Three takeaways from classifying AI systems designed to fight the COVID-19 pandemic
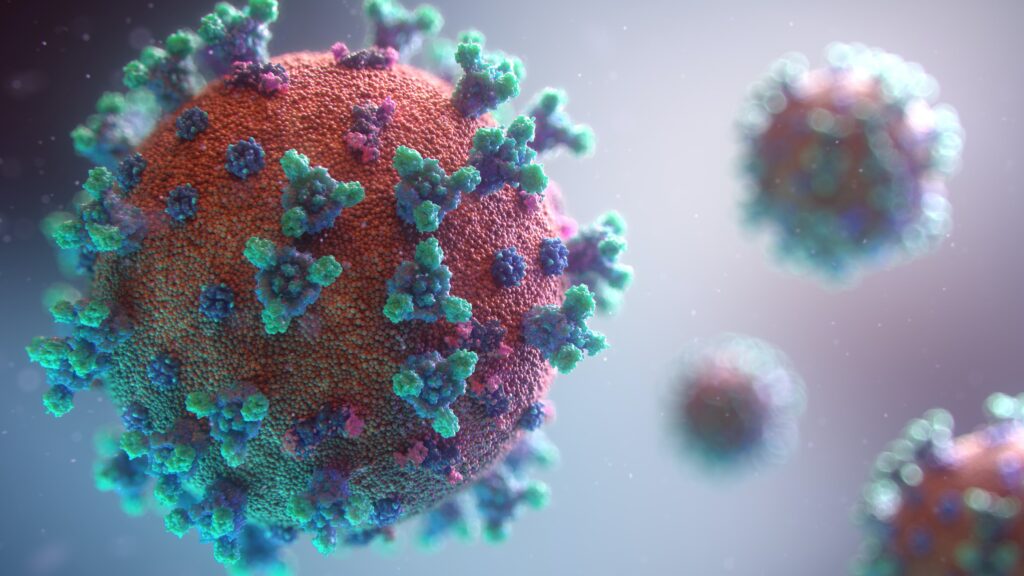
Historically, pandemics have served as catalysts for some of the most significant public health and scientific breakthroughs, including the practice of quarantining and the discovery of vaccines. But it took decades after these measures were developed for public health officials to understand and implement them correctly. In today’s interconnected world, technological discoveries can advance from development to deployment in a matter of days. The sheer breadth of information also means it can be harder to keep track of technology that exists at a given moment, particularly in times of crisis.
To better understand the AI systems developed in response to the COVID-19 pandemic, The Future Society (TFS) has worked with the Global Partnership on AI (GPAI) AI & Pandemic Response Subgroup and OECD.AI to build a “living” repository to classify these systems and assess their impact. We have a number of observations that we think are worth sharing that can contribute to the larger body of AI measurement and monitoring research.
The big picture of AI and COVID-19
AI systems can help fight diseases with their extraordinary abilities to draw inferences from data and leverage algorithms to unravel complex decisions. During the COVID-19 pandemic, developers created AI systems to assist in many public health domains, including viral biology, forecasting outbreaks, governments’ intervention strategies, and flagging misinformation, to name a few.
Some of these AI systems may play pivotal roles in resolving the COVID-19 pandemic or stunting the next large-scale pathogenic outbreak. Years from now, some of these tools could be a critical component of outbreak-response portfolios. To get to that stage, we need to develop the infrastructure for monitoring AI systems being used today, so that we better understand what they are, where they are used, and the effects they have on their environments. This will also help us assess their benefits, risks, and potential for impact.
To this end, we built a Living Repository of AI systems used in the COVID-19 pandemic response and Summaries of Top Initiatives (publication forthcoming), which covers the initiatives that the GPAI AI & Pandemic Response Subgroup identified to be the most promising in terms of their potential to scale to larger populations and to benefit from a potential partnership with GPAI.
