A first look at the OECD’s Framework for the Classification of AI Systems, designed to give policymakers clarity
Find out about the OECD’s Framework for the Classification of AI Systems and follow the next steps
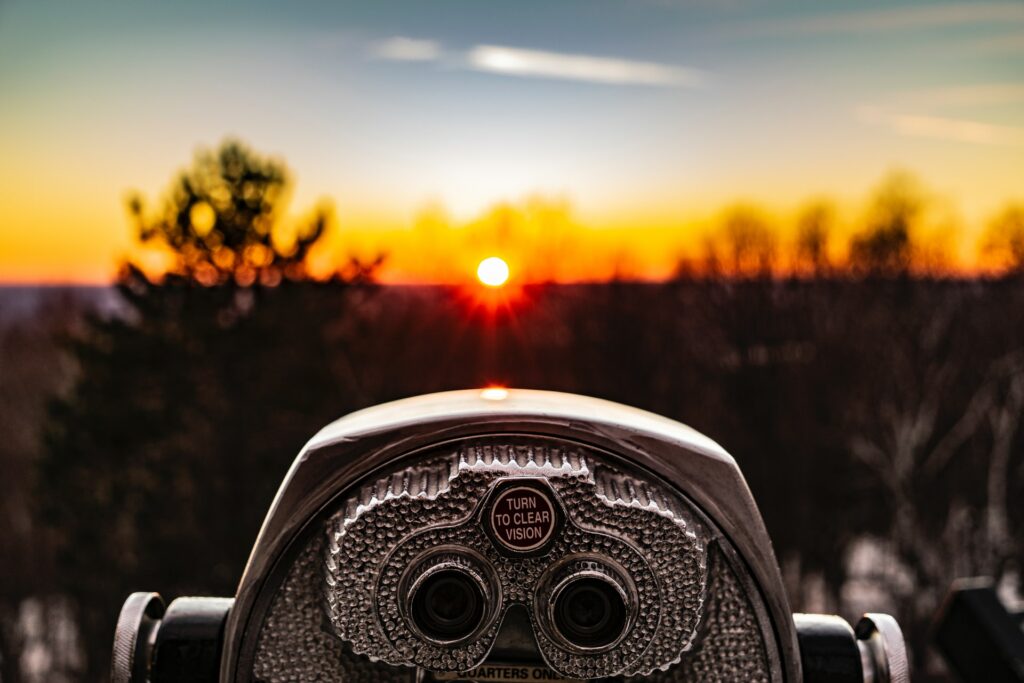
Today, AI systems are becoming more and more integrated into our daily economic life: factories are using image recognition systems to improve automated production lines, hospitals are using machine learning approaches to better understand the changing needs of their patients; scientists are using ‘deep learning’ approaches to improve COVID-19 characterization and diagnosis, and shipping companies are using AI to optimize the routes their trucks take.
Though all of these tasks involve AI-based technology, they are enabled by a wide variety of methods and data. They also exhibit a great diversity of impacts in terms of the sectors where they are deployed, the entities that deploy them, and the extent to which the AI system could have a positive (or adverse) effect on people.
That’s why at the OECD we’ve spent this year working on an AI classification framework. The purpose of this framework is to give policymakers a simple, easy to use lens through which they can view the deployment of any given AI system and better understand the particular challenges with deployment in that domain.
Watch the video: The OECD is developing a classification of AI systems
A four part classification framework
The classification system is based on the OECD’s definition of AI and divided into four dimensions:
- Context: The environment where the system is being deployed and who is deploying it.
- Data and Input: The data the system uses and the kinds of input it receives.
- AI Model: The underlying particularities that make up the AI system – is it, for instance, a neural network, or a linear model?
- Task and Output: The tasks the system performs and the outputs that make up the results of its work.
This first draft of this policymaker-friendly tool for understanding AI systems has been developed this year, using a virtual multistakeholder process that leveraged the expertise of more than 57 people in over 40 countries. The OECD’s Network of Experts spent several months developing this framework and prototyping examples. It also conducted a survey to test its effectiveness.
From a broad definition to an AI classification system
The OECD’s high-level general definition of an AI system inspired the classification framework’s structure. The definition was designed to be technology-neutral and applicable to both short and long-term time horizons. It is abstract and broad enough to encompass most of the more precise definitions that are used by the scientific, business and policy communities:
An AI system is a machine-based system that is capable of influencing the environment by making recommendations, predictions or decisions for a given set of objectives. It uses machine and/or human-based inputs/data to: i) perceive environments; ii) abstract these perceptions into models; and iii) interpret the models to formulate options for outcomes. AI systems are designed to operate with varying levels of autonomy (OECD, 2019).
