Ensuring trustworthy algorithmic decision-making
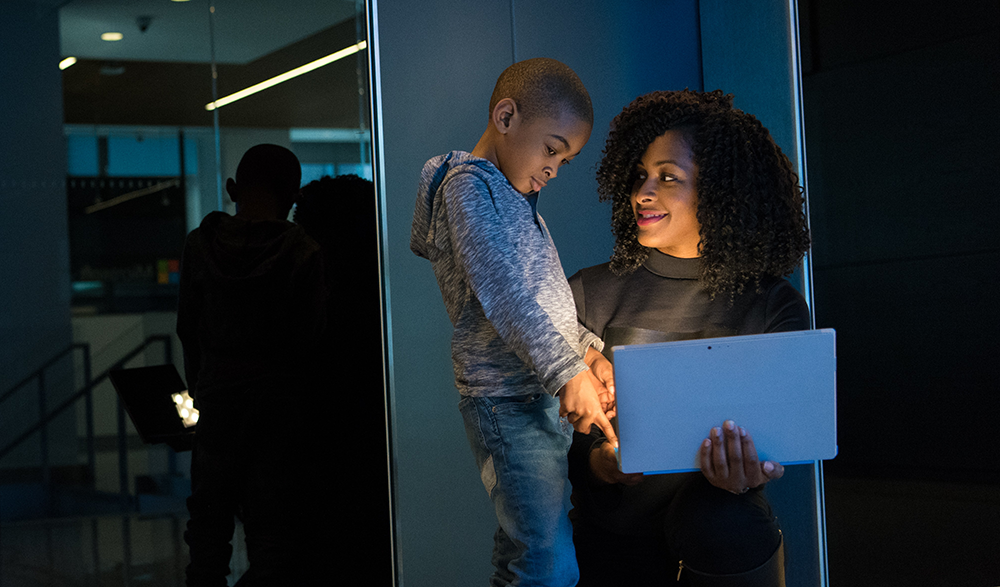
Can algorithms help us make fair decisions?
Decision-making is currently going through a period of change. The use of data and automation has existed in some sectors for many years, but it is currently expanding rapidly due to an explosion in the volumes of available data, and the increasing sophistication and accessibility of machine learning algorithms.
Growth in algorithmic decision-making has been accompanied by significant concerns about bias. Left unchecked, algorithms have the potential to skew decision-making and lead to unfair outcomes. We have seen many examples of algorithms amplifying historic biases, or creating them anew. In the US, for example, an algorithm used to predict the likelihood of a criminal reoffending was shown to have a bias against black defendants: white defendants were more likely to be incorrectly judged as low risk, and black defendants more likely to be incorrectly judged by the algorithm as high risk.
We must and can do better. Fair and unbiased decisions are not only good for the individuals involved, but they are also good for business and society. Successful and sustainable innovation is dependent on building and maintaining public trust.
Good use of data also presents us with an opportunity to enhance fairness. It can serve as a powerful tool that can enable us to see where bias is occurring and measure whether our efforts to combat it are effective. If an organisation has hard data about differences in how it treats people, it can build insight into what is driving those differences, and seek to address them.
The CDEI’s review into bias in algorithmic decision-making
At the Centre for Data Ethics and Innovation (CDEI), we recently published our review into bias in algorithmic decision-making.
The review focused on the use of algorithms in significant decisions about individuals, looking across four sectors: recruitment, financial services, policing, and local government. We found underlying challenges across these four sectors, and indeed other sectors where algorithmic decision-making is happening. We explore three approaches to addressing these challenges:
- The enablers needed by organisations building and deploying algorithmic decision-making tools to help them do this in a fair way.
- The regulatory levers, both formal and informal, needed to incentivise organisations to do this, and create a level playing field for responsible innovation.
- How the public sector, as a major developer and user of data-driven technology, can show leadership in this area through transparency.
We made cross-cutting recommendations for government, regulators, and industry, which aim to help build the right systems so that algorithms improve, rather than worsen, decision-making. While our review focused on the UK context, we believe that many of the findings can be applied in other OECD countries.
What can organisations do?
The review focuses primarily on the UK government’s role and regulators, but individual organisations can and should address algorithmic bias. Senior decision-makers in organisations need to understand and engage with the trade-offs inherent in introducing an algorithm. They should expect and demand sufficient explainability. If they know how an algorithm works, they can make informed decisions to balance risks and opportunities as they deploy it into a decision-making process. Organisations are accountable for their decisions, whether they came from a team of humans or an algorithm.
Decisions about what fairness means are too important to be left to data science teams to make in isolation. Organisations also need to understand how different definitions of fairness might be relevant to their context and institutional goals so that they can use them to detect and mitigate bias. As part of our research on approaches to bias detection and mitigation, we worked with a partner to build a web application that seeks to explain the complex trade-offs between different approaches. However, there is still more to be done to build an understanding of which approaches are best in which contexts.
