Towards algorithm auditing: A research overview
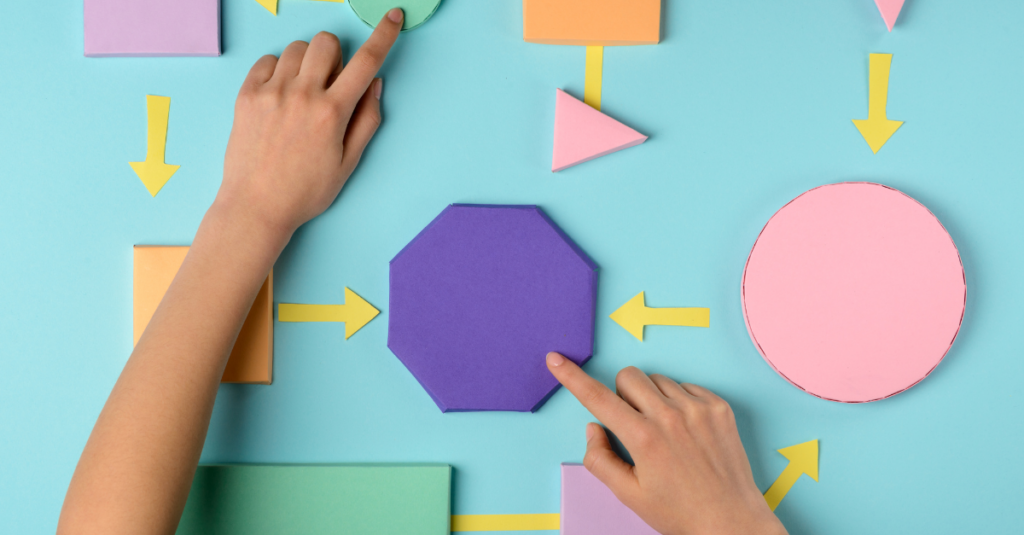
We are witnessing an exponential increase in the adoption of AI across businesses, governments, and society. Very quickly, technology has become a competitive necessity to streamline operations, improve user experience, and reduce the human burden of tedious tasks. Yet, while the power of AI has great transformational potential, the application of the technology is not without risk of significant financial or reputational damage if the algorithms underlying these complex models fail or result in undesirable or unethical outputs.
Indeed, recent years have seen risks turn into harm in several high-profile cases across sectors, including bias in automated recruitment tools, insurance and credit scoring, emissions scandals, and companies even going bust due to their algorithms.
As such, the benefits of AI must be harnessed while risks and harms are controlled. Accordingly, social movements and public bodies call for safety and control mechanisms. In response, governments worldwide are legislating AI and imposing bans on unacceptably risky applications. Regulators are imposing considerable fines on businesses using AI in ways that do not comply with current laws.
Algorithm auditing: A comprehensive framework
To support these efforts, our latest paper, ‘Towards algorithm auditing: managing legal, ethical and technological risks of AI, ML and associated algorithms’, published in the Royal Society’s Open Science Journal, lays the groundwork for algorithm audits. Included are the research and practice of assessing, monitoring and assuring an algorithm’s safety, legality and ethics by embedding appropriate socio-technical interventions to manage and monitor risks. Such audits serve as a mechanism for formal assurance that algorithms are legal, ethical and safe by providing a robust and independent evaluation of an AI system’s reliability, safety vulnerabilities, and risk management capabilities.
Like financial audits, algorithm audits are becoming the norm in AI. However, this success heavily depends on how effectively it is systematised, scaled, and standardised. As such, in our paper, we leverage multidisciplinary expertise and practical, real-world insights to present an iterative framework for algorithm auditing with four key dimensions, as seen in the figure below.