This definition is essential to making the OECD AI Principles future-proof– it is a legal instrument that needs terms of reference. But when it comes to policymaking, it is not enough to have such a broad definition because it does not offer enough detail to confront the wide variety of AI systems that raise specific policy opportunities and challenges. For example, neural networks may have more issues when it comes to explainability or interpretability than, for example, Bayesian statistics-based methods.
Even systems that use the same technology can require different levels of scrutiny, simply because the decisions they make have a much greater impact on peoples’ lives. For example, the technology behind granting mortgages and loans is essentially the same as that used for suggesting movies to users, but the consequences that errors can have in the former context are much more serious than in the latter.
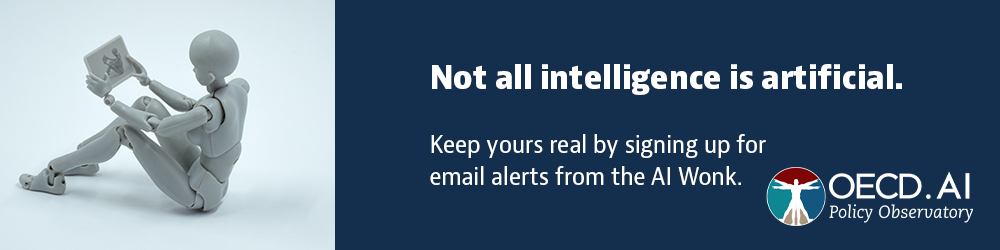
How is the AI classification framework structured?
This initial version of the classification framework is designed to help guide policymakers through the important things they need to understand to design adequate policies for each type of system.
The taxonomy is based on the simplified OECD definition and gives more detail for each dimension and sub-dimension. It takes a more detailed look at the implications for specific AI systems by focusing on the policy-relevant aspects. The classification taxonomy offers analysis for each type of system through the four main dimensions.
This will allow policymakers to review and classify AI systems according to their potential impact on public policy, particularly in areas covered by the OECD AI Principles.
Applying the AI classification framework to credit ratings
The operations behind a credit-scoring system is a good example of how the AI framework can operate in the high stake context of financial and insurance activities.
To establish the score, a machine-based system influences its context by determining whether or not people should be granted loans. Its output task is to establish a credit score for a given set of objectives, based on the AI model that defines credit-worthiness. It does so by using both machine-based inputs such as historical data on people’s profiles and on whether they repaid loans, and human-based inputs, such as a set of rules. With these two sets of inputs, the system perceives real environments: whether or not people regularly repay their loans. It then automatically abstracts these perceptions into models.
It is easy to imagine that a credit-scoring algorithm could use a statistical model to recommend a credit score and determine if a loan application is approved or denied.
How the AI system dimensions are structured
The classification framework aims to be simple and user-friendly rather than exhaustive and complex. The OECD AI Principles help structure the analysis of policy considerations associated with each dimension and sub-dimension. This makes it applicable to the most relevant cases.
Each dimension has distinct properties and attributes, i.e. sub-dimensions that raise specific policy considerations. For example, ‘Data and Input’ properties include data and input collection, structure, domains, quality and qualification.
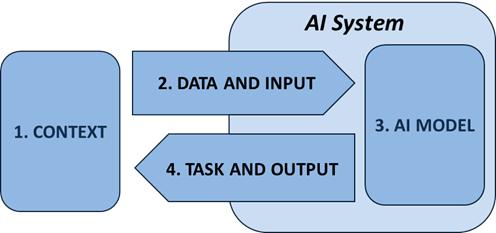
All four dimensions clarify zones of accountability and attribute them to the actors involved in each phase: The Context implicates system users; the Data and Input dimension concerns the actors who collect and process data and inputs; those involved in the AI model dimension are mostly system developers; Tasks and Output actors include anyone involved in the chain of system operators and those who exploit the outputs.
Review the classification framework and follow the next steps
The Network of Experts plans to test the robustness and applicability of the present framework in late 2020 / early 2021, possibly leading to adjustments. The Network will also seek wider feedback on the interim report from more AI expert groups, policy communities at the OECD and elsewhere, and individual policy makers.
The OECD’s work on developing a classification framework for AI systems is spearheaded by The OECD’s Network of Experts on AI, and its Working Group on the Classification of AI.