Reflections on safety: General-purpose AI developers can learn from the Challenger disaster
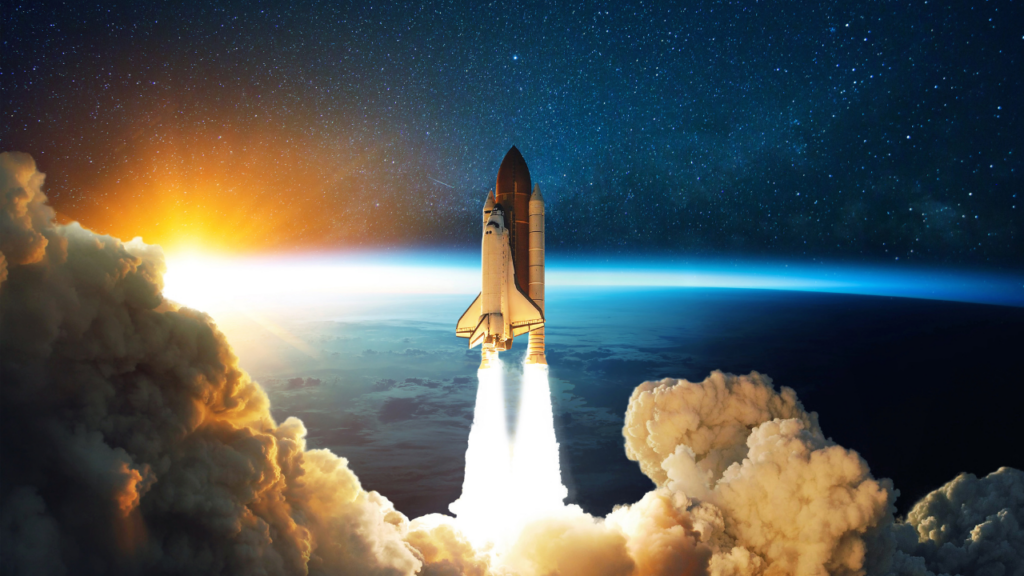
Thirty-eight years ago, on a cold January morning in Florida, the space shuttle Challenger began its final journey towards the heavens with millions of children watching at schools across the United States. Seventy-three seconds later, the orbiter was enveloped in a fireball, and it violently disintegrated, killing its crew of seven shortly thereafter.
Schools had tuned into the mission because Christa McAuliffe, from Concord, New Hampshire, was on board and poised to be the first teacher in space. Her death had an immense impact on New Hampshire, my home state, and influenced my career path. My love of space was fueled by visits to the Christa McAuliffe Planetarium in Concord when I was young. Later, I interned at NASA, became a spacecraft test engineer, and worked on ISS resupply missions.
During this time, I learned firsthand what it means for things to go catastrophically wrong on a space mission; while working on the launch console for the Orb-3 spacecraft bound for the ISS, the launch vehicle exploded shortly after liftoff. Thankfully, the mission was uncrewed, and no one on the ground was injured. However, it was a significant setback to the program, which took more than a year to return to flight, and it was a blow on a personal level to those of us who built and tested the spacecraft by hand.
After fifteen years in the space industry, I have shifted to developing risk management standards for high-risk AI systems and General-Purpose AI (GPAI) models after seeing a need for professionals experienced in risk management principles. With the recent anniversary of Challenger’s final flight, I want to take a moment to reflect on its lessons and how they can help improve the safety of GPAI model development. I’ll explore how essential it is to understand a system before its risks can be managed and how a strong safety culture is necessary for any organisation that operates near the limits of our engineering capabilities.
Lessons from the Challenger
In the wake of the disaster, the Rogers Commission was convened to investigate the cause. Notably, commissioner and Nobel Prize-winning physicist Richard Feynman’s independent investigative approach was highly irregular but yielded important results. He became strongly critical of NASA and nearly dissented from the Commission’s final report, but his conclusions were included as Appendix F – Personal Observations on Reliability of Shuttle. Here are some of his most important findings.
Maintaining schedule over safety
On a technical level, the Challenger disaster was caused by faulty design of the solid rocket boosters, specifically their O-rings. The night before the launch, the boosters’ engineers at Thiokol expressed concerns about the unprecedented freezing temperatures and recommended postponing the launch. NASA management pushed back, eventually getting the “Go” they sought from Thiokol management. Ultimately, the O-rings were too cold and rigid at launch to prevent hot exhaust from escaping and rupturing the external fuel tank.
NASA management was focused on maintaining a rapid launch schedule to justify the substantial investment in the shuttle program but this came at the cost of safety. Feynman pointed out in Appendix F, “If a reasonable launch schedule is to be maintained…subtly, and often with apparently logical arguments, the [safety] criteria are altered so that flights may still be certified in time.”