Click on image to enlarge
The GPAI AI & Pandemic Subgroup will use these resources to guide their approach to partnerships in 2022. Beyond GPAI workstreams, we hope that this work can serve as a model for assessing the impact of AI systems designed for pandemic response or any other situation where they could be useful.
In addition to testing the OECD Framework for the Classification of AI Systems, we uncovered some challenges that can arise when attempting to classify and monitor AI systems. We hope that these takeaways will be useful to the OECD and other governmental, intergovernmental, corporate, academic, and civil society organizations’ efforts to measure and monitor AI systems.
Three takeaways from our research
#1: Classification frameworks are the bedrock of AI governance
Our last blog post discussed the role of classification frameworks in establishing a common language between industry, investors, researchers, policymakers, regulators, and laymen. Functionally, a classification framework provides an analytical foundation for—and a connective tissue between—a wide range of policy, regulatory, business, and research objectives, including:
- Standards, benchmarking, auditing, and certification, by establishing characteristics to be defined and measured
- Laws and regulations, by influencing how AI systems are characterized and differentiated in legal/regulatory texts
- Impact assessments, by influencing how AI systems and their environments are conceptualized and measured
To be effective over such a broad range of objectives, a classification framework must be built with interoperability and precision in mind. It has to strike a balance between being general enough for criteria to be applicable to any AI system, but narrow enough so that it is only applicable to technologies that fall within the definition of an “AI system.”
When using a classification framework, one must keep in mind that it serves as an entry point, not an all-encompassing tool, for policy, investment, or research objectives. It describes to some extent an AI system’s composition and function but depending on the user’s goals, it may not capture everything they wish to know about the AI system. For example, to assess the real-world impact of AI systems developed for COVID-19, our team had to develop a complementary impact-oriented framework that captured, among other details, the stakeholders involved in AI systems’ development, how the systems performed, and whether they faced any technical or non-technical barriers to implementation. The OECD Framework for the Classification of AI Systems served as a foundation for our work, by framing the dimensions—context, data, model, and function, along with their respective subdimensions—from which an AI system’s impact could be measured. However, the framework alone could not achieve our research objective.
In the coming years, we expect to see research and law-making building upon the OECD’s classification framework for a wide range of purposes. Regulators may use it, for example, to determine which characteristics make an AI system subject to a particular law or regulation, such as the EU AI Act. They may also use it to develop soft law instruments like technical standards, such as those being negotiated between the European Commission and the US Government through the Trade & Technology Council, or codes of conduct, like the AI Bill of Rights that the White House Office of Science and Technology Policy is developing.
Researchers and analysts have begun and will continue to develop complementary tools tailored to specific sectors or research objectives. Some tools will dive more deeply into dimensions that fall within the OECD’s framework, and some will explore aspects not covered by the framework. Some more narrowly-focused classification tools, such as Model Cards for Model Reporting and Datasheets for Datasets, already exist and are becoming widely used. We encourage researchers to make efforts to test, evaluate, and help standardize these tools, which will be accessible via the OECD tools catalogue launching in Fall 2022. This will require mechanisms for engineers and researchers to provide feedback on the robustness, fitness-for-purpose, and limitations of the tools that they use. The field as a whole stands to benefit from more durable AI system measurement and monitoring infrastructure as technology and value chains mature.
#2: A good measurement tool is a process, not a product
Measurement tools are socially constructed—they are the result of humans working together to define something observable in terms that are accurate, consistent, and relevant. Precisely for this reason, they are vulnerable to errors and biases: the process of selecting what properties should be measured, and how they should be measured, involves assumptions and decisions that reflect human values, which could ultimately result in misalignment between what we claim to be measuring and what the tool is truly built to measure. And because of the many ways that measurement tools influence the governance of AI systems, such a misalignment can ultimately lead to significant real-world fairness-related harms.