Organisations often find it challenging to develop the capacity to understand and address bias in a data-driven world. The truth is that organisations need people with a wide range of skills to navigate between the analytical techniques that expose bias and the ethical and legal considerations that inform the best responses. Some organisations may be able to handle this internally, others will want to call on external experts to advise them. Seeing an emerging demand, the CDEI is also looking at the growing ecosystem of AI assurance tools and services. We hope to bring together all the relevant players in the field to help build consensus on how the ecosystem can best develop to support responsible innovation.
The role of transparency in trustworthy AI
For innovation to be sustainable there needs to be a sufficient level of public trust. There is clearly some way to go to build public trust in algorithms, and the obvious starting point for this is to ensure that algorithms are trustworthy.
While this is true in all sectors, the public sector has a particular responsibility to set an example and show what good transparency in the use of algorithms should look like. After all, decisions that come from the public sector often have a considerable impact on peoples’ lives. Additionally, while an individual can opt out of using a commercial service whose approach to data they do not agree with, they do not have the same option with essential services that the state provides.
Fortunately, the UK government has put mechanisms in place for transparency in decision-making and technology. The government has increased transparency in the use of technology with its design principles and declared that “making things open makes them better”. Significant information about human-driven decision-making processes is also published (and interested parties can ask for further information under the Freedom of Information Act and Data Protection Act).
Still, there is not a consistent approach to transparency for algorithmic decision-making. The CDEI believes that the UK government should make transparency mandatory for all public sector organisations that use algorithms to make decisions that have a significant impact on individuals’ lives. The CDEI’s final report suggests specific definitions for these terms.
Deciding how much and what kind of information to share about the use of algorithmic tools can sometimes be tricky. It is crucial to strike the right balance between ensuring that organisations are providing intelligible information and not creating greater concern by sharing information that is incomplete or too complex. We suggest the scope of information should include:
- Details of the decision-making process in which an algorithm/model is used.
- A description of how the process used the algorithm/model, including the level of human oversight.
- An overview of the algorithm/model itself and how it was developed, covering for example: the type of machine learning technique used to generate the model, a description of the data on which it was trained, an assessment of the known limitations of the data and any steps taken to address or mitigate them.
- An explanation of why the overall decision-making process was designed in this way, including impact assessments covering data protection, equalities, human rights, and relevant legislation.
A few cities have already begun to experiment with approaches to increase transparency, including Amsterdam, Helsinki and New York. Some thought still needs to go into how this could work across the UK’s public sector. To move this forward, the CDEI is currently working with the UK’s Central Digital and Data Office (CDDO) as it seeks to develop an approach to algorithmic transparency.
A sectoral approach to regulation
In many countries, including the UK and US, there have been calls for new cross-cutting AI regulation. To heed these calls in our review, we spoke to a large number of people with varied and opposing views on this issue.
Algorithmic bias does introduce new interactions between discrimination law, data protection law and sector regulations. This creates a need for careful thought about legal compliance in an algorithmic age. We concluded, however, that the current UK legal framework can be used to incentivise fairness in algorithmic decision-making, and the focus for now should be on how to make existing legislation work.
There are a number of reasons for this. Many decision-making processes, at least for the significant decisions which we focused on in our review, combine elements of algorithms and human judgement. Different organisations might seek to balance these in different ways, and there is no easy answer on the right approach. What matters to the individual is the overall decision – whether they have been fairly treated in the process, and whether the outcome is fair. Although the mechanisms might be different, we feel that we should be applying consistent standards to fairness in decision-making across human and algorithmic elements of decisions, and this is potentially quite hard to achieve once we step into algorithm-specific legislation.
Separately, and from a practical perspective, there is plenty of work to be done in the UK and elsewhere to build a common understanding of how existing laws should be applied to algorithms. Focusing on that will achieve progress more quickly than trying to design new legal approaches. The first step is to clarify current legal interpretations, including the Equality Act of 2010. This would give certainty to organisations deploying algorithms, as well as ensure that existing individual rights are not eroded, and wider equality duties are met.
Concrete examples of areas where more clarity would be useful abound. Organisations need clearer guidance to interpret indirect discrimination in an algorithmic context, what level of testing for bias is appropriate, how much demographic data should be collected to monitor outcomes, and what mitigations are appropriate. Some of this can be cross-cutting, but much of it will need to be resolved in the context of individual sectors and use-cases.
The financial services industry already has some of the regulatory arrangements in place, for example, to determine which factors can legitimately be used in a credit score or insurance decision. As the use of data and sophisticated models increases in other sectors, companies, industry bodies and sector regulators will have to take the lead in setting appropriate norms. As algorithmic decision-making grows and more norms are established, there will be a need to revisit existing legislation, which should be kept under consideration as guidance is developed and case law evolves.
Interested? Please get in touch
Enabling data to drive better, fairer, more trusted decision-making is a challenge that countries face around the world. We are looking forward to sharing and discussing our recommendations with international partners who want to ensure that data-driven technologies are adopted in a responsible and trustworthy way, particularly in the public sector. If you would like to talk to us about our work in this space, please get in touch at bias@cdei.gov.uk.