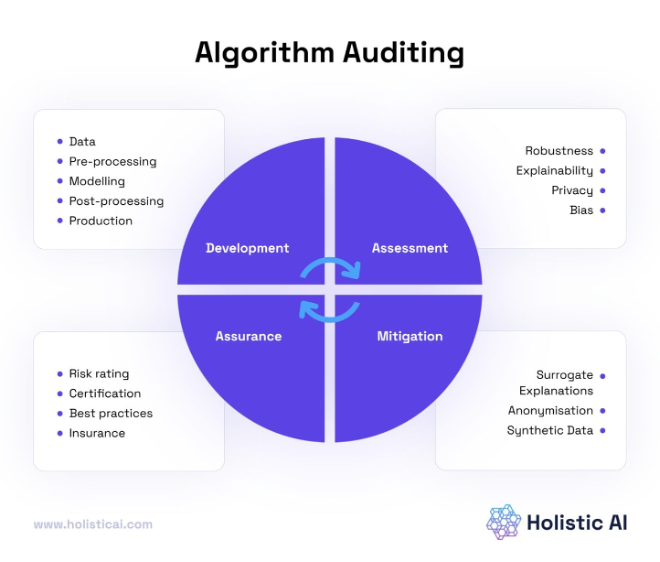
Phases of algorithm auditing
In the development phase of the audit, all elements of an AI system are documented, including information on data inputs and outputs, any pre- or post-processing of data, details about model selection and factors influencing decisions etc. This documentation supports the assessment phase, which assesses AI systems according to five key risk verticals:
- Bias – the risk that the system treats individuals or groups unfairly
- Efficacy – the risk that a system underperforms relative to its intended use case, particularly on unseen data
- Robustness – the risk that a system fails in response to changes in datasets or adversarial attacks by malicious actors
- Privacy – the risk that a system is sensitive to personal or critical data leakage or facilitates the reverse engineering of such data
- Explainability – the risk that an AI system is not understandable to users and developers caused by not effectively communicating important information
Each risk is quantified by state-of-the-art and context-specific metrics for an impartial system evaluation.
Where risks are identified, mitigation actions to reduce the risk of harm include developing better documentation or communication mechanisms, surrogate explanations, retaining models with more representative data, and anonymising private data. This can lead to an iterative process where systems are re-evaluated to determine if the risk has been reduced and the mitigations were successful.
On the other hand, if no mitigations are required, recommendations could include reviewing processes for their “up-to-datedness”.
Either way, documentation should be updated to reflect any changes made to systems or associated procedures to support the next phase’s development and contribute to overall AI governance. Indeed, audits should not be considered a one-time activity – AI systems should be monitored continuously, and audits should be conducted periodically, particularly after a system has been updated or redeveloped.
Algorithm assurance
The audit process’s outcome is assurance – declaring that a system conforms to predetermined standards, practices or regulations. To do so requires governance, impact and technical assessments to ensure clear lines of accountability and liability. To navigate the differences between countries and economic blocks, the relevant contextualised and jurisdictional legal landscape must also be mapped out. These and technical assessments of the five key verticals outlined above feed into documentation and an audit trail, which, in turn, facilitates the certification of systems.
We also envision an emerging market for algorithm insurance, which could become a significant governance requirement for companies using AI systems, particularly those in critical sectors such as healthcare, education, recruitment, financial services, and housing. This will align closely with explainability and algorithm auditing in accordance with regulations and standards, where insurance premium pricing will need an understanding of the risks involved in each vertical of the algorithm system. In line with requirements under the EU AI Liability Directive, which provides protections and routes for recourse for users harmed by AI, we also predict a requirement for indemnity insurance for high-risk sectors or end applications.
Laws requiring algorithm auditing
It is important to note that assurance coverage can only be provided against the frameworks, regulations, or laws a system was evaluated against during the audit; assurance cannot be taken as an indication that an algorithm completely complies with all relevant laws. Instead, an audit should focus on a specific (legal) framework to ensure that it follows the required methodology and uses appropriate metrics.
For example, two laws have made algorithm auditing a legal requirement – New York City Local Law 144 and the EU Digital Services Act (DSA). Local Law 144, governing automated employment decision tools, narrows the audit’s focus to bias, while the DSA is more of a process audit of online platforms that aims to protect the users’ fundamental rights of these services by ensuring that transparency is maximised. At the same time, disinformation and illegal content are removed from platforms through robust mechanisms.
Using a generalised approach to auditing, it is unlikely that either legal framework’s requirements would be fully met, highlighting the need for framework-specific audits and subsequent assurance. As such, the paper outlines a flexible but comprehensive framework that can be adapted to different contexts and frameworks to maximise compliance and trust in AI while minimising risk.
For policy stakeholders to develop algorithm auditing
Our goal with this paper is to initiate conversations among stakeholders in policy, regulation, and industry and to contribute to the constructive development and maturation of algorithm auditing. As such, our target audience spans a range of players in the AI governance space, including policymakers, regulators, government agencies, practitioners, developers, lawyers, policy professionals, and academic researchers.
Overall, we contend that algorithm auditing will become integral to AI governance and experience exponential growth in the forthcoming decade. This trajectory aligns seamlessly with the increasing emphasis on the comprehensive management of risks associated with AI applications, as demonstrated by a sharp increase in AI legislation over the past few years. This will be vital for ensuring that AI’s power can be harnessed safely and fairly to facilitate innovation and consequential societal benefits.