An incomplete understanding of the O-ring problem
NASA rationalized the warning sign of O-ring erosion with a model that incorrectly showed a safety factor rather than a defect. However, the Commission’s report found that a “careful analysis of the flight history of O-ring performance” was never carried out. Feynman levelled further criticism at NASA officials for not fully understanding the problem:
The acceptance and success of these flights is taken as evidence of safety. But erosion and blow-by are not what the design expected. They are warnings that something is wrong. The equipment is not operating as expected, and therefore there is a danger that it can operate with even wider deviations…The fact that this danger did not lead to a catastrophe before is no guarantee that it will not the next time, unless it is completely understood.
A poor safety culture
Though the disaster was directly caused by O-ring erosion, the commission found the true root cause was NASA’s poor safety culture. Feynman further stated that “the management of NASA exaggerates the reliability of its product, to the point of fantasy.” Appendix F compares how the managers believed the probability of losing a shuttle and its crew was 1 in 100,000, while the engineers believed it to be 1 in 100. In the end, after 135 missions and the loss of two shuttles and fourteen crew members, the final program tally was 1 in 67.5.
Feynman highlighted that there were “difficulties, near accidents, and accidents, all giving warning that the probability of flight failure was not so very small.” After the Challenger accident, the solid rocket boosters were redesigned, but NASA’s safety culture remained flawed, resulting in the loss of Columbia in 2003. Just before the Columbia disaster, NASA was studying the US Navy’s SUBSAFE quality assurance programme to improve its own mission assurance. SUBSAFE was created to ensure the integrity of submarine hulls after the loss of the USS Thresher. It is an excellent example of an organisation with a strong safety culture. Its cultural principles include a “questioning attitude”, “critical self-evaluation”, “lessons learned and continual improvement”, “continual training”, and “a management structure that provides checks and balances and assures appropriate attention to safety”. Since its founding in 1963, not one SUBSAFE-certified submarine has been lost. Unfortunately, these lessons came too late for Columbia’s final crew.
Parallels to current AI development
Feynman’s Appendix F still has great value to me in AI risk management. Its lessons are relevant to all engineering fields, and it is worthwhile reading for everyone working on GPAI models and high-risk AI systems. The above points have particular relevance to developing GPAI models.
Moving fast over risk evaluation
As with the pressure to launch Challenger, I see a similarly worrying trend in the race to train GPAI models. However, the rush to deploy the next frontier model presents risks today, which may delay the benefits that could come to pass, just as the loss of two shuttles tempered our ambitions to explore space.
In addition, billions of dollars drive the research and development of human-level AI models and systems. Still, AI safety is far less funded and is playing catch-up at every step. While recent risk management proposals from some AI labs are moving in the right direction, I fear that they assume scaling will continue even as they encroach into the territory of potentially dangerous capabilities. If we scale first and evaluate risks second, safety and risk management must be a secondary priority.
An incomplete understanding of how GPAI will work
Just as NASA did not fully understand the O-ring problem, and today’s GPAI models frequently operate unexpectedly, indicating that we do not thoroughly understand how they work. Until we do, we must expect a non-zero likelihood of serious consequences from their development and use, especially because there are already signs of trouble. And even if we take a safety-first approach with current model architectures, we still cannot rule out the possibility of grave failure or accident because reliably predicting the risks of a system requires an understanding that we do not have for GPAI models.
In light of this, AI labs and governments should invest much more in provably safe model architectures. Even if it comes at the expense of rapid AI product launches now – the investment will pay dividends and seem downright cheap in hindsight compared to the potential consequences of accelerating on the current path.
Seek out a safety culture that works
Unfortunately, even with reduced schedule pressure and a better understanding of GPAI models, there will always be uncertainty and risk. This is the nature of all complex systems. Therefore, we must learn from exemplary organisations, such as SUBSAFE, with strong safety cultures that effectively and consistently manage these risks. They put safety before schedule and other considerations for good reason;. However, no SUBSAFE-certified submarine has been lost since the program started in 1963, the USS Scorpion was lost in 1968 because it was expressly not certified due to schedule pressure and a lack of commitment to the newly established safety culture. Effective safety culture must start at the top and be adhered to consistently in both word and deed.
Even AI cannot fool nature
As we continue deeper into the uncharted territory of GPAI models, it would behove us to study lessons from the space industry and other domains where lives rely on sound engineering and dependable risk management. To begin, organisations that build and deploy GPAI models must follow schedules that make adequate room for safety, and they must have strong safety cultures. In addition, we need a better understanding of how these systems actually work. The Challenger disaster shows us what can happen if we do not heed this advice. Here again, Feynman said it best:
For a successful technology, reality must take precedence over public relations, for nature cannot be fooled.