuming as much electricity annually as 100 000 households.” Reportedly, the “largest planned data centre capacity considered is 5 000 MW.” There are indications that “tech companies are asking for more computing power and more electricity than the world can provide.”
Location is a key factor in any analysis of negotiation leverage for a local community. New data centres for high-performance AI need access to massive power sources, ideally as close as possible to existing data centres filled with Graphics Processing Units (GPUs). As the New York Times reported: “Tech companies are now packing GPUs, which are ideal for running the calculations that power AI, as tightly as possible into specialised computers. The result is a new kind of supercomputer — a collection of up to 100,000 chips wired together in buildings known as data centres to hammer away at making powerful AI systems.” The global data centre map and other tools show existing locations. It is worth noting that data centres in secondary and emerging markets are increasingly in demand because primary markets are overwhelmed.
Data centres and water
Data centres for AI need a lot of water, ideally freshwater. A recent report from March 2025 found that one particular “series of models . . . consumed 2.769 million litres of water, equivalent to about 24.5 years of water usage by a person in the United States, even though [the] data centre is extremely water-efficient.”
Public health costs from AI pollution are also significant. Researchers have estimated that: “The total public health burden of U.S. data centres in 2030 is valued at up to more than $20 billion per year . . . .” Even a 72% increase in tax rates on data centres, such as that imposed in Northern Virginia, may not cover such costs.
Depending on negotiation leverage, the transparency of rate-setting proceedings, and local needs, additional criteria from a local community for new or expanded AI data centres and their associated power and water demands may include:
- funding for community power microgrids that allow rural areas and critical infrastructure to switch between grid-connected and stand-alone operation;
- freshwater replenishment (for direct use and indirect use);
- use of reclaimed wastewater for cooling data centres and requirements for river restoration;
- jobs (not only during construction), trade and technology training, schools;
- healthcare facilities and programme funding; and
- funding for parks and recreation, farming, public lands and wildlife preservation.
According to Microsoft, ChatGPT was made “next to the cornfields west of Des Moines [, Iowa].” Shortly thereafter, OpenAI was valued at US$157 billion. Now, “OpenAI hopes to raise hundreds of billions of dollars to construct computer chip factories in the Middle East. Google and Amazon recently struck deals to build and deploy a new generation of nuclear reactors. And they want to do it fast.”
Given the stakes, knowledge of power, water, and pollution is power.