Measurement tools will have to be continuously tested and modified to ensure they reflect state-of-the-art technology. Otherwise, information gaps could arise from AI systems advancing in ways not captured by the measurement tools that exist. In our research, we tested the robustness of the OECD Framework for the Classification of AI Systems by applying it to the AI systems we identified in the context of pandemic response. In the process, we noted how accurately and precisely the framework’s dimensions described each AI systems’ characteristics. We found it to be reliable overall, with just a few instances where we felt there was room for improvement, and we reported those to the OECD.AI team.
The maintenance of measurement tools will be an ongoing, interdisciplinary effort that demands the active contribution of researchers and practitioners to ensure that these tools accurately describe the broad range of domains and functions for which AI systems are being developed. For example, engineers and researchers can classify the AI systems they are developing or studying. Researchers may also wish to explore whether more significant conceptual errors exist in measurement tools’ design by testing alternative measurement methods against one another; Catherine Aiken’s “Classifying AI Systems” report is an example of this approach applied to the OECD Framework for the Classification of AI Systems. By testing measurement tools from a wide variety of approaches, we optimize their fitness for purpose and reduce the likelihood that they contain errors or biases.
#3: New governance mechanisms and institutional norms are necessary to enable adequate AI system monitoring
Traditional governance approaches have been unable to keep pace with the development and deployment of AI systems. Within booming digital markets, AI developers often opt to conceal their intellectual property, leaving regulators largely unaware of existing AI systems and their value chains and therefore unequipped to regulate them effectively. Solutions are needed to reconcile diverging interests and improve our ability to govern AI systems.
In our own information-gathering efforts, we found it difficult (and at times impossible) to find all the information we wanted to know about an AI system. The type of information that would interest researchers and regulators was rarely centrally-located and exhaustive. The accessibility of these details tended to depend on their developers’ incentives. For instance, academic groups often described the technical characteristics of their AI systems in detail, but commercial enterprises seldom did so. By contrast, commercial enterprises often shared information about their tool’s deployment (sometimes through exaggerated or false claims), but academic papers rarely touched upon such details.
Without incentives for engineers to report the technical characteristics or deployment of their AI systems, it seems unlikely that this situation will improve substantially. New governance mechanisms, including hard laws, soft laws, and self-regulation, are needed to expand monitoring capacities and hold developers and operators accountable for the systems they create.
Fortunately, such mechanisms are beginning to materialize. With regards to hard law, the proposed EU AI Act, for example, would require developers of high-risk AI systems to perform ex-ante conformity assessments, which stipulate the procurement of technical documentation, quality management systems, and post-market monitoring systems. In the US, the recently-introduced Algorithmic Accountability Act of 2022 would require companies to complete impact assessments of their automated decision systems and provide summary reports to the FTC, as well as empower the FTC to establish a public repository of these systems. In regards to soft law, the IEEE Standards Association has pioneered instruments including Ethically Aligned Design (EAD) and their Ethics Certification Program for Autonomous and Intelligent Systems (ECPAIS). And in 2020, NeurIPS conference organizers demonstrated leadership in self-regulation by requiring AI researchers to consider their systems’ societal impact and to disclose their personal financial conflicts of interest. More corporate governance mechanisms are analyzed in depth in “Corporate Governance of Artificial Intelligence in the Public Interest.”
Functioning in tandem, these governance mechanisms will embed precaution and transparency in the AI development ecosystem by enabling large-scale and long-term monitoring efforts. By allowing us to track the effects that AI systems have in the real world, we will be more capable of ascertaining the properties of AI systems that tend to be safe or problematic, identifying developmental trends, and forecasting future outcomes. In the future, these data may prove instrumental in flagging AI systems with harmful outcomes that warrant swift termination or in identifying the next public health breakthrough that would serve us well to adopt.
Other contributors to this work include Adriana Bora, Rui Wang, Bruno Kunzler, Lewis Hammond, and Kristy Loke. This work was funded by GPAI and was conducted in partnership with members of the AI-powered Immediate Response to Pandemics Project Steering